1 Introduction
On July 29, 2016, U.S. President Barack Obama signed a bill directing the Secretary of Agriculture to establish a national, mandatory bioengineered food disclosure standard, mandating the labeling of foods containing genetically modified ingredients. The enactment of the bill marked the latest step in an often contentious debate involving agribusiness, interest groups, scientists, legislators, and the public since the first genetically modified food products were approved by the Food and Drug Administration in the early 1990s [Lang and Hallman, 2005 ].
The Bioengineered Food Disclosure Standard requires that manufacturers disclose whether their food products contain any “bioengineered”, or genetically modified, ingredients. What qualifies as “genetically modified” and what term ought to be used to describe it (e.g., GMO, genetically engineered, bioengineered, etc.) is an issue that the United States Department of Agriculture (USDA) is currently working to resolve [Hallman, 2018 ]. As described by Tagliabue [ 2016 ], the colloquial term “GMO” is imprecise and could refer to a number of items (food products, crops, and others) created through a number of processes (e.g., transgenesis, gene editing). There is no “common denominator” for the term [Tagliabue, 2016 ]. Instead, the term GMO is a cultural construct that is tied up with issues of safety, human rights, environmental protection, purity, fair trade, naturalness, and morality [Johnson, 2015 ; Scott, Inbar and Rozin, 2016 ].
Meanwhile, despite the fact that people know little to nothing about bioengineered foods and cannot articulate what “GMO” means [Hallman, Cuite and Morin, 2013 ; Hallman, 2018 ], the term “non-GMO” is being used to market products to consumers, and these non-GMO products are one of the fastest growing segments of the food market [Bain and Selfa, 2017 ]. In fact, many companies, such as Whole Foods, 1 Ben and Jerry’s, 2 and Chipotle 3 are all jumping on the values-based marketing bandwagon not only to label foods as non-GMO or GMO-free, but to completely eliminate “GMOs” from their product lines. Companies that have not eliminated “GMOs” from their products are increasingly implementing efforts to boost transparency about the use of GM ingredients (in part to fulfill the Federal disclosure standard), resulting in statements on the SmartLabel.org website, under a tab specifically marked “GMO disclosure” (see for example: http://smartlabel.generalmills.com/16000427310 ). The disclosure in the example states the following:
This product includes ingredients sourced from genetically engineered (GE) crops, commonly known as GMOs. Farmers use GE seed to grow the vast majority of corn, soy, canola, and sugar beets in the U.S., and those ingredients, or ingredients made from these crops, are in 70 percent of foods on U.S. grocery store shelves.
Despite its scientific ambiguity and because of its social relevance, we use the terms GMO, GM foods, and GM ingredients in the current study as “catch all” terms for the products of modern agricultural biotechnology that are under scrutiny amongst some segments of the public for the perceived conflict (whether real or imagined) between the processes used to create these products and public values. After all, this study is not one about GM technology or products specifically, but one about how they and the companies that produce them are perceived in the minds of the public. Specifically, in a novel experiment, we examine whether disclosing to the public that the organizations engage in open and transparent research practices will increase public perception of those organizations trustworthiness.
1.1 Public input and responsible innovation
Incorporating public values into the process of new technological development and policy-making is reflective of steps toward responsible innovation [see e.g., Taebi et al., 2014 ; also Guston et al., 2014 ]. Though the underlying concepts of responsible innovation (e.g., anticipation of social, ethical and legal implications; moving beyond just engaging expert stakeholders to include the wider public; [Stilgoe, Owen and Macnaghten, 2013 ] ) seem worthy of automatic acceptance to many [Guston, 2015 ], there is ongoing discussion among scholars and policy makers about whose opinion about a given technology matters and when [Taebi et al., 2014 ].
The implementation of responsible innovation is often complicated by values divides among stakeholder groups, such as researchers and the public or even divisions among different researchers and different publics. These differences can lead to a societal-level rejection of applications that researchers might find beneficial. For instance, societal-level debate continues over embryonic stem cell research. Whereas scientists value the potential of stem cell research to help cure disease, certain publics reject this research due to the perceived moral implications of destroying human embryos.
In some cases, however, differences in stakeholder sentiment is not reflective of inherent values differences but of unwarranted fears and misconceptions. Before knowledge can accrue about new technologies’ genuine risks and benefits, public sentiment toward those new and unfamiliar technologies can be swayed by emotionally-charged campaigns (e.g., “Frankenfood”; [Welchman, 2007 ] ). As such, one challenge for industries that wish to engage in responsible innovation is determining how to overcome the barriers and biases that prevent individuals from forming beliefs based on the best available evidence. Doing so can involve pinpointing and addressing the source(s) of misinformation and the unfounded fears.
In this research, we examine one potential source of unfounded public fears about GMOs. Scientists generally agree that the GMOs that are currently on the market are as safe for human consumption as more conventionally-developed crops and that any regulation should focus on the products and not on the process used to create those products [NASEM, 2016b ]. One reason the public may be skeptical about the safety of these products, at least in part, is because much of the data comes from the organizations that stand to profit from these products. This conflict of interest may reduce the public’s trust in the credibility of any results that are supportive of the technology.
1.2 Trustworthiness
When people are unable to judge the quality of information about highly specific scientific domains, as is the case with various emerging technologies [Hardwig, 1985 ; Hendriks, Kienhues and Bromme, 2016 ; Siegrist, 2000 ], they tend to rely, instead, on their assessments of the originator’s trustworthiness; or the extent to which they perceive relevant stakeholders as having topical knowledge (e.g., expertise) and positive motivations, such as putting public welfare before their own interests [e.g., Fiske, Cuddy and Glick, 2007 ; Hovland, Janis and Kelley, 1953 ; Landrum, Eaves and Shafto, 2015 ]. There are a variety of stakeholders for whom consumers’ perceptions of trustworthiness affect views of the research, development, production, and sale of GMOs. These include specific individuals, specific organizations, and the broader context in which transactions are taking place [Grayson, Johnson and Chen, 2008 ].
For instance, consumers may mistrust the government regulatory processes implemented to determine the safety of any GM products [Gutteling et al., 2006 ]. A recent survey found that about half of individuals surveyed expressed skepticism about federal regulatory agencies’ (e.g., USDA, FDA) abilities “to provide impartial and accurate findings on the safety of genetically engineered or modified crops”; only 42% of survey respondents reported “a great deal” or “a fair amount” of trust, whereas 55% reported “just some” or “very little” trust [APPC & Pew Research Center, 2015 ]. 4
1.3 Conflicts of interest
In addition to skepticism toward regulators, consumers are often skeptical toward individuals and organizations that have a financial stake in the success of research outcomes. For instance, when participants were asked about their overall impression of Monsanto, an agriculture corporation known for making GM products, 43.1% offered an unfavorable opinion compared with only 20.6% reporting a favorable one 5 [APPC & Pew Research Center, 2015 ]. In comparison, when asked their opinion about the USDA, 30.4% of participants reported having an unfavorable opinion and over 70% of participants expressed a favorable one.
Research has demonstrated that people are less trusting of research from for -profit corporations than from non -profit ones such as universities [eg., Critchley, 2008 ; Critchley and Nicol, 2011 ; Lang and Hallman, 2005 ], presumably because of the potential for conflicts of interest. Thus, it is unsurprising that when corporate funding is involved, even university research can lose credibility. These concerns can be intensified by media coverage of such cases.
In September of 2016, for instance, a review article published in the internal medicine division of the Journal of the American Medical Association revealed the previously undisclosed fact that the sugar industry had funded decades-old Harvard research that downplayed sugar’s contributing role to coronary heart disease and instead stressed the role of fats [Kearns, Schmidt and Glantz, 2016 ]. This revelation received extensive media attention [Bailey, 2016 ; Domonoske, 2016 ; O’Connor, 2016 ; Shanker, 2016 ; Sifferlin, 2016 ], with articles calling the situation a “scandal” and highlighting other instances of questionable industry-funded research [Rodman, 2016 ; Schumaker, 2016 ]. A statement from the Sugar Association in response to the findings said that the sugar industry “should have exercised greater transparency in all of its research activities” [O’Connor, 2016 ].
Such exposures, of course, fuel public skepticism about corporate-funded research. This is particularly evident in reports about the safety of GMOs for human consumption. A panel of scientists convened by the National Academies of Sciences, Engineering, and Medicine, for example, stated in a recent consensus report that the GM products currently on the market are as safe for human consumption as their non-GMO counterparts [NASEM, 2016b ]. In response, anti-GMO activists and others questioned the credibility of the panel, stating that some on it had unreported financial conflicts of interest, such as having received research funding from corporations and holding a patent on GM technology [e.g., Krimsky and Schwab, 2017 ].
In many situations, it is impossible for financial conflicts of interest to be completely absent. After all, the groups most willing and able to fund such work are often those who desire the products of such research to succeed. With this in mind, the National Academies rigorously screens potential panelists, excluding top experts who have conflicts of interest whenever possible. When it is not possible, the National Academies reports existing conflicts of interest that meet specific criteria (e.g., when equity holdings are greater than $10,000). An official from the National Academies reported that of the almost 5,500 experts they used as panelists in 2016, only 14 had what the Academies considered a relevant conflict [Basken, 2017 ] ]. Furthermore, to counteract any potential effects of conflicts of interest on the final reports, the reports undergo a thorough, iterative peer-review process. Kathleen Hall Jamieson, a reviewer on the NASEM report (and an author on this paper) said “they review things all but to death” [Basken, 2017 ].
