1 Review of the literature: setting the record straight
To provide some context for the reader into the nature of Kahan’s [ 2017 ] concerns about our work, we start by briefly outlining Kahan’s own position in this on-going debate. The “cultural cognition of scientific consensus” thesis predicts that exposing the public to information about the scientific consensus will only further attitude polarization by reinforcing people’s “cultural predispositions” to selectively attend to evidence [Kahan, Jenkins-Smith and Braman, 2011 ]. This prediction, by and large, has failed to materialize in empirical studies that specifically examine public reactions to scientific consensus statements on a range of issues. For example, in the context of climate change, research has actually found the opposite: highlighting scientific consensus neutralizes polarizing worldviews [Lewandowsky, Gignac and Vaughan, 2013 ; Cook and Lewandowsky, 2016 ; Cook, Lewandowsky and Ecker, 2017 ; van der Linden et al., 2015 ; van der Linden et al., 2017b ; van der Linden, Leiserowitz and Maibach, 2017 ].
Other research has found that communicating the scientific consensus has positive effects across the political spectrum in the context of both climate change [Deryugina and Shurchkov, 2016 ; Myers et al., 2015 ] and vaccines [van der Linden, Clarke and Maibach, 2015 ]. Still other work has found that conveying the scientific consensus on climate change has greater effects among those with low environmental interest [Brewer and McKnight, 2017 ] and has positive effects among evangelical Christians [Webb and Hayhoe, 2017 ]. Conversely, conveying even a small amount of scientific dissent directly undermines support for environmental policy [Aklin and Urpelainen, 2014 ].
In his opening review of the evidence, Kahan [ 2017 ] fails to mention most of this body of work, despite the fact that the National Academies of Sciences [ 2017 ] has called for more such research [p. 20]. Kahan [ 2017 ] does discuss a few studies in his introduction, but his discussion is highly selective and mischaracterizes the evidence. For example, in discussing Bolsen and Druckman [ 2017 ], Kahan [ 2017 ] fails to mention key conclusions such as; “ The gateway model’s mediational predictions are supported ” [p. 13], or “ a scientific consensus statement leads all partisan subgroups to increase their perception of a scientific consensus ” [p. 14]. Similarly, although not cited by Kahan [ 2017 ], Brewer and McKnight [ 2017 ] find independent support for the Gateway Belief Model (GBM)’s mediational hypotheses, as does Dixon [ 2016 ] in the context of GMO’s. Cook and Lewandowsky [ 2016 ] indeed found negative effects (but only for their US sample) and among a subset of respondents characterized as extreme free-market endorsers. However, Kahan [ 2017 ] neglects to mention that Cook, Lewandowsky and Ecker [ 2017 ] then failed to replicate this backfire-effect in a larger US sample, where they found that information about the scientific consensus partially neutralized the influence of free-market ideology. Dixon [ 2016 ] notes that the effect of conveying a scientific consensus message on GMO safety was smaller among audiences with skeptical prior attitudes, but importantly, no actual backfire-effect was observed. It is interesting that Kahan [ 2017 ] cites Dixon, Hmielowski and Ma [ 2017 ] to support his claims, as, contrary to Kahan’s argument, this study did not replicate our work (e.g., perceived consensus was not measured), yet the authors do clearly state that; “ It is notable that a backfiring effect among conservatives was not observed ” [p. 7].
In sum, we think it is fair to conclude that the evidence to date is strongly in favor of the Gateway Belief Model (GBM) while there is little to no evidence to support the polarizing predictions flowing from the cultural cognition of scientific consensus thesis. In fact, Hamilton [ 2016 ] reports that in state-level data, both public awareness of the scientific consensus and belief in human-caused climate change have grown in tandem over the last six years, while the liberal-conservative gap on the existence of a scientific consensus has actually decreased . More generally, research has found that providing the public with scientific information about climate change does not backfire [Bedford, 2015 ; Johnson, 2017 ; Ranney and Clark, 2016 ], and questions have been raised about the validity of the cultural cognition thesis [van der Linden, 2015 ], including failed replications of the “motivated numeracy effect” [Ballarini and Sloman, 2017 ]. 1 Moreover, the specific conditions under which directionally motivated reasoning and belief polarization occurs is much more nuanced than previously thought [Flynn, Nyhan and Reifler, 2017 ; Guess and Coppock, 2016 ; Hill, 2017 ; Kuhn and Lao, 1996 ; Wood and Porter, 2016 ].
Nonetheless, we agree with Kahan’s [ 2017 ] remark that this exchange is not about reviewing the literature on consensus messaging. However, in our view, Kahan [ 2017 ] has greatly distorted the weight of evidence in his article and we felt compelled to correct this for the reader here. Moreover, we should clarify that our position is not that communicating the scientific consensus has no potential to backfire among some people with extremely motivated beliefs. However, our data, and that of several other independent teams of investigators, shows that, on average, no such back-fire effect occurs across the political spectrum.
Importantly, we have also urged scholars not to create false dilemmas of the “culture vs. cognition” kind [van der Linden et al., 2017a ] as it is possible — indeed likely — that both cultural and cognitive factors simultaneously influence people’s beliefs and attitudes about contested societal issues. The apparent conflict between the “scientific consensus as a gateway cognition” vs. the “cultural cognition of scientific consensus” thesis is clearly a false dilemma as these two processes are not mutually exclusive. Indeed, as Flynn, Nyhan and Reifler [ 2017 ] state; “the relative strength of directional vs. accuracy motivations can vary substantially between individuals and across contexts” [p. 134]. For example, the average updating of beliefs in line with the scientific consensus does not in itself preclude motivated reasoning from occurring [Bolsen and Druckman, 2017 ] nor does it mean that the scientific consensus cannot be communicated by appropriate in-group members to help overcome cultural biases [e.g. see Brewer and McKnight, 2017 ; Webb and Hayhoe, 2017 ] Moreover, in related research, we and others have shown that information about the scientific consensus can be neutralized when competing claims (based on misinformation) are presented, but that inoculation (i.e., forewarning) techniques can successfully help counteract such efforts to politicize science [Bolsen and Druckman, 2015 ; Cook, Lewandowsky and Ecker, 2017 ; van der Linden et al., 2017b ].
This line of research also demonstrates that although accuracy-motivation is often present, it is easily undermined by misinformation, selective exposure, and false media balance [Koehler, 2016 ; Krosnick and MacInnis, 2015 ]. Indeed, the decades-long climate disinformation campaigns organized by vested-interest groups — the key purpose of which were to create doubt and confusion about the scientific consensus — have limited action on global warming [Oreskes and Conway, 2010 ]. Accordingly, survey research indicates that only 13% of Americans correctly understand that the scientific consensus ranges between 90% to 100% and that this understanding is about five times higher among liberals than conservatives [Leiserowitz et al., 2017 ]. Even many US science teachers remain largely unaware of the scientific consensus and continue teaching “both sides of the debate” [Plutzer et al., 2016 ]. We therefore conclude that there is a strong and compelling rationale for communicating the scientific consensus that human-caused climate change is happening.
2 The Gateway Belief Model (GBM)
Kahan [ 2017 ] has independently confirmed our key finding: exposing people to a simple cue about the scientific consensus on climate change led to a significant pre-post change in public perception of the scientific consensus (12.8%, Cohen’s ). Unfortunately, in his commentary, Kahan [ 2017 ] also misrepresents the nature of our model, its assumptions, and other findings reported in van der Linden et al. [ 2015 ]. Prior correlational survey studies have reported that the public’s perception of the scientific consensus on human-caused global warming is associated with other key beliefs people have about the issue [Ding et al., 2011 ; McCright, Dunlap and Xiao, 2013 ] and acceptance of science more generally [Lewandowsky, Gignac and Vaughan, 2013 ]. The theoretical approach in our paper was therefore confirmatory and directly built on this line of research by providing experimental evidence for the “Gateway Belief Model” (GBM).
Using national data (N 1,104) from a consensus-message experiment, we found that increasing public perceptions of the scientific consensus is significantly associated with an increase in the belief that climate change is happening, human-caused, and a worrisome threat (Stage 1). In turn, changes in these key beliefs predicted increased support for public action (Stage 2). In short, the GBM is a two-stage sequential 2 mediation model based on causal data and we confirmed that perceived scientific consensus is an important gateway cognition, influencing public support for climate change. Kahan [ 2017 ] argues (a) that we did not evaluate the direct effects of our experimental treatment on any variable other than subjects’ subsequent perceived scientific consensus (e.g., not on the mediator or outcome variables); (b) in his analyses, he finds no significant differences in the values of the mediator variables (personal beliefs) and final outcome variable (support for action) between the treatment and control groups; and (c) he claims that this lack of significance matters, because, a significant direct effect of the treatment message on the mediator variables must be established for mediation to occur between the treatment message, the personal beliefs, and final outcome variable. We respond to these criticisms in detail below and demonstrate that his assertions are incorrect.
2.1 Philosophical and empirical perspectives on mediation analysis
Kahan [ 2017 ] claims that the consensus message 3 did not directly impact the key (mediating) variables in the model, namely; (a) the belief that climate change is happening, (b) the belief that climate change is human-caused and (c) how much people worry about climate change. Kahan [ 2017 ] is much concerned with the effect on support for public action, but we note that support for action is not a mediator in the model and hence there is no requirement that the treatment directly influences this variable. More importantly, Kahan [ 2017 ] maintains that in order for the effect of (consensus treatment) on (personal beliefs) to be mediated by (perceived consensus), there must be a direct effect of X (consensus message) on Y (personal beliefs). He claims; “there must be an overall treatment effect on the mediator” [p. 9]. In our view, Kahan [ 2017 ] then conducts several inappropriate statistical analyses looking for significant direct effects, and, finding none, concludes that the model must be misspecified.
We note that Kahan’s [ 2017 ] conclusions are incorrect. First, the process of mediation does not require a direct effect of X on Y. Kahan [ 2017 , p. 11] seems to rely on the “old” Baron and Kenny [ 1986 ] criteria, which have been shown to be wrong [Hayes, 2009 ]. One reason for this is that the total effect in a SEM model is the sum of many different paths of influence. Another reason is lower power to detect direct effects on more distal variables in the chain [e.g. see Hayes, 2009 ; Rucker et al., 2011 ]. In social psychological research, detecting an indirect effect in the absence of a total effect can happen as frequently as 50% of the time [Rucker et al., 2011 ]. Since a direct effect is not a necessary condition, imposing the requirement that a direct effect be established prior to mediation is both unjustified and can hamper theory development and testing [Rucker et al., 2011 ; Shrout and Bolger, 2002 ]. Moreover, because the approach we took in our paper was confirmatory and theory-driven, Kahan’s [ 2017 ] objection to our estimation approach could be dismissed purely on theoretical grounds.