1.4 Coping with conflicts of interest
In agricultural biotechnology, as in the pharmaceutical and nanotechnology research sectors, there is a clear and necessary role for private corporations — namely, investment — particularly as federal funding wanes. Such funding hastens knowledge production and accelerates product development [Chalmers and Nicol, 2004 ; Critchley, 2008 ]. However, by their nature, these investments also create conflicts of interests, and with them the worry that such conflicts cause researchers to overstate the benefits and understate the risks of any particular product or technology. Accordingly, when university researchers engage in collaborative efforts with industry partners, or when corporations hire their own researchers to test the safety of products, questions are raised about the reliability and full disclosure of all results [DeAngelis, 2000 ; Myhr and Traavik, 2003 ; Diels et al., 2011 , also see].
More research ought to focus on determining the best ways to limit the influence of conflicts of interest on research outcomes themselves, beyond simply reporting the fact that such conflicts exist. Although many research institutions have implemented conflict of interest reporting policies to reveal when research is industry-funded [Gurney and Sass, 2001 ], such measures are not sufficient to reassure the public that there are measures in place to help thwart bias. In other words, although reporting such conflicts of interest demonstrates transparency, it likely does little to increase consumer confidence in the trustworthiness of the findings. Instead, highlighting conflicts of interest likely provides consumers with more reason to question research findings, thus reinforcing antagonistic attitudes.
Incorporating a program to help mitigate potential effects of conflict of interest is comparable to implementing safe-by-design (or prevention through design) practices. In the responsible innovation literature, “safe by design” highlights the need to limit (and the benefit of limiting) any potential hazards during the design process [e.g., Baram, 2007 ]. By building methods of combatting negative effects of research bias into the process of evaluating new products and technological methods, we can limit negative effects of human error [e.g., Wilpert, 2007 ].
Implementing research standards that hold researchers accountable for the full disclosure of results, is one potential method of combatting human bias that can be built into the system of designing GM products. Moreover, disclosing such research standards to the public may help to increase public perceptions of the organizations’ trustworthiness. One set of standards is telegraphed by the terms “open and transparent research practices” [Alberts et al., 2015 ; Nosek et al., 2015 ]. These practices have recently been gaining traction as a way to thwart similar biases in academic research [Nosek et al., 2015 ].
1.5 Open and transparent research standards
In research, a number of processes may lead to overestimation of the strength and robustness of research findings. For one, studies that find a “significant” effect are more likely to be submitted and accepted for publication than are those that do not find such an effect (i.e., publication bias). Moreover, researchers may engage in selective analysis, changing their hypotheses after the fact to find support (i.e., “hypothesizing after the results are known”; [Kerr, 1998 ] ) or attempting many analyses but report only the ones finding the desired result (“p-hacking”; [Simmons, Nelson and Simonsohn, 2011 ] ). In addition, mistakes may occur in data entry, cleaning, or analysis, and when raw data remain the exclusive property of the original laboratory, these mistakes may not be detected or corrected.
To address these problems, a number of open and transparent research standards have been developed. To prevent publication bias, some journals have adopted the “registered report” format for academic articles. In a registered report, peer reviewers evaluate and accept an article on the basis of its methods; once accepted, the research is performed and published regardless of the statistical significance of its results. Similarly, to prevent selective analysis, researchers can “preregister” a set of intended primary analyses before conducting a study or experiment. This prevents researchers from changing the hypotheses, analyses, or outcomes to try to find support for a preferred conclusion. Finally, the open sharing of data facilitates scrutiny across laboratories, enabling additional layers of error detection and correction [see: COS, 2016 ].
Free online tools for preregistration and data-sharing are provided by the Center for Open Science, a non-profit organization. In addition to building and maintaining such tools as the Open Science Framework [Spies, 2013 ], the Center for Open Science organizes collaborative projects between researchers in order to conduct research in a transparent way that minimizes opportunities for biases caused by self-interest.
In theory, the same principles could be applied to the corporate world, particularly when reporting the results of safety testing. If corporations engaged in some variant of open and transparent research practices when conducting and reporting the results of safety tests, then checks and balances would be in place to ensure that research does not suffer from the negative effects that can result from conflicts of interest. Indeed, the presence of such checks and balances seems to have strong effects on reported research. For instance, in the year 2000, the U.S. National Institutes of Health required that all large randomized clinical trials preregister their outcomes and analyses. Since that time, such trials are more likely to report null or negative, rather than positive results. Prior to these regulations, 17 out of 30 randomized clinical trials reported significant benefits; after these regulations, only 2 out of 25 reported significant benefits [Kaplan and Irvin, 2015 ]. Thus, open and transparent research practices appear to reduce the influence of conflicts of interest.
1.6 Experiment aims
Ensuring that the research on the safety of GMOs is actually trustworthy (i.e., is conducted responsibly and is replicable and reproducible) is important. However, when study results are trustworthy, how do we reassure the general public that is indeed the case?
Related work by Dixon and colleagues has shown that transparency in describing how an organization came to a decision and highlighting that public input was elicited can increase public support for policy decisions regarding GM technology [Dixon et al., 2016 ]. We, in contrast, examine the influence of another type of transparency on public confidence; that is, does describing the fact that an organization engages in open and transparent research practices increase public trust? As stated earlier, there has been little work on how to address the lack of trust in research funded by corporations that stems from conflicts of interest (beyond simply reporting it). Similarly, it is not known whether making research practices more transparent and communicating said transparency might promote trust among non-expert publics. Our experiment is novel in that it addresses these open questions.
Specifically, this experiment examines the effects of organization type (university versus corporation) and the disclosure of open and transparent research practices (versus “business as usual” practices) on public perceptions of trustworthiness of the organizations and researchers examining and developing GMOs. Prior survey work has shown that the public has greater confidence in universities than in corporations when issues involving researching and regulation of GMOs are at play [e.g., Lang and Hallman, 2005 ], and experimental work has shown that participants trust universities more than industry (and industry-funded organizations, [Critchley, 2008 ; Critchley and Nicol, 2011 ] ). Therefore, we predict that
- H1.
- Universities will be perceived as more trustworthy than corporations when it comes to researching and developing GMOs.
Moreover, we hypothesize that the members of the public will recognize that open and transparent research practices can hold organizations more accountable for reporting findings objectively, leading to our prediction that
- H2.
- Organizations described as engaging in open and transparent research practices will be perceived as more trustworthy than organizations not described in this way.
Importantly, other heuristics are also likely to influence trustworthiness evaluations of these organizations and their researchers. For example, research that supports one’s views is often seen as more trustworthy than that which challenges them (e.g., motivated reasoning, confirmation bias, myside bias; [Kunda 1990 ; Baron, 2000 ; Koehler, 1993 ; MacCoun, 1998 ] ). Accordingly, members of the public who hold strong anti-GMO attitudes are not likely to trust organizations using that technology, regardless of the type of sponsoring organization or whether that organization engages in open and transparent research practices. Thus, we predict that:
- H3.
- Negative attitudes towards GMOs will be negatively associated with perceptions of trustworthiness.
To examine these hypotheses, we conducted an online experiment. Prior to data collection, we preregistered the experiment design and our hypotheses with the Center for Open Science using their Open Science Framework (see https://osf.io/6pwrz/ ).
2 Method
2.1 Sample
Participants for this experiment were part of a consumer panel recruited by Research Now. See appendix A for information on the recruitment, compensation, and exclusion of panel participants. The final sample consisted of 1097 participants.
This sample was demographically and ideologically diverse and comparable to many nationally-representative surveys in terms of age and gender. Participants ranged from 18 to 88 years old ( median = 48 years, mean = 47.39). About 48.9% identified as male, 51.0% identified as female, and two participants declined to provide gender. Sixty-five percent (65.6%) identified as white, non-Hispanic, 14.2% identify as black or African-American, 8.7% identify as Latino/Hispanic, and 7.8% identify as Asian. Regarding political affiliation, 47.4% reported being Democrats or leaning toward supporting the Democratic Party, 35.5% said they were Republicans or leaned toward supporting the Republican Party, and 13.3% identified as strictly independent. Regarding ideology, 35.3% saw themselves as very or somewhat liberal, 34.0% identified as moderate, and 30.7% identified as very or somewhat conservative. Importantly, these demographic variables did not vary significantly across the experimental conditions. See appendix B .
2.2 Experiment Design
Participants were randomly assigned to one of six conditions. In each, subjects read a press release 6 from an organization describing its research activities. Organization and research practices varied by condition. In the control condition, subjects read a press release about a baseball research society’s upcoming conference. In the real corporation condition, participants read about a real corporation engaging in business-as-usual research practices. This organization was Monsanto, a recognizable agricultural corporation known for researching and developing GMOs. 7 8 The four remaining conditions were designed as a 2 (Organization: university agricultural department vs. novel corporation) 2 (Research practices: baseline vs. open and transparent) experimental design.
The press releases used in the four main experimental conditions were based on the material from the original press release used in the real corporation condition. There were two manipulations in each press release: the organization type and its research practices. For all of the conditions except the baseball-control condition, the press release announced that an organization developed a new type of soybean that benefits the consumer by offering an improved nutritional profile.