2.2 Main experimental effects, non-parametric alternatives, and direct replications
However, even though it is not a statistical requirement, we nonetheless evaluate the direct effects here and show that Kahan [ 2017 ] is still incorrect in asserting that there were no significant main effects of the treatment (consensus message) on any of the mediating variables (climate beliefs). We start by offering a simple and straightforward test of the mean pre-post (within-subject) differences between the treatment and control groups for each of the included measures (Figure 1 ). The results demonstrate that participants exposed to the consensus treatment (vs. control) significantly changed their beliefs in the hypothesized direction. In short, out of the 5 variables, 4 are mediators, and the consensus treatment did in fact influence at least 3 of them directly; the remaining effects also trend in the expected direction. Note that a direct effect can still be mediated, even if the effect is not significant at the “ ” level. The scientific consensus statement specifically targets perceived consensus and beliefs about human-causation, which is clearly reflected in the significant main effects presented in Figure 1 .
We should note that one-tailed -values for the belief that climate change is happening ( ) and support for action ( ) are marginally significant. We present two-tailed -values here to be conservative, although our hypotheses were clearly directional. Kahan [ 2017 ] argues that one-tailed -values are inappropriate here because some respondents updated in the opposite direction [p. 7]. Although this sounds intuitive, this view is incorrect because the statistic that is being compared in null hypothesis significance testing (NHST) is the mean, and the mean-change is positive and directional. Thus, one-tailed -values are appropriate for directional hypotheses [Jones, 1952 ; Cho and Abe, 2013 ]. Kahan [ 2017 ] then changes his criticism and claims that because the distribution of the variables is skewed, mean comparisons are not informative. We are happy to entertain this criticism, but we do not understand Kahan’s [ 2017 ] subsequent decision to focus on the mode , as in our data, the modal change-score (e.g., “0”) only represents the responses of a minority of the sample. In our view, the more appropriate statistical test is to examine the median using a non-parametric approach.
Moreover, it is relatively unclear in which sections of the analysis Kahan [ 2017 ] is using the between-group comparisons versus the within-group comparisons. Our study uses a mixed factorial experimental design. A mixed design combines both within-subject (pre-post) as well as between-subject (treatment vs. control) measures. A unique feature of this design is that it allows for a “ difference-in-difference ” estimator (i.e., the pre-post change scores for each subject between the treatment and control groups). The analyses used in our study utilize the most statistically powerful combination: the pre-post difference scores between the treatment and control groups, not just “post-only” comparisons. In other words, Kahan’s [ 2017 ] argument that he was unable to find significant differences between the treatment and control groups on the mediator and outcome variables using post-only scores is irrelevant, as the main results do show significant mean and median differences when the change scores are properly compared.
Our results are further confirmed when subjected to non-parametric testing procedures. For example, the Mann-Whitney “equality of medians” test groups the number of responses that either fall below or rise above the median for both the treatment and control groups. This test directly evaluates whether or not the medians of the distributions are the same when comparing the pre-post change scores between groups. For the belief that climate change is human-caused, the medians are significantly different (50% of the responses are above the median value in the treatment condition whereas only 35% are above in the control group), (9.46), , indicating that more people in the treatment group shifted toward the belief that climate change is human caused. The same is true for worry about climate change (49% of responses are above the median in the treatment group vs. only 32% in the control), (11.41), . The same pattern even holds for support for public action (40% vs. 21%), (15.30), . The only exception is the belief that climate change is happening (45% of responses are above the median, whereas 39% in the control group), (1.71), .
To avoid further (and obscure) discussions about the mean, median and mode, it is more efficient to simply test whether or not the entire distribution of the change scores is the same in the treatment and control groups. Kahan [ 2017 ] compares the post-test scores between treatment and control, not considering the pre-test scores. However, the more powerful way to evaluate this is to examine the distribution of the pre-post change scores in each group and test whether or not the underlying distributions are statistically equal. The nonparametric Kolmogorov-Smirnov test clearly shows that for the belief that climate change is human-caused ( , ), worry ( , ) and public action ( , ), the empirical distributions of the observed change scores are not equal between the treatment and control groups. Consistent with the mean and medians tests, the distribution of the change scores is higher in the treatment group for all items except for the belief that climate change is happening ( , ).
Lastly, on March 10, 2016, before Kahan’s [ 2017 ] criticism was published, we posted a link to a large direct replication of our research in the comment section of PloS ONE [van der Linden, Leiserowitz and Maibach, 2016 ] to help resolve the unbalanced sample issue in the data. The replication was based on a high-powered large nationally representative experiment ( , treatment and control). Results of that replication show unequivocally clear direct effects of the consensus message on all key mediator and outcome variables (Table 1 ).
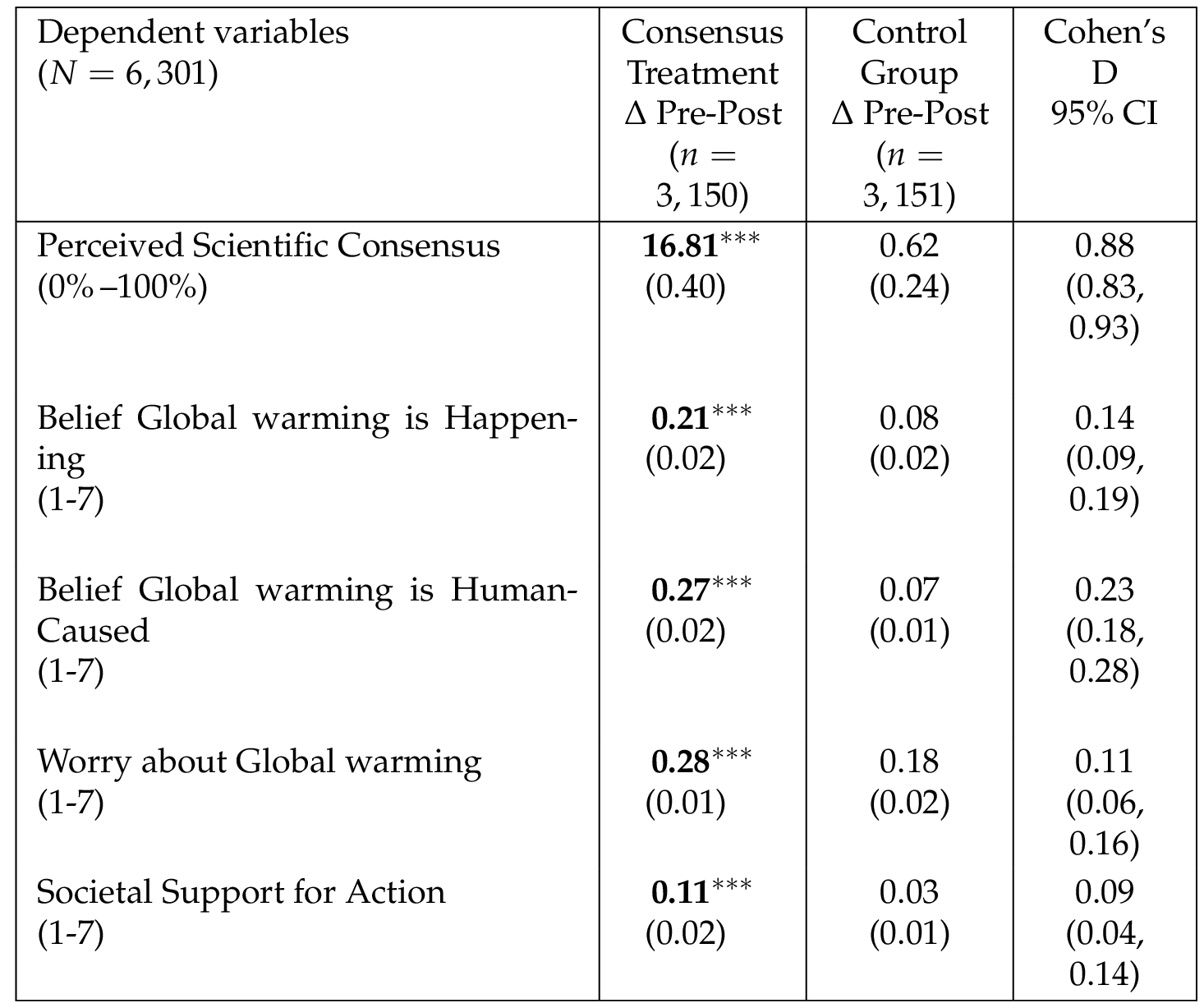
Note : Standard errors in parentheses. All mean comparisons significant at <0.001 (bold face). Unequal variances ( -test). Cohen’s is a standardized measure of effect size. Values between 0.3 and 0.6 are generally considered to be “moderate” effect-sizes in behavioral science research [Cohen, 1988 ].
Kahan [ 2017 ] claimed that our initial results were not significant. After our replication, detailing robust and significant results, Kahan then claims that our replication sample is too “large”. We simply encourage the reader to evaluate the effect-sizes in addition to statistical significance. In sum, we argue that Kahan [ 2017 ] incorrectly concluded that the consensus message did not directly impact the mediators. Moreover, as mentioned, a direct effect of the treatment on the key belief variables is not a necessary condition for mediation to occur. Lastly, Kahan [ 2017 ] misunderstands a central hypothesis of the Gateway Belief Model: any direct effect of the consensus message on the key beliefs is expected to be fully mediated by perceived scientific consensus.
2.3 Sequential mediation and the Structural Equation Model (SEM)
Kahan’s [ 2017 ] other major argument suggests that only “perceived scientific consensus” appears to be conditional on experimental treatment assignment (consensus vs. control) in the structural equation model, and that as a result, the model is “misspecified” and correlational in nature. This claim is false. Although the depiction of the GBM only visualizes a direct path from experimental assignment to perceived scientific consensus, all variables were estimated conditional on experimental assignment in the model evaluation stage. Indeed, there is a simple way to make all of the mediators conditional on treatment assignment by drawing direct paths/arrows from the independent variable (treatment vs. control) to all of the mediators in the model, as was done for perceived scientific consensus.
In fact, when the model was initially constructed, there were indeed paths going directly from the independent variable (treatment vs. control) to all other variables in the model. That analysis found that when controlling for perceived scientific consensus, the direct effect of the consensus treatment on all other variables disappears (i.e., the paths become non-significant). In other words, we wouldn’t have been able to determine that perceived consensus acts as a “gateway” (note: full mediation) without drawing those paths in the first place. Leaving those paths in the final model, i.e. including 4 extra non-significant paths makes the model unnecessarily more complex without improving model fit (i.e., the added complexity actually penalizes model fit). More importantly, these paths do not change any of the coefficients nor do they add any theoretical insight, as the model finds that the effect of the consensus message on the key beliefs is fully mediated by perceived consensus. Thus, Kahan’s [ 2017 ] claim that “there are no arrows from the experimental condition to the other variables” [p. 9] demonstrates his confusion about the nature of mediation and how SEM models are constructed and tested.