In the two novel corporation conditions, the press release was designed to look as if it came from an agricultural corporation called “Virens”, a company that we fabricated for the purpose of this study. In the two university agricultural department conditions, the press release was designed to look as if it came from the University of Pennsylvania College of Agriculture and Life Sciences, a department which does not exist.
The press release also included a “research practices” section. In the baseline conditions (including the real corporation condition; i.e., condition 5), the research practices section had text from the real corporation’s website:
[Organization Name] pledges to take responsibility in achieving results. This pledge includes:
- Building strong relationships with our external partners;
- Making wise decisions; and
- Taking responsibility for achieving agreed-upon results.
“Our research and development team is dedicated to developing seeds that make growing vegetables easier for farmers, while also meeting the needs of everyone in the produce chain –including retailers, food service, and consumers.”
In the two open and transparent conditions, the research practices section included text we drafted based on information from the Center for Open Science on open and transparent research practices.
[Organization Name] now partners with the Center for Open Science (COS), a non-profit dedicated to fostering the integrity and reproducibility of scientific research. As a part of this partnership, [organization] has adopted COS research guidelines. We now:
- Pre-register our research studies (reporting in advance what we plan to do);
- Make our data available to the public; and
- Explain all of our methods for analyzing data.
“These guidelines are aimed at making sure our research upholds the values of scientific integrity. We pledge to make information available, accessible, and understandable.”
The sixth condition (baseball control), did not have any information about research practices. Instead, it discussed an upcoming convention, including a list of the scheduled speakers, the dates the convention was to be held, and the location of the convention. The control condition made it possible to determine whether the GMO knowledge and attitudes measures (which occurred after the manipulation) were influenced by the manipulations (the press releases) that discussed GMOs. See appendix C for comparisons of GMO items across conditions. Appendix D contains press releases used in the experiment.
2.3 Dependent variables
Our study aimed to examine the influence of our manipulation on how trustworthy participants found the organization and its researchers to be. We want to highlight that we are not measuring consumers demonstrations of trust (e.g., behaviors such as the purchasing of products), but their perceptions of the organization and its researchers’ trustworthiness . We operationalized trustworthiness in four ways: explicit ratings of organization credibility (e.g., “how credible do you find…”), ratings of researcher credibility (with subscales of trusting researchers to have positive intentions, i.e., “benevolent”, trusting researchers to have expertise, i.e., “competent”, and not trusting researchers, or being skeptical of them), rankings of perceived researcher knowledge, and rankings of perceived researcher ethics. These ways of operationalizing trustworthiness are based on prior research by Critchley [ 2008 ] and theories of epistemic trust, credibility, and dimensions of social cognition [Fiske, Cuddy and Glick, 2007 ; Landrum, Eaves and Shafto, 2015 ; Pornpitakpan, 2004 ]. We describe these variables in more detail below.
Organization credibility. Our first measure of trustworthiness, organization credibility, was created by combining two ratings of organization credibility on scales from 0 to 100 into an averaged index. The first item asked how credible the research is that comes from the organization ( , ) and the second asked how credible the organization itself is ( , ). The two items were highly correlated ( , ) and showed similar relationships to education, familiarity with GMOs, and perception of GMO safety.
Researcher credibility. Our second measure of trustworthiness was created using a researcher credibility scale that we adapted from Critchley [ 2008 ]. Each item was rated on a scale from 1 to 6, where 6 indicates higher agreement. See appendix D . A factor analysis revealed three primary factors consistent with Critchley [ 2008 ]: one reflected participants’ trust that researchers are competent (e.g., researchers are very knowledgeable about their areas of expertise), one reflected participants trust that researchers are benevolent (e.g., researchers consider how their research influences the health and well-being of the American public), and the third reflected participants’ skepticism towards researchers (e.g., researchers are primarily motivated by financial interests). We used the items that loaded onto each factor to create an averaged index of each factor: trustworthiness: competence ( , ); trustworthiness: benevolence ( , ); and Skepticism: not trustworthy ( , ). For the two trust scales, higher scores indicated more perceived trustworthiness, and for the skeptical scale, higher scores indicate less perceived trustworthiness (more skeptical).
Researcher rankings. Our third and fourth measures of trustworthiness were obtained by asking subjects to rank a set of 7 organizations (one of which was the test organization) on how knowledgeable the researchers are when it comes to research about GMOs (knowledge ranking) and how ethical the researchers are when it comes to research about GMOs (ethics ranking). The other organizations listed were scientific organizations (e.g., the National Academies of Science), non-profit organizations, university agricultural departments and research centers, anti-GMO advocacy groups (e.g., Non-GMO project, Organic Consumers Association), regulating agencies (e.g., the Food and Drug Administration), and agricultural corporations (e.g., DuPont, Syngenta). Note that the lower the number, the higher the ranking, such that 1 = top-ranked and 7 = bottom-ranked. See Table 2 for the mean and median rankings collapsed across condition. However, for our regression analysis, we reverse coded the rankings into scores (e.g., converted a ranking of 1 to a score of 7 and, conversely, a ranking of 7 to a score of 1) so that the direction of the relationships would be more intuitive — higher scores indicate greater perceived trustworthiness. These two rankings — knowledge and ethics — served as our dependent variables for further analyses.
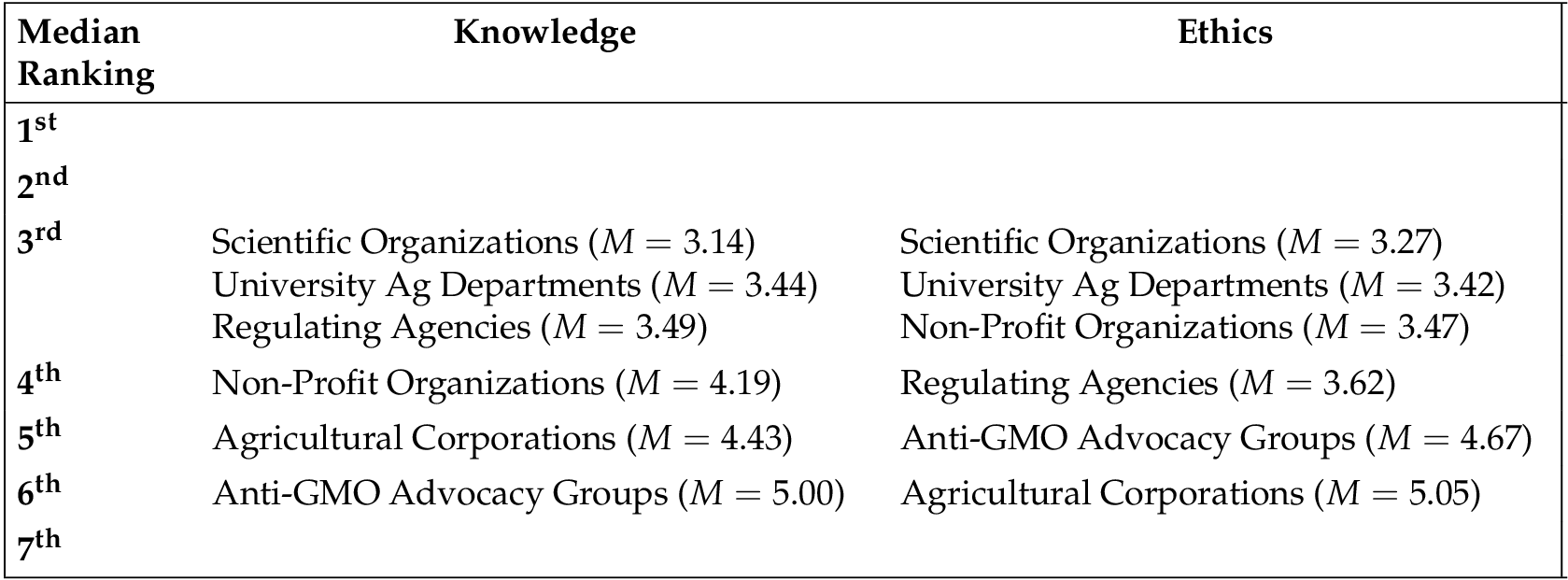
Our third hypothesis involved examining how attitudes toward GMOs influenced trust. Thus, we included a series of items aimed to measure attitudes towards GMOs, participant policy positions regarding GMOs, and knowledge about GMOs. See appendix C . For the attitude items, we asked participants whether they perceived GMOs to be safe where 4 was “GMOs are as safe as conventional crops” and 0 was “GMOs are NOT as safe as conventional crops” ( , , ). We also asked participants a series of risk and benefit items. These items were combined into an averaged index of GMO risk perceptions ( , , ) and an averaged index of GMO benefit perceptions ( , , ), both of which were on a scale from 0.25 to 2.25. We also asked participants whether they purposefully avoid eating GMOs (34% agreed).
Regarding participants’ GMO policy positions, we asked participants whether GMO technology should be (a) banned in all circumstances, (b) banned for making food items, but allowed for making non-food items, or (c) should not be banned. We recoded this into two variables: one representing whether the participant believes in banning all GM technology (10.8% of the sample) and one representing whether the participant believes in banning GM food only (40.8% of the sample). We also asked participants whether GMOs should be labeled (about 90% agreed) and whether GM technology should be regulated (85% agreed).