A second and related misunderstanding is Kahan’s [ 2017 ] claim that the mediational model is essentially “correlational” in nature [p. 8]. This is also incorrect. As explained before, this paper focuses on pre-post change scores. All of the variables in the GBM were modeled as experimentally-conditioned observed changes, i.e., every variable in the model was entered to reflect the pre-post difference scores . This includes the primary variable of interest — perceived scientific consensus (PSC) — but also all other variables. In other words, we estimated a sequential mediational process where a pre-post change in perceived scientific consensus (PSC) predicts a pre-post change in each of the key beliefs about climate change, which in turn, predicts a change in support for public action. The effect of the consensus message on the key beliefs is fully mediated by PSC (step 1), and the subsequent effect on public action is fully mediated by the key beliefs (step 2). The “cascading” effects on beliefs and support for public action are relatively small in comparison to the main effect precisely because the model estimates experimental effects. Crucially, contrary to what Kahan [ 2017 ] suggests, there is a significant indirect effect flowing from differential exposure to the treatment (vs. control) to public action via the multiple mediators ( , SE , ). 4
If we had estimated the model in a purely correlational fashion, most of the path coefficients would have been larger by a factor of 2 to 3. Similarly, if we had estimated the model on only the “treated” portion of the sample the results would have been much stronger. If anything, the fact that we estimated the model on the full sample (including pre-post controls) actually makes the model more conservative in its estimates. In short, contrary to Kahan’s [ 2017 ] claim that we overstate our findings, our findings are actually conservative estimates of the treatment effect.
2.4 On alternative models, a-theoretical simulations, and the affect-heuristic
In the supplementary information, Kahan [ 2017 ] argues that “many possible models” can be estimated on a given data set. We agree, but as we explained earlier, the purpose of our study was confirmatory, not an exploratory fishing expedition. Our mediation analysis is also based on experimental rather than correlational data. Thus, this study not only confirms prior theory, but also fits the model on causal data obtained from a randomized experiment (with additional covariate controls), which substantially reduces the likelihood of misspecification and provides a much stronger case for mediation than models based on correlational data [Hayes, 2013 ; Stone-Romero and Rosopa, 2008 ]. Thus, sweeping claims of SEM “misspecification” without providing meaningful theoretical alternatives is not constructive, as such criticisms are essentially baseless [Hayes, 2013 ; Lewandowsky, Gignac and Oberauer, 2015 ].
Moreover, the “many possible models” argument is specious. The best way to address the possibility of “other” potential model specifications is to let the model choices be guided by theoretically meaningful relationships [MacCallum et al., 1993 ]. This is exactly why our study tested the Gateway Belief Model (GBM), as several prior studies had independently identified and validated the core structure of the model [e.g., see Ding et al., 2011 ; McCright, Dunlap and Xiao, 2013 ]. The GBM has also been tested across different contexts, including vaccines [van der Linden, Clarke and Maibach, 2015 ], climate change [Bolsen and Druckman, 2017 ] and GMO’s [Dixon, 2016 ]. In fact, a recent meta-analysis found that perceived scientific consensus is a key determinant of public opinion on climate change [Hornsey et al., 2016 ]. In short, our model specification is based on a cumulative theory-informed social science evidence base.
Although we are open to alternative suggestions, we are baffled by the alternative “affect-heuristic” model advanced by Kahan [ 2017 ], in part because it seems to misrepresent the literature, and the nature of the affect-heuristic. At its most generic level, the GBM offers a dual-processing account of judgment formation [Chaiken and Trope, 1999 ; Evans, 2008 ; Marx et al., 2007 ; van der Linden, 2014 ] in the sense that the model combines both cognitive (belief-based) and affective (worry) determinants of public attitudes toward societal issues. To the extent that the left-hand side of the model represents input in the form of a consensus cue, the GBM is also consistent with the literature on heuristic information processing [Chaiken, 1980 ]. In the absence of a strong motivation to elaborate, people tend to heuristically process consensus cues because “consensus implies correctness” [Mutz, 1998 ; Panagopoulos and Harrison, 2016 ]. For example, when the scientific consensus is explicitly politicized (motivating elaboration), its persuasiveness is significantly reduced [Bolsen and Druckman, 2015 ; van der Linden et al., 2017b ]. However, we recognize that heuristics, such as “experts can be trusted” can be used in both a reflective and intuitive manner [Todorov, Chaiken and Henderson, 2002 ].
Kahan’s [ 2017 ] “affect” model, however, suggests that both cognition (belief-based) and emotional reactions (worry) all load onto the same underlying “affect” factor. This is not only circular and severely underspecified in the sense that one type of emotion (worry) is predicting another type (affect) but it also presupposes that people’s cognitive understanding of the issue is expressed as an affective disposition. The affect-heuristic describes a fast, associative, and automatic reaction that guides information processing and judgment [Zajonc, 1980 ; Slovic et al., 2007 ]. We find it unlikely that people’s cognitive understanding of climate change is expressed in the same manner as their affective reactions, as this clearly contradicts a wealth of literature distinguishing “risk as analysis” from “risk as feelings” [Marx et al., 2007 ; Slovic et al., 2004 ]. In short, we find Kahan’s [ 2017 ] alternative model poorly specified and unconvincing.
2.5 Practical significance and internal validity
“ Individual opinions influence political outcomes through aggregation. Even a modest amount of variation in opinion across individuals will profoundly influence collective deliberations ” — Kahan and Braman [ 2003 , p. 1406].
When the cultural cognition thesis was criticized for explaining only 1.6% of the variation in gun control attitudes [Fremling and Lott, 2003 ], Kahan and Braman [ 2003 ] responded with a baseball metaphor to explain how in the real world a tiny difference in batting averages can make a world of difference. In other words, “ it’s not the size of the R that matters, it’s what you do with it ” [Kahan and Braman, 2003 , p. 1406]. We largely agree with the sentiment expressed by Kahan in response to criticisms about his work, and therefore find it puzzling that Kahan [ 2017 ] uses his critic’s argument to question the practical relevance of our findings.
From a research design perspective, the experimental treatment was designed to directly influence respondents’ perception of the scientific consensus — it was not designed to directly influence support for action. In other words, the research design achieved proper measurement correspondence between the experimental manipulation ( consensus ) and the primary dependent measure ( perceived consensus ). Accordingly, the personal beliefs and support for action variables are appropriately modeled as secondary and tertiary in the causal chain of events and we would entirely expect the effect-sizes to decrease as a function of the how distal the variable is conceptually from the consensus message (Table 1 ).
Kahan [ 2017 ] views this pattern of findings as possible evidence of an “anchoring” effect, where providing subjects with any high number (97%) could potentially lead to higher post-test scores. This possibility has been empirically ruled out by Dixon [ 2016 ], who found that an unrelated high-number anchor (i.e. “90”) had no effect on subsequent judgments about the scientific consensus, whereas, in contrast, specific information about the scientific consensus did have a significant effect. We therefore find this “internal validity” criticism unconvincing. 5
We conducted a -curve analysis [Simonsohn, Nelson and Simmons, 2014 ] of all statistical tests we have conducted in our four scientific consensus papers to date, main effects, as well as interactions, the result of which is displayed in Figure 2 . The curve is significantly right-skewed, with 92% of our effects having a -value lower than <0.01. In fact, the actual -value for nearly all observed effects is indicating that the probability of observing the reported effects (or higher) — given that there really is no effect — is extremely low (0.001%). The power and truth-value of the scientific consensus effects are therefore extremely high.
Ultimately, whether or not the effects reported in the paper are “practically useful” is for the reader to decide. The average effect of the perceived scientific consensus message (~13% change, Cohen’s ) is considered large by most effect-size standards in social and behavioral science [Cohen, 1988 ]. To illustrate the robustness of this finding, we present a Bayesian analysis of the main effect on perceived consensus in Figure 3 . The posterior distribution of the effect-size is clearly non-overlapping with the prior (null). The second panel shows a sequential analysis of the Bayes Factor as a function of sample size, resulting in extreme evidence in favor of the alternative hypothesis (360,000 times more likely than the null). 6
We further note that it is not surprising that the subsequent (indirect) changes in personal beliefs, worry, and support for public action are much smaller, because (a) the treatment did not target these beliefs directly and (b) these changes are observed in response to an extremely minimal treatment (i.e., a single exposure to a brief statement). Under these conditions, even small effects can be considered impressive [Prentice and Miller, 1992 ]. To return to the quote that started this section, small effects can add up to produce meaningful changes. If these effect-sizes are observed after a single exposure to a minimal message, we take this as reason to be optimistic about the potential of consensus messaging, and we encourage scholars and colleagues to continue to conduct research on the Gateway Belief Model (GBM).
3 Conclusion
In this response we have demonstrated that Kahan’s [ 2017 ] claims about the study’s main effects are incorrect and largely irrelevant to the validity of the mediation analysis. In addition, we maintain that his misunderstanding of the structural equation model has led to inaccurate conclusions about the study’s main findings, and his analysis of the modal scores only confuses and distracts from a straightforward (non-parametric) analysis of the pre-post difference scores between groups. In short, Kahan’s [ 2017 ] comments mostly rely upon straw man arguments and do not advance any substantially new insights about the data. They also fail to meaningfully engage with social science theory, and in our opinion, use inappropriate statistical methods.
However, we thank Kahan for giving us the opportunity to highlight that: (a) although relatively few social science studies estimate mediational models on experimental data [Stone-Romero and Rosopa, 2008 ], our study does; (b) even though direct effects are not a necessary condition for mediation (and that in practice, experiments often fail to influence a posited mediator), the treatment in this study did influence the posited mediators; and lastly, (c) our mediation model is theoretically well-specified and constructively builds upon and confirms the findings of prior literature. In short, we remain unconvinced by Kahan’s [ 2017 ] objections and stand by our conclusion that our paper indeed provides the strongest evidence to date that perceived scientific consensus acts as a “gateway” to other key beliefs about climate change.
References
-
Aklin, M. and Urpelainen, J. (2014). ‘Perceptions of scientific dissent undermine public support for environmental policy’. Environmental Science & Policy 38, pp. 173–177. https://doi.org/10.1016/j.envsci.2013.10.006 .
-
Ballarini, C. and Sloman, S. (2017). ‘Reasons and the “Motivated Numeracy Effect”’. In: Proceedings of the 39th Annual Meeting of the Cognitive Science Society , pp. 1580–1585. URL: https://mindmodeling.org/cogsci2017/papers/0309/index.html .