Regarding participants’ knowledge about GMOs, we asked how much they would say that they have heard or read about GMOs on a scale from 1 (not very much at all) to 5 (a great deal; , ). We also asked participants which best describes the process used to create GMOs. About 10% thought the description of mutagenesis best described genetic-modification process, about 45% thought that gene-editing best described the genetic-modification process, and the remaining either chose cross-pollination (16%) or said that they did not know (27.4%). We dummy coded the variable so that we had a variable for those who thought it was the description of mutagenesis and one for those who chose the description of gene-editing.
Importantly, although we asked these items after the experimental manipulation (so we would not influence participants’ responses to the trust items by priming them to think about their views toward GMOs), we did not expect that knowledge about and attitudes toward GMOs would vary based on our experimental manipulation. To test this, we compared each of the knowledge and attitudes items across the six conditions, which included the baseball control condition. No condition varied significantly from the baseball condition, for which participants were not primed to think about GMOs prior to answering the knowledge and attitudes questions. These analyses are reported in appendix C with the description of each of the items.
3 Results
We used hierarchical ordinary least squares (OLS) regression models to separately test the effect of our experimental manipulation as well as the influence of attitudes, policy positions, and beliefs about GMOs on each of the dependent variables. 9 In hierarchical OLS, the independent variables are entered in blocks in order to examine their relative explanatory power. We entered our independent variables in the following blocks:
- Block 1.
- Organization type (university = 1; corporation = 0);
- Block 2.
- Research practices (open & transparent = 1; baseline = 0);
- Block 3.
- Attitudes towards GMOs (views about the safety, risks, benefits, and avoidance of GMOs);
- Block 4.
- GMO policy positions (banning GM technology, banning GM food, labeling GMOs, regulating GMOs);
- Block 5.
- Knowledge about GMOs (familiarity, process: gene editing, process: mutagenesis, and identification of consensus); and
- Block 6.
- Interaction effect between organization type and research practices.
See Table 2 for the results from the regression analyses.
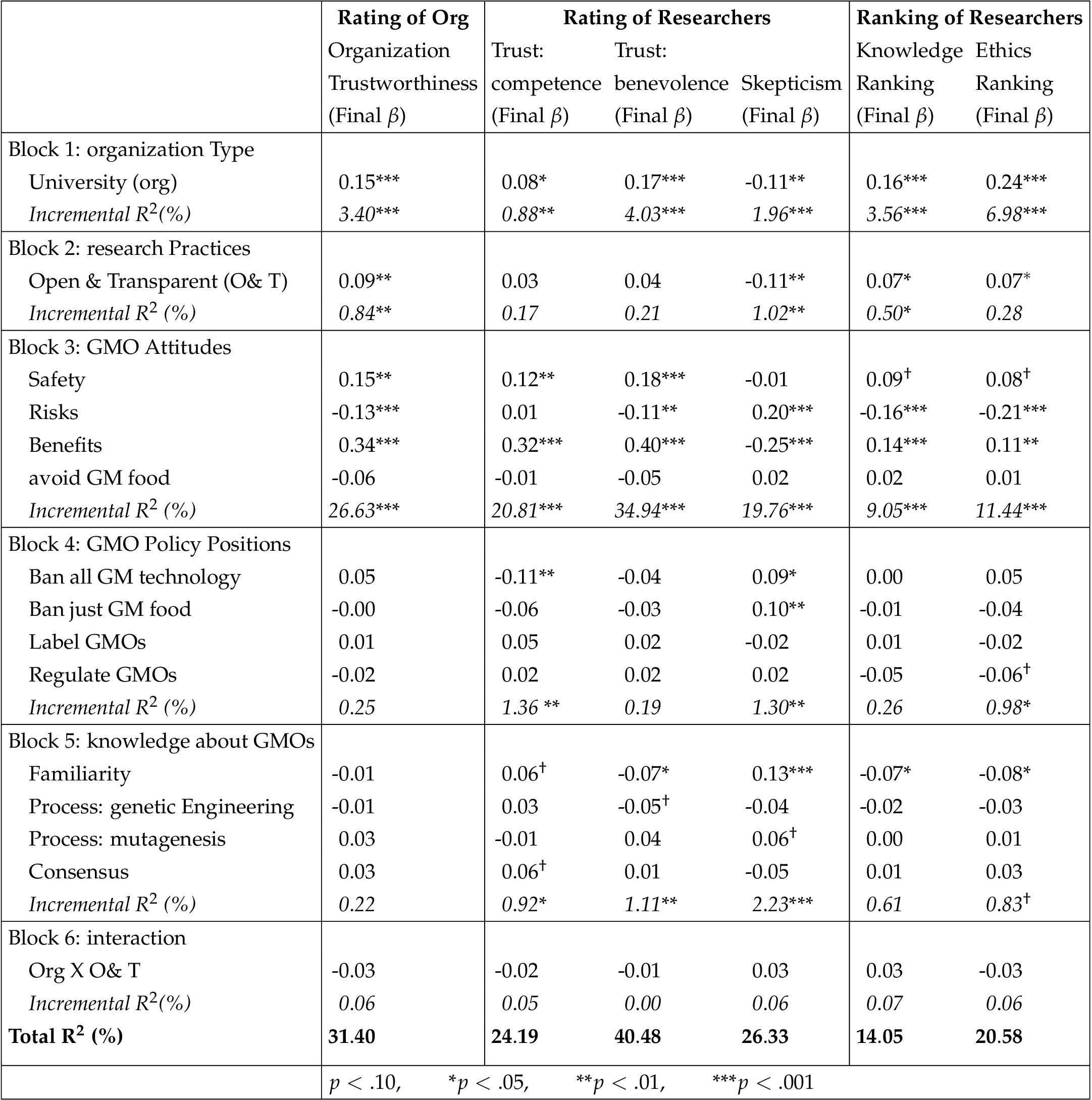
Effects of experimental manipulations. As hypothesized (H1), we found a significant effect of organization type for each of the dependent variables, such that participants who read about the university agricultural department found the organization to be more trustworthy than participants who read about a corporation. We also found partial support for our second hypothesis (H2). Overall rating of organization trustworthiness, rating of skepticism towards researchers, and the relative rankings of knowledge and ethics varied such that organizations that were described as engaging in more open and transparent research practices were seen as more trustworthy. However, the use of such research practices had no significant effect on ratings of researchers’ competence and benevolence. 10
Effects of GMO attitudes. Attitudes toward GMOs also influenced perceptions of trustworthiness of the organizations researching and developing GMOs (H3). Perceptions of risks and benefits influenced perceptions of trustworthiness in the anticipated direction: greater perceived risk predicted lower perceptions of trustworthiness and greater perceived benefits predicted greater perceived trustworthiness. 11 Beliefs about the safety of GMOs, on the other hand, only partially predicted trustworthiness: perception of GMO safety was positively associated with ratings of organization trustworthiness and ratings of competence and benevolence, was marginally positively associated with the ranking of researchers along knowledge and ethics, and was not significantly related to skepticism toward researchers.
Effects of GMO policy positions and knowledge. Results regarding GMO policy positions were mixed. Participants who would like to ban all GM technology or ban just GM foods were more critical (i.e., higher skepticism scores) of researchers developing GMOs. Policy positions on labeling and regulating GMOs, however, were not related to trustworthiness, potentially because there was not much variance in these positions. Similarly, knowledge about GMOs was also mostly unrelated to perceptions of trustworthiness. The exception is self-assessed familiarity: people who reported more familiarity with GMOs were also more skeptical of the researchers working on GMOs, less likely to rate those researchers as benevolent, and more likely to give the organization that they read about lower rankings (higher numbers such as 6 and 7 versus lower numbers like 1 and 2 ) in both knowledge and ethics.
3.1 Exploratory analyses
Although we found some support for the hypothesis that disclosing open and transparent research practices would increase trustworthiness, these findings were not very robust nor did they hold across all dependent variables. One reason that the effects of disclosing open and transparent research practices were not stronger may be a lack of public understanding of the benefits of open and transparent research practices. Although we described such practices in the press releases in a way that we hoped would make sense to the lay public, our sample may not have been sure what to make of this information. To gain a sense of what the sample knew about the ubiquity of such open and transparent practices, we asked participants the following two questions near the end of the experiment:
- True or False (or Unsure): scientists are usually required to post their data publicly, so that it may be analyzed and checked by other scientists (ans: false); and
- True or False (or Unsure): scientists are NOT required to specify what types of analyses they are going to do before collecting their data, which allows them to try several different options before reporting their results (ans: true).
Examining participants’ responses suggests that people are not really sure which practices are typical. Regarding the first item about data sharing, only 19.8% of the sample correctly answered that scientists are not usually required to post data, whereas close to half of the sample (48.1%) thought that scientists are required to do this (32% were uncertain). Regarding the second item about specifying analyses in advance, only 31.6% of the sample correctly stated that scientists are not required to specify the types of analyses they are going to do in advance, whereas 28.3% stated that scientists do have to do this and 40% were uncertain. Importantly, the experimental manipulations did not seem to affect participants’ responses to these items: chi-square tests demonstrate that participants’ responses do not vary based on the experimental condition to which they were randomly assigned (question 1: , ; question 2: , ). See Figure 2 .
These results suggest that, at least among those in this sample and using these questions, people are not familiar enough with open and transparent research practices (or cognizant that they are not already the norm) for awareness of such practices to drastically increase trust. People’s knowledge about the process of science and about open and transparent research practices should be further explored.
4 Discussion
Innovation of new GM products can incorporate responsible innovation practices in at least two ways. First, by incorporating public values when developing new technologies; and second, by incorporating safe-by-design practices into the development and testing of new GM products, such as those that can help mitigate the effects of human bias (e.g., conflict of interest).