-
Baron, R. M. and Kenny, D. A. (1986). ‘The moderator-mediator variable distinction in social psychological research: Conceptual, strategic, and statistical considerations’. Journal of Personality and Social Psychology 51 (6), pp. 1173–1182. https://doi.org/10.1037/0022-3514.51.6.1173 .
-
Bedford, D. (2015). ‘Does Climate Literacy Matter? A Case Study of U.S. Students’ Level of Concern about Anthropogenic Global Warming’. Journal of Geography 115 (5), pp. 187–197. https://doi.org/10.1080/00221341.2015.1105851 .
-
Bolsen, T. and Druckman, J. (2017). ‘Do partisanship and politicization undermine the impact of scientific consensus on climate change beliefs?’ NIPR Working Paper Series , pp. 16–21.
-
Bolsen, T. and Druckman, J. N. (2015). ‘Counteracting the Politicization of Science’. Journal of Communication 65 (5), pp. 745–769. https://doi.org/10.1111/jcom.12171 .
-
Brewer, P. R. and McKnight, J. (2017). ‘“A Statistically Representative Climate Change Debate”: Satirical Television News, Scientific Consensus, and Public Perceptions of Global Warming’. Atlantic Journal of Communication 25 (3), pp. 166–180. https://doi.org/10.1080/15456870.2017.1324453 .
-
Chaiken, S. and Trope, Y. (1999). Dual-process theories in social psychology. U.S.A.: Guilford Press.
-
Chaiken, S. (1980). ‘Heuristic versus systematic information processing and the use of source versus message cues in persuasion.’ Journal of Personality and Social Psychology 39 (5), pp. 752–766. https://doi.org/10.1037/0022-3514.39.5.752 .
-
Cho, H.-C. and Abe, S. (2013). ‘Is two-tailed testing for directional research hypotheses tests legitimate?’ Journal of Business Research 66 (9), pp. 1261–1266. https://doi.org/10.1016/j.jbusres.2012.02.023 .
-
Cohen, D. (1988). Statistical power analysis for the behavioral sciences. Hillsdale, NJ, U.S.A.: Erlbaum.
-
Cook, J. and Lewandowsky, S. (2016). ‘Rational Irrationality: Modeling Climate Change Belief Polarization Using Bayesian Networks’. Topics in Cognitive Science 8 (1), pp. 160–179. https://doi.org/10.1111/tops.12186 . PMID: 26749179 .
-
Cook, J., Lewandowsky, S. and Ecker, U. K. H. (2017). ‘Neutralizing misinformation through inoculation: Exposing misleading argumentation techniques reduces their influence’. PLOS ONE 12 (5), e0175799. https://doi.org/10.1371/journal.pone.0175799 .
-
Deryugina, T. and Shurchkov, O. (2016). ‘The Effect of Information Provision on Public Consensus about Climate Change’. PLOS ONE 11 (4), e0151469. https://doi.org/10.1371/journal.pone.0151469 .
-
Ding, D., Maibach, E. W., Zhao, X., Roser-Renouf, C. and Leiserowitz, A. (2011). ‘Support for climate policy and societal action are linked to perceptions about scientific agreement’. Nature Climate Change 1 (9), pp. 462–466. https://doi.org/10.1038/nclimate1295 .
-
Dixon, G. (2016). ‘Applying the Gateway Belief Model to Genetically Modified Food Perceptions: New Insights and Additional Questions’. Journal of Communication 66 (6), pp. 888–908. https://doi.org/10.1111/jcom.12260 .
-
Dixon, G., Hmielowski, J. and Ma, Y. (2017). ‘Improving Climate Change Acceptance Among U.S. Conservatives Through Value-Based Message Targeting’. Science Communication 39 (4), pp. 520–534. https://doi.org/10.1177/1075547017715473 .
-
Evans, J. S. B. T. (2008). ‘Dual-Processing Accounts of Reasoning, Judgment, and Social Cognition’. Annual Review of Psychology 59 (1), pp. 255–278. https://doi.org/10.1146/annurev.psych.59.103006.093629 .
-
Flynn, D., Nyhan, B. and Reifler, J. (2017). ‘The Nature and Origins of Misperceptions: Understanding False and Unsupported Beliefs About Politics’. Political Psychology 38, pp. 127–150. https://doi.org/10.1111/pops.12394 .
-
Fremling, G. M. and Lott, J. R. (2003). ‘The Surprising Finding That “Cultural Worldviews” Don’t Explain People’s Views on Gun Control’. University of Pennsylvania Law Review 151 (4), pp. 1341–1348. https://doi.org/10.2307/3312932 .
-
Gelman, A. and Rubin, D. B. (1995). ‘Avoiding Model Selection in Bayesian Social Research’. Sociological Methodology 25, p. 165. https://doi.org/10.2307/271064 .
-
Guess, A. and Coppock, A. (2016). ‘The exception, not the rule? The rarely polarizing effect of challenging information’. Working Paper, Yale University. URL: https://alexandercoppock.files.wordpress.com/2014/11/backlash_apsa.pdf .
-
Hamilton, L. C. (2016). ‘Public Awareness of the Scientific Consensus on Climate’. SAGE Open 6 (4), p. 215824401667629. https://doi.org/10.1177/2158244016676296 .
-
Hayes, A. F. (2009). ‘Beyond Baron and Kenny: Statistical Mediation Analysis in the New Millennium’. Communication Monographs 76 (4), pp. 408–420. https://doi.org/10.1080/03637750903310360 .
-
— (2013). Introduction to Mediation, Moderation, and Conditional Process Analysis. New York, NY, U.S.A.: The Guilford Press.