Although public values should be taken into consideration when developing new technologies, to keep such discussions productive, stakeholders should focus on weighing different value perspectives and managing risk and not on disproportionate fears exacerbated by suspicion and misinformation. Otherwise, bending to unwarranted fears in the face of new technology less resembles responsible innovation than irresponsible enabling. Incorporating safe-by-design practices into the development of GM products may encourage more productive dialogue and remove reasons for suspicion that research on GMOs is negatively influenced by conflicts of interest. Our study finds support for our hypothesis that one way to justifiably increase perceptions of trustworthiness of the researchers and organizations involved with GM technology is to report when they are engaging in open and transparent research practices.
Consistent with prior studies, the current study finds that people place greater trust in universities, as opposed to corporations, to provide credible, knowledgeable, and ethical research and development of GMOs. Additionally, open-science disclaimers in press releases may have a positive, albeit small and somewhat inconsistent, influence on perceived trustworthiness. In contrast, pre-existing attitudes toward the safety, risks, and benefits of GMOs were generally associated with the perceived trustworthiness (knowledge and ethical behaviors) of researchers.
Although open-science disclaimers did have some positive effects on trustworthiness, we were surprised that the effects were not larger. Two factors may have limited the influence of the open and transparent disclaimer. First, the disclaimer is small and resembles boilerplate press release material. Thus, it may not have sufficiently captured participants’ attention. Future research might examine the influence of more visually salient cues for open and transparent research, such as the badges designed by the Center for Open Science for attaching to research articles [COS, 2016 ]. Alternatively, future research should consider using a format more traditional for public consumption than a press release, such as a news article, print advertisement, or commercial. Second, our exploratory analyses suggest that participants may not be familiar with the distinction between open-practices and typical ones. A plurality of participants thought that data-sharing was already standard practice in research. Thus, the open and transparent disclaimer may have been interpreted as indicating “business as usual.” Additionally, the benefits of preregistration may still be unclear to respondents. The hazards of adjusting analysis plans after data have been collected and explored are likely too technical to be a primary cause of public distrust in corporations developing GMOs. Nevertheless, this research highlights a potential benefit of engaging in open and transparent research practices: an increase in public trust. In industries on which public opinion exerts considerable pressure on policymakers (e.g., agricultural biotechnology), an organization’s ability to innovate rests not only on the quality and benefits of the innovation, but on its ability to elicit public confidence [Hicks, 1995 ; Marcus, 2015 ].
The desirability of these open and transparent practices is well established. As noted by Marcia McNutt, former editor-in-chief of Science and current president of the National Academies of Science, Engineering, and Medicine, “Nothing matters more than a good reputation in science. Always take the high road and strive for openness and transparency” (Stanford Medicine News Center, 2016). Likewise, a recent report from the National Academies of Science, Engineering, and Medicine (NASEM) recommends that gene drive research embrace transparency as a crucial component of public engagement [NASEM, 2016a ].
Although it is clear that open and transparent research practices pose challenges for corporate and corporate-sponsored research [e.g., Jasny et al., 2017 ], it may be possible to apply them to some types of research (e.g., safety testing), even if they reasonably cannot be applied to others (e.g., product development). Mars, Inc., maker of M&Ms and Wrigley’s gum, recently announced that they would not tie research funding to specific outcomes and support studies that can be published freely, regardless of its results [Prentice, 2018 ]. The Vice President of Public Affairs for Mars, Inc., stated that they “do not want to be involved in advocacy-led studies that so often, and mostly for the right reasons have been criticized” [Prentice, 2018 ].
Innovators in biotechnology areas must understand the societal implications of their research; developing and implementing these technologies will depend on productive dialogue with a wary public. Reporting the incorporation of openness and transparency into research practices can lend credibility and increase the likelihood that future research is perceived as responsible innovation rather than as industry advocacy.
A Sample information and exclusion criteria
Participants for this experiment were recruited by Research Now (RN), an online data collection company, from their US Consumer panel. Data was collected between June 20 and June 27, 2016.
To compensate panel participants, RN uses an incentive-scale based on the length of the survey and the panelists profile. Panel participants that are considered “time-poor/money-rich” are paid significantly higher incentives per completed survey than the average panelist so that participating is attractive enough to be perceived as worth the time investment. The incentive options allow panel participants to redeem from a range of options such as gift cards, point programs, and partner products and services.
We requested a sample of 1200 participants. To obtain this sample, RN emailed 18,230 of their panelists; 1,761 opened the email, 1,659 started the survey, and 1,199 participants were coded as “completes”. Participants who were not coded as complete were not paid for and were excluded from the study.
In order to be coded as “complete” participant had to meet the following criteria.
First, we excluded participants who did not click through to the end of the survey (participants could skip questions they preferred not to answer them) and submit their responses (n = 346; remaining participants = 1,313).
Second, we excluded participants who were suspected of “speeding” through the survey (i.e., clicking random options to quickly get through the survey and receive their incentive payment). As the survey was designed to take 20 to 25 minutes, and the median response time was 20 minutes, participants who took less than 7 minutes (n = 58) were excluded and participants who took less than 10 minutes (but more than 7) and missed two or more of the reading check questions (n = 56) were also excluded from being coded as complete (remaining sample = 1,199).
When coding participants as “complete”, we did not take into account participants who took too long on the survey. Thus, 8 participants were excluded from the study who were 2 standard deviations above the average number of minutes spent taking the survey (Original Sample: minutes, minutes, ). In addition, 94 participants in the two open & transparent conditions who missed the manipulation check item (i.e., recognizing that the organization engaged in open and transparent research practices) were also excluded. The final sample used for analysis consisted of 1097 participants.
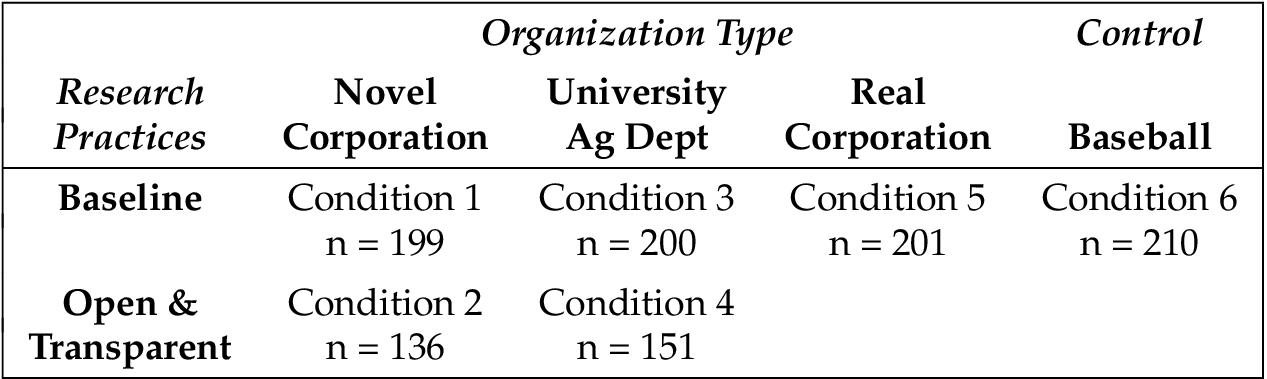
B Test for demographic differences between conditions
Because random assignment does not guarantee that people of varying demographics will be evenly distributed amongst the conditions, we tested to ensure that each demographic variable did not vary amongst the six conditions. To do this, we used one-way ANOVAs to test the continuous variables (education, age, religion, ideology, and income) and chi-square analyses to test the binary variables (gender, Hispanic/Latino, black). No differences were found.
C Measuring attitudes toward and knowledge about GMOs
C.1 Measuring attitudes toward GMOs
To measure attitudes toward GMOs, we asked participants what they thought about the safety of GMOs, about several potential risks and benefits of GMOs, about banning GM technology, about labeling GMOs, about regulating GMOs, and whether the participant avoids eating GMOs.
C.1.1 Safety
Participants were asked to state whether they believed that GMOs were safe where 4 was “GMOs are as safe as conventional crops” and 0 was “GMOs are NOT as safe as conventional crops” ( , , ). A one-way ANOVA shows no significant differences between perceptions of GMO safety across the six conditions, , .
C.1.2 Risks and benefits
Participants to rate a set of four specific potential risks and four specific potential benefits of GM Os. Participants first had to say “how likely this is to be a real risk/benefit from GMO technology”. The responses for this item were coded to be a proxy for the participant’s perception of base rate, where we coded not at all likely as .25, somewhat likely as .50, and very likely as .75. Then, participants were asked to rate “how dangerous/helpful is this risk/benefit, if it is a true risk/benefit”. These responses were coded to be degree of dangerousness or helpfulness, where we coded a little dangerous/helpful as 1, moderately dangerous/helpful as 2, and extremely dangerous/helpful as 3. We then multiplied these two values together, allowing us to weight each of the perceptions of how helpful a benefit or dangerous a risk is with the perceived base rate.
The four risk items were: increased herbicide use, increased allergens, antibiotic resistance, and unpredictability. These items were internally consistent (alpha = .82) and loaded onto one factor in a principal axis factor analysis. Moreover, the items generally show similar relationships as one another with age, education, and safety. 12
The four benefit items were: improving nutrition, saving crops from viruses, combatting disease, and protecting the environment. These items also loaded onto one factor and had high internal consistency (alpha = .85). Moreover, like the risk items, the benefit items show similar relationships as one another with age, education, and perceptions of safety.