-
Hill, S. J. (2017). ‘Learning Together Slowly: Bayesian Learning about Political Facts’. The Journal of Politics 79 (4), pp. 1403–1418. https://doi.org/10.1086/692739 .
-
Hornsey, M. J., Harris, E. A., Bain, P. G. and Fielding, K. S. (2016). ‘Meta-analyses of the determinants and outcomes of belief in climate change’. Nature Climate Change 6 (6), pp. 622–626. https://doi.org/10.1038/nclimate2943 .
-
Johnson, D. R. (2017). ‘Bridging the political divide: Highlighting explanatory power mitigates biased evaluation of climate arguments’. Journal of Environmental Psychology 51, pp. 248–255. https://doi.org/10.1016/j.jenvp.2017.04.008 .
-
Jones, L. V. (1952). ‘Test of hypotheses: one-sided vs. two-sided alternatives.’ Psychological Bulletin 49 (1), pp. 43–46. https://doi.org/10.1037/h0056832 .
-
Kahan, D. M. (2017). ‘The “Gateway Belief” illusion: reanalyzing the results of a scientific-consensus messaging study’. JCOM 16 (5), A03. URL: https://jcom.sissa.it/archive/16/05/JCOM_1605_2017_A03 .
-
Kahan, D. M. and Braman, D. (2003). ‘Caught in the Crossfire: A Defense of the Cultural Theory of Gun-Risk Perceptions’. University of Pennsylvania Law Review 151 (4), p. 1395. https://doi.org/10.2307/3312936 .
-
Kahan, D. M., Jenkins-Smith, H. and Braman, D. (2011). ‘Cultural cognition of scientific consensus’. Journal of Risk Research 14 (2), pp. 147–174. https://doi.org/10.1080/13669877.2010.511246 .
-
Koehler, D. J. (2016). ‘Can journalistic “false balance” distort public perception of consensus in expert opinion?’ Journal of Experimental Psychology: Applied 22 (1), pp. 24–38. https://doi.org/10.1037/xap0000073 .
-
Krosnick, J. and MacInnis, B. (2015). ‘Fox and not-Fox television news impact on opinions on global warming: Selective exposure, not motivated reasoning’. In: Social Psychology and Politics. Ed. by J. P. Forgas, K. Fiedler and W. D. Crano. NY, U.S.A.: Psychology Press.
-
Kuhn, D. and Lao, J. (1996). ‘Effects of Evidence on Attitudes: Is Polarization the Norm?’ Psychological Science 7 (2), pp. 115–120. https://doi.org/10.1111/j.1467-9280.1996.tb00340.x .
-
Leiserowitz, A., Maibach, E., Roser-Renouf, C., Rosenthal, S. and Cutler, M. (2017). Climate change in the American mind: May, 2017. Yale University and George Mason University. New Haven, CT, U.S.A.: Yale Project on Climate Change Communication.
-
Lewandowsky, S., Gignac, G. E. and Oberauer, K. (2015). ‘The Robust Relationship Between Conspiracism and Denial of (Climate) Science’. Psychological Science 26 (5), pp. 667–670. https://doi.org/10.1177/0956797614568432 .
-
Lewandowsky, S., Gignac, G. E. and Vaughan, S. (2013). ‘The pivotal role of perceived scientific consensus in acceptance of science’. Nature Climate Change 3 (4), pp. 399–404. https://doi.org/10.1038/nclimate1720 .
-
MacCallum, R. C., Wegener, D. T., Uchino, B. N. and Fabrigar, L. R. (1993). ‘The problem of equivalent models in applications of covariance structure analysis.’ Psychological Bulletin 114 (1), pp. 185–199. https://doi.org/10.1037/0033-2909.114.1.185 .
-
Marsman, M. and Wagenmakers, E.-J. (2017). ‘Bayesian benefits with JASP’. European Journal of Developmental Psychology 14 (5), pp. 545–555. https://doi.org/10.1080/17405629.2016.1259614 .
-
Marx, S. M., Weber, E. U., Orlove, B. S., Leiserowitz, A., Krantz, D. H., Roncoli, C. and Phillips, J. (2007). ‘Communication and mental processes: Experiential and analytic processing of uncertain climate information’. Global Environmental Change 17 (1), pp. 47–58. https://doi.org/10.1016/j.gloenvcha.2006.10.004 .
-
McCright, A. M., Dunlap, R. E. and Xiao, C. (2013). ‘Perceived scientific agreement and support for government action on climate change in the USA’. Climatic Change 119 (2), pp. 511–518. https://doi.org/10.1007/s10584-013-0704-9 .
-
Mutz, D. (1998). Impersonal Influence: How Perceptions of Mass Collectives Affect Political Attitudes. Cambridge, U.K.: Cambridge University Press.
-
Myers, T. A., Maibach, E., Peters, E. and Leiserowitz, A. (2015). ‘Simple Messages Help Set the Record Straight about Scientific Agreement on Human-Caused Climate Change: The Results of Two Experiments’. PLOS ONE 10 (3). Ed. by K. Eriksson, e0120985. https://doi.org/10.1371/journal.pone.0120985 .
-
National Academies of Sciences (2017). Communicating Science Effectively: A Research Agenda. Washington, DC, U.S.A.: The National Academies Press.
-
Oreskes, N. and Conway, E. (2010). Merchants of doubt. New York, NY, U.S.A.: Bloomsbury Press.
-
Panagopoulos, C. and Harrison, B. (2016). ‘