Therefore, we combined these items into an averaged index of GMO risk perceptions ( , , ) and an averaged index of GMO benefit perceptions ( , , ), both of which were on a scale from 0.25 to 2.25. One-way ANOVAs show no significant differences of GMO risk perceptions or GMO benefit perceptions across the six conditions: GMO risks: , ; GMO benefits: , .
C.1.3 Banning GMO technology
Participants were also asked whether GMO technology should be (a) banned in all circumstances, (b) banned for making food items, but allowed for making non-food items, or (c) should not be banned. We recoded this into two variables: one representing whether the participant believes in banning all GM technology (10.8% of the sample) and one representing whether the participant believes in banning GM food only (40.8% of the sample). A chi-square test showed no significant differences in proportion of participants supporting the banning of all GM technology across the six conditions, , . In addition, a chi-square test showed no significant differences in proportion of participants supporting the banning of GM foods across the six conditions, , .
C.1.4 Labeling GMOs
Participants were told that some people believe GMOs should be labeled because it gives consumers a choice in what they purchase and eat, whereas other people believe products containing GMOs should NOT be labeled because it intensifies the misperception that GMOs on the market are toxic or allergenic (the order of these two beliefs was randomized between participants). Then, participants were asked whether products containing GMOs should or should not be labeled. About 90% of participants said that GMO products should be labeled. A chi-square test showed no significant differences in proportion of participants supporting the labeling of GMOs across the six conditions, , .
C.1.5 Regulating GM technology
Participants were told that some people believe that the Environmental Protection Agency (EPA) and the Food and Drug Administration (FDA) should strictly regulate GMOs to make sure that there are no environmental or health risks, whereas other people believe that GMOs should NOT be regulated because they have been shown to be as safe as conventional crops and conventional crops are not regulated (the order of these two was randomized between subjects 13 ). Then, participants were asked whether GMOs should be regulated or not. About 85% of participants in the study said that GMOs should be regulated.
A chi-square test showed no significant differences in proportion of participants supporting the regulation of GMOs across the six conditions, , .
C.1.6 Avoid eating GMOs
Participants were asked if they purposefully avoid eating GMOs or not (regardless of whether or not they have ever eaten GMOs). About 34% of participants in the study said that they purposefully avoid eating GMOs. A chi-square test showed no significant differences in proportion of participants avoiding GMOs across the six conditions, , .
C.2 Measuring GMO knowledge
To measure knowledge about GMOs, we asked participants how much they have heard about GMOs (i.e., familiarity), what they believe is the scientific consensus of GMO safety, and what process is used to make GMOs.
C.2.1 Familiarity
Participants were asked how much they would say that they have heard or read about GMOs on a scale from 1 (not very much at all) to 5 (a great deal). Participants across all conditions had a mean familiarity of 3.11 (“some”) and a . A one-way ANOVA showed no significant differences in familiarity with GMOs across the six conditions, F (5, 1185) = 0.712, .
C.2.2 Process
Participants were asked, to the best of their knowledge, which best describes the process used to create genetically-modified organisms: 14
- Plants are mated or cross-pollinated with other plants in order to create more desirable traits [ cross-pollination ].
- Plant genomes are subjected to radiation treatments to induce changes to genes in order to create more desirable traits [ mutagenesis ].
- A specific gene or sequence of genes is targeted and either “turned-off”, “turned-on”, or exchanged to create more desirable traits [ gene-editing ].
We dummy coded the variable so that we had a variable for those who thought it was mutagenesis (10.4%) and one for those who chose gene-editing (45.3%). A chi-square analysis showed no significant differences in proportion of participants choosing mutagenesis across the six conditions, X (5) = 3.33, ; nor was there significant differences in the proportion of participants choosing gene editing across the six conditions, X (5) = 8.40, .
C.2.3 Consensus
Participants were also asked what most scientists view of the safety of GMOs is on a scale from -2 (GMO’s are not as safe to eat as conventional crops) to 2 (GMOs are as safe to eat as conventional crops), with “uncertain about the safety of GMOs” (0) in the middle. We recoded this variable into one called “consensus”, such that the two responses that indicated that GMOs are as safe to eat as conventional crops (2 and 1) were given a 1 and the other responses were given 0s. A little over half of the subjects recognized that scientific consensus currently is that GMOs are as safe to eat as conventional crops (51%). A chi-square analysis showed no significant differences in proportion of participants correctly recognizing what the scientific consensus is across the six conditions, , .
D Example press releases used in the experiment
E Changes to credibility scale from Critchley [2008]
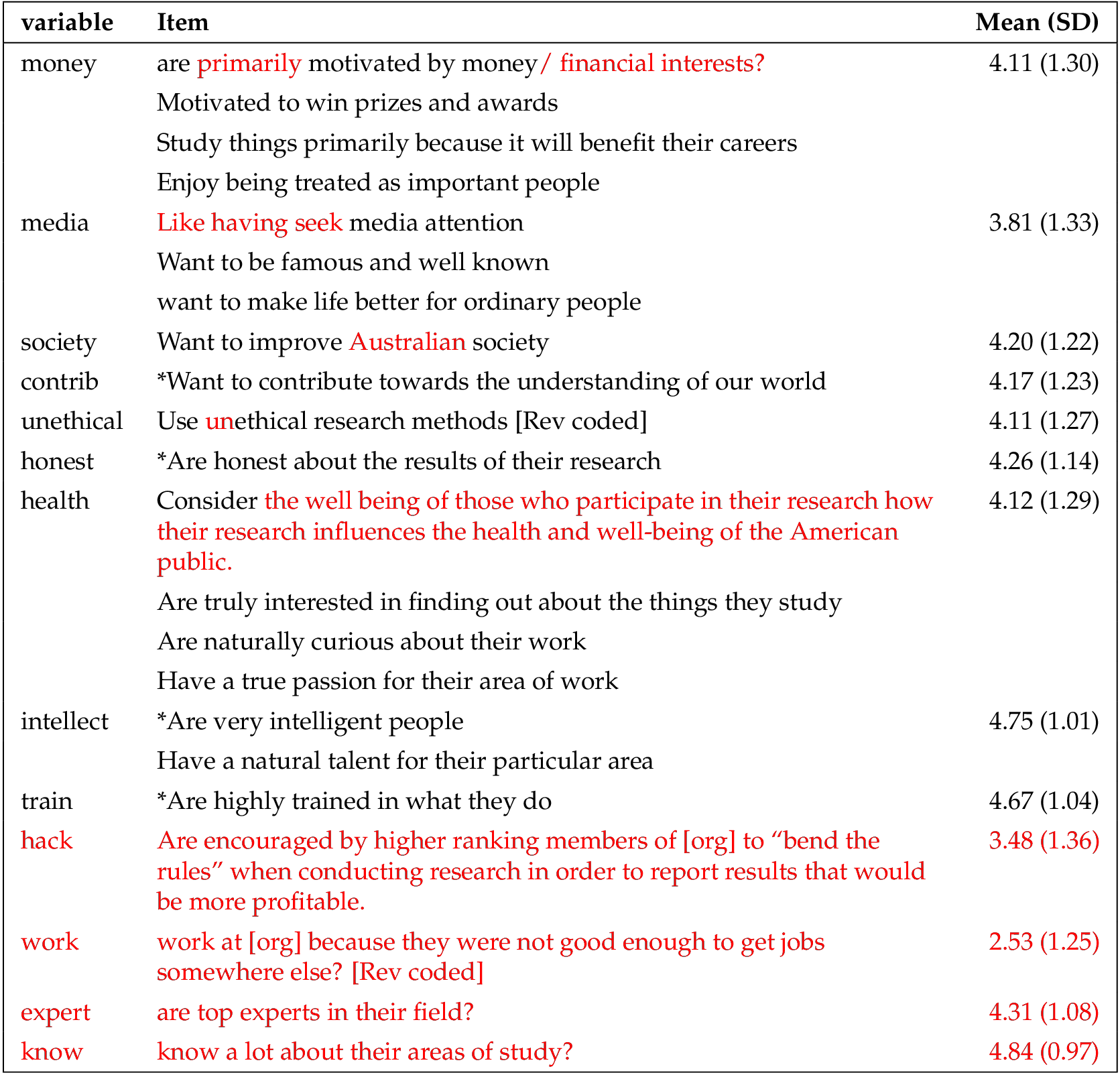
References
-
Alberts, B., Cicerone, R. J., Fienberg, S. E., Kamb, A., McNutt, M., Nerem, R. M., Schekman, R., Shiffrin, R., Stodden, V., Suresh, S., Zuber, M. T., Pope, B. K. and Jamieson, K. H. (2015). ‘Self-correction in science at work’. Science 348 (6242), pp. 1420–1422. https://doi.org/10.1126/science.aab3847 .
-
APPC & Pew Research Center (2015). Pew American Trends Panel - Wave 11.
-
Bailey, M. (2016). ‘How the Sugar Industry Artificially Sweetened Harvard Research’. PBS NewsHour . URL: https://www.pbs.org/newshour/health/sugar-industry-artificially-sweetened-harvard-research .
-
Bain, C. and Selfa, T. (2017). ‘Non-GMO vs organic labels: purity or process guarantees in a GMO contaminated landscape’. Agriculture and Human Values 34 (4), pp. 805–818. https://doi.org/10.1007/s10460-017-9776-x .
-
Baram, M. (2007). ‘Liability and its influence on designing for product and process safety’. Safety Science 45 (1-2), pp. 11–30. https://doi.org/10.1016/j.ssci.2006.08.022 .
-
Baron, J. (2000). Thinking and deciding. 3rd ed. New York, U.S.A.: Cambridge University Press.
-
Basken, P. (2017). ‘Under fire, National Academies toughen conflict-of-interest policies’. Chronicle of Higher Education . URL: http://www.chronicle.com/article/Under-Fire-National-Academies/239885 .
-
Chalmers, D. and Nicol, D. (2004). ‘Commercialisation of biotechnology: public trust and research’. International Journal of Biotechnology 6 (2/3), p. 116. https://doi.org/10.1504/ijbt.2004.004806 .
-
COS (22nd June 2016). ‘Openness is a core value of scientific practice: Badges to acknowledge open practices’. Open Science Framework . URL: https://osf.io/tvyxz/wiki/home/ .
-
Critchley, C. R. (2008). ‘Public opinion and trust in scientists: the role of the research context, and the perceived motivation of stem cell researchers’. Public Understanding of Science 17 (3), pp. 309–327. https://doi.org/10.1177/0963662506070162 . PMID: 19069082 .
-
Critchley, C. R. and Nicol, D. (2011). ‘Understanding the impact of commercialization on public support for scientific research: Is it about the funding source or the organization conducting the research?’ Public Understanding of Science 20 (3), pp. 347–366. https://doi.org/10.1177/0963662509346910 .
-
DeAngelis, C. D. (2000). ‘Conflict of Interest and the Public Trust’. JAMA 284 (17), p. 2237. https://doi.org/10.1001/jama.284.17.2237 .
-
Diels, J., Cunha, M., Manaia, C., Sabugosa-Madeira, B. and Silva, M. (2011). ‘Association of financial or professional conflict of interest to research outcomes on health risks or nutritional assessment studies of genetically modified products’. Food Policy 36 (2), pp. 197–203. https://doi.org/10.1016/j.foodpol.2010.11.016 .
-
Dixon, G., McComas, K., Besley, J. and Steinhardt, J. (2016). ‘Transparency in the food aisle: the influence of procedural justice on views about labeling GM foods’. Journal of Risk Research 19 (9), pp. 1158–1171. https://doi.org/10.1080/13669877.2015.1118149 .
-
Domonoske, C. (2016). ‘50 Years Ago, Sugar Industry Quietly Paid Scientists to Point Blame at Fat’. NPR . URL: http://www.npr.org/sections/thetwo-way/2016/09/13/493739074/50-years-ago-sugar-industry-quietly-paid-scientists-to-point-blame-at-fat .
-
Fiske, S. T., Cuddy, A. J. and Glick, P. (2007). ‘Universal dimensions of social cognition: warmth and competence’. Trends in Cognitive Sciences 11 (2), pp. 77–83. https://doi.org/10.1016/j.tics.2006.11.005 .
-
Grayson, K., Johnson, D. and Chen, D.-F. R. (2008). ‘Is Firm Trust Essential in a Trusted Environment? How Trust in the Business Context Influences Customers’. Journal of Marketing Research 45 (2), pp. 241–256. https://doi.org/10.1509/jmkr.45.2.241 .
-
Gurney, S. and Sass, J. (2001). ‘Public trust requires disclosure of potential conflicts of interest’. Nature 413 (6856), pp. 565–565. https://doi.org/10.1038/35098242 .
-
Guston, D. H. (2015). ‘Responsible innovation: who could be against that?’ Journal of Responsible Innovation 2 (1), pp. 1–4. https://doi.org/10.1080/23299460.2015.1017982 .
-
Guston, D. H., Fisher, E., Grunwald, A., Owen, R., Swierstra, T. and Burg, S. van der (2014). ‘Responsible innovation: motivations for a new journal’. Journal of Responsible Innovation 1 (1), pp. 1–8. https://doi.org/10.1080/23299460.2014.885175 .
-
Gutteling, J., Hanssen, L., Veer, N. van der and Seydel, E. (2006). ‘Trust in governance and the acceptance of genetically modified food in the Netherlands’. Public Understanding of Science 15 (1), pp. 103–112. https://doi.org/10.1177/0963662506057479 .
-
Hallman, W. K. (2018). ‘Consumer Perceptions of Genetically Modified Foods and GMO Labeling in the United States’. In: Consumers’ Perception of Food Attributes. Ed. by S. Matsumoto and T. Otsuki. Boca Raton, U.S.A.: CRC Press. ISBN: 978-1138196841.
-
Hallman, W. K., Cuite, C. L. and Morin, X. K. (2013). Public Perceptions of Labeling Genetically Modified Foods. Working Paper 2013-01. The State University of New Jersey. New Brunswick, NJ, U.S.A.: Rutgers. https://doi.org/10.7282/t33n255n .
-
Hardwig, J. (1985). ‘Epistemic Dependence’. The Journal of Philosophy 82 (7), p. 335. https://doi.org/10.2307/2026523 .
-
Hendriks, F., Kienhues, D. and Bromme, R. (2016). ‘Trust in Science and the Science of Trust’. In: Progress in IS . Springer International Publishing, pp. 143–159. https://doi.org/10.1007/978-3-319-28059-2_8 .
-
Hicks, D. (1995). ‘Published Papers, Tacit Competencies and Corporate Management of the Public/Private Character of Knowledge’. Industrial and Corporate Change 4 (2), pp. 401–424. https://doi.org/10.1093/icc/4.2.401 .
-
Hovland, C., Janis, I. L. and Kelley, H. H. (1953). ‘Communication and Persuasion’. In: Psychological Studies of Opinion Change. New Haven, CT, U.S.A.: Yale University Press.
-
Jasny, B. R., Wigginton, N., McNutt, M., Bubela, T., Buck, S., Cook-Deegan, R., Gardner, T., Hanson, B., Hustad, C., Kiermer, V., Lazer, D., Lupia, A., Manrai, A., McConnell, L., Noonan, K., Phimister, E., Simon, B., Strandburg, K., Summers, Z. and Watts, D. (2017). ‘Fostering reproducibility in industry-academia research’. Science 357 (6353), pp. 759–761. https://doi.org/10.1126/science.aan4906 .
-
Johnson, N. (2015). It’s practically impossible to define “GMOs” . Grist.org. URL: https://grist.org/food/mind-bomb-its-practically-impossible-to-define-gmos/ .
-
Kaplan, R. M. and Irvin, V. L. (2015). ‘Likelihood of Null Effects of Large NHLBI Clinical Trials Has Increased over Time’. PLOS ONE 10 (8), e0132382. https://doi.org/10.1371/journal.pone.0132382 .
-
Kearns, C. E., Schmidt, L. A. and Glantz, S. A. (2016). ‘Sugar Industry and Coronary Heart Disease Research’. JAMA Internal Medicine 176 (11), p. 1680. https://doi.org/10.1001/jamainternmed.2016.5394 .
-
Kerr, N. L. (1998). ‘HARKing: Hypothesizing After the Results are Known’. Personality and Social Psychology Review 2 (3), pp. 196–217. https://doi.org/10.1207/s15327957pspr0203_4 .
-
Koehler, J. J. (1993). ‘The Influence of Prior Beliefs on Scientific Judgments of Evidence Quality’. Organizational Behavior and Human Decision Processes 56 (1), pp. 28–55. https://doi.org/10.1006/obhd.1993.1044 .
-
Krimsky, S. and Schwab, T. (2017). ‘Conflicts of interest among committee members in the National Academies’ genetically engineered crop study’. PLOS ONE 12 (2), e0172317. https://doi.org/10.1371/journal.pone.0172317 .
-
Kunda, Z. (1990). ‘The case for motivated reasoning’. Psychological Bulletin 108 (3), pp. 480–498. https://doi.org/10.1037/0033-2909.108.3.480 .
-
Landrum, A. R., Eaves, B. S. and Shafto, P. (2015). ‘Learning to trust and trusting to learn: a theoretical framework’. Trends in Cognitive Sciences 19 (3), pp. 109–111. https://doi.org/10.1016/j.tics.2014.12.007 .
-
Lang, J. T. and Hallman, W. K. (2005). ‘Who Does the Public Trust? The Case of Genetically Modified Food in the United States’. Risk Analysis 25 (5), pp. 1241–1252. https://doi.org/10.1111/j.1539-6924.2005.00668.x .
-
MacCoun, R. J. (1998). ‘Biases in the interpretation and use of research results’. Annual Review of Psychology 49 (1), pp. 259–287. https://doi.org/10.1146/annurev.psych.49.1.259 .
-
Marcus, E. (2015). ‘Credibility and Reproducibility’. Structure 23 (1), pp. 1–2. https://doi.org/10.1016/j.str.2014.12.001 .
-
Myhr, A. I. and Traavik, T. (2003). ‘Genetically modified (GM) crops: precautionary science and conflicts of interests’. Journal of Agricultural and Environmental Ethics 16 (3), pp. 227–247.
-
NASEM (2016a). Gene Drives on the Horizon: Advancing Science, Navigating Uncertainty, and Aligning Research with Public Values. Washington, DC, U.S.A.: National Academies Press. https://doi.org/10.17226/23405 .
-
— (2016b). Genetically Engineered Crops: Experiences and Prospects. Washington, DC, U.S.A.: National Academies Press. https://doi.org/10.17226/23395 .
-
Nosek, B. A., Alter, G., Banks, G. C., Borsboom, D., Bowman, S. D., Breckler, S. J., Buck, S., Chambers, C. D., Chin, G., Christensen, G., Contestabile, M., Dafoe, A., Eich, E., Freese, J., Glennerster, R., Goroff, D., Green, D. P., Hesse, B., Humphreys, M., Ishiyama, J., Karlan, D., Kraut, A., Lupia, A., Mabry, P., Madon, T., Malhotra, N., Mayo-Wilson, E., McNutt, M., Miguel, E., Paluck, E. L., Simonsohn, U., Soderberg, C., Spellman, B. A., Turitto, J., VandenBos, G., Vazire, S., Wagenmakers, E. J., Wilson, R. and Yarkoni, T. (2015). ‘Promoting an open research culture’. Science 348 (6242), pp. 1422–1425. https://doi.org/10.1126/science.aab2374 .
-
O’Connor, A. (2016). How the Sugar Industry Shifted Blame to Fat . URL: http://www.nytimes.com/2016/09/13/well/eat/how-the-sugar-industry-shifted-blame-to-fat.html .
-
Pornpitakpan, C. (2004). ‘The Persuasiveness of Source Credibility: A Critical Review of Five Decades’ Evidence’. Journal of Applied Social Psychology 34 (2), pp. 243–281. https://doi.org/10.1111/j.1559-1816.2004.tb02547.x .
-
Prentice, C. (2018). ‘M&M’s Maker Publishes Science Policy in Bid to Boost Transparency’. Food Quality & Safety . URL: http://www.foodqualityandsafety.com/article/mms-maker-publishes-science-policy-bid-boost-transparency/ .
-
Rodman, M. C. (2016). ‘Holes in Harvard Sugar Study Expose Dangers of Industry Funding’. Harvard Crimson . URL: http://www.thecrimson.com/article/2016/9/14/sugar-study-exposes-dangers/ .
-
Schumaker, E. (2016). ‘Harvard’s Sugar Industry Scandal Is Just the Tip of the Iceberg’. The Huffington Post . URL: http://www.huffingtonpost.com/entry/sugar-harvard-scandal-nutrition-study_us_57d8088ee4b0aa4b722c6417 .
-
Scott, S. E., Inbar, Y. and Rozin, P. (2016). ‘Evidence for Absolute Moral Opposition to Genetically Modified Food in the United States’. Perspectives on Psychological Science 11 (3), pp. 315–324. https://doi.org/10.1177/1745691615621275 .
-
Shanker, D. (2016). ‘How Big Sugar Enlisted Harvard Scientists to Influence How We Eat–in 1965’. Bloomberg . URL: http://www.bloomberg.com/news/articles/2016-09-12/how-big-sugar-enlisted-harvard-scientists-to-influence-how-we-eat-in-1965 .
-
Siegrist, M. (2000). ‘The Influence of Trust and Perceptions of Risks and Benefits on the Acceptance of Gene Technology’. Risk Analysis 20 (2), pp. 195–204. https://doi.org/10.1111/0272-4332.202020 .
-
Sifferlin, A. (2016). ‘How the Sugar Lobby Skewed Health Research’. Time Magazine . URL: http://time.com/4485710/sugar-industry-heart-disease-research/ .
-
Simmons, J. P., Nelson, L. D. and Simonsohn, U. (2011). ‘False-Positive Psychology’. Psychological Science 22 (11), pp. 1359–1366. https://doi.org/10.1177/0956797611417632 .
-
Spies, J. R. (2013). ‘The Open Science Framework: Improving Science by Making It Open and Accessible’. PhD thesis. https://doi.org/10.18130/v35c6x .
-
Stilgoe, J., Owen, R. and Macnaghten, P. (2013). ‘Developing a framework for responsible innovation’. Research Policy 42 (9), pp. 1568–1580. https://doi.org/10.1016/j.respol.2013.05.008 .
-
Taebi, B., Correljé, A., Cuppen, E., Dignum, M. and Pesch, U. (2014). ‘Responsible innovation as an endorsement of public values: the need for interdisciplinary research’. Journal of Responsible Innovation 1 (1), pp. 118–124. https://doi.org/10.1080/23299460.2014.882072 .
-
Tagliabue, G. (2016). ‘The necessary “GMO” denialism and scientific consensus. Journal of Science Communication’. JCOM 15 (4), Y01. URL: https://jcom.sissa.it/archive/15/04/JCOM_1504_2016_Y01 .
-
Welchman, J. (2007). ‘Frankenfood, or, Fear and Loathing at the Grocery Store’. Journal of Philosophical Research 32 (9999), pp. 141–150. https://doi.org/10.5840/jpr_2007_14 .
-
Wilpert, B. (2007). ‘Psychology and design processes’. Safety Science 45 (1-2), pp. 293–303. https://doi.org/10.1016/j.ssci.2006.08.016 .
Authors
Asheley R. Landrum is a social-cognitive psychologist and assistant professor of science communication in the College of Media & Communication at Texas Tech University. Her research investigates how cultural values and worldviews influence people’s perceptions of science, and how these perceptions develop from childhood into adulthood. This work bridges theories from psychology, political science, communication, and public policy. E-mail: asheley.landrum@ttu.edu .
Joseph Hilgard is an assistant professor in the Department of Psychology at Illinois State University. In addition to his research on the effects of playing video games, Dr. Hilgard examines questions about research itself, such as what proportion of research findings are true and how we can make scientific publication more fair and more truthful. E-mail: jhilgard@gmail.com .
Robert B. Lull is an assistant professor in the Department of Communication at California State University, Fresno. Dr. Lull’s research addresses the intersections of science communication, risk communication, and strategic communication, focusing on the influence of emotional arousal on information processing and risk perceptions. E-mail: lull@mail.fresnostate.edu .
Heather Akin is an assistant professor with appointments in the Missouri School of Journalism and the College of Agriculture, Food, and Natural Resources at the University of Missouri. Dr. Akin’s research examines the role of motivated reasoning and media in influencing public perceptions about topics ranging in scope from the very small (e.g., materials at the nanoscale) to global issues like climate change. E-mail: heathereakin@gmail.com .
Kathleen Hall Jamieson is the Elizabeth Ware Packard Professor of Communication at the University of Pennsylvania’s Annenberg School for Communication, the Walter and Leonore Director of the university’s Annenberg Public Policy Center, and Program Director of the Annenberg Retreat at Sunnylands. She recently co-edited The Oxford Handbook of Political Communication (2017) and The Oxford Handbook of the Science of Science Communication (2017). Jamieson’s work has been funded by the FDA and the MacArthur, Ford, Carnegie, Pew, Robert Wood Johnson, Packard, and Annenberg Foundations. She is the co-founder of FactCheck.org and its subsidiary site, SciCheck, and director of The Sunnylands Constitution Project, which has produced more than 30 award-winning films on the Constitution for high school students. Jamieson is a fellow of the American Academy of Arts and Sciences, the American Philosophical Society, the American Academy of Political and Social Science, and the International Communication Association, and a past president of the American Academy of Political and Social Science. E-mail: Kjamieson@asc.upenn.edu .
Endnotes
1 http://www.wholefoodsmarket.com/our-commitment-gmo-transparency
2 https://www.benjerry.com/values/issues-we-care-about/support-gmo-labeling/our-non-gmo-standards
3 https://www.chipotle.com/gmo
4 Participants were part of the wave 11 Pew American Trends Panel, a probability based online sample of 3,057 U.S. adults, surveyed between June 8, 2015 and July 29, 2015. Note that attrition does occur for each wave of the American Trends Panel, but the sample has remained demographically and ideologically diverse. For more information, see http://www.pewresearch.org/methodology/u-s-survey-research/american-trends-panel/
5 Of the remaining participants, about 30% reported either not being sure or never having heard of Monsanto and 6.4% refused to answer.
6 Press releases often are intended for journalists, not the public. Instead, members of the public read the articles composed by journalists based on the press releases. In this sense, our experimental design is somewhat artificial. However, we wanted to provide participants with a much shorter passage to read than an article that also clearly identifies the organization involved. We also wanted to remove any intermediary, as adding a journalist or media channel adds another source to be evaluated, and thus could complicate interpretation of our results. We thought that a shortened press release was the best way to achieve our objectives.
7 The press release material for Monsanto was constructed from real press release material, but was shortened so that the length matched the other conditions and to increase the likelihood that participants read the press release.
8 A survey from the Pew American Trends Panel shows that close to 70% of participants have heard of Monsanto [APPC & Pew Research Center, 2015 ].
9 We excluded the sixth baseball control condition from this analysis.
10 Note that because we are reporting the final betas, these results for the experimental manipulations control for the effects of GMO attitudes, policy positions, and knowledge.
11 Perceptions of risk did not predict ratings of researchers’ competence, but did significantly predict all other dependent variables.
12 Relationships with age and education are all non-significant except for the risks of antibiotic resistance item, which was significantly, positively related to education ( , ) and age ( , ).
13 The order of the presented beliefs about labeling that were described prior to asking participants what their own beliefs about labeling did not influence participants’ responses, , .
14 The labels shown here in brackets were not shown to participants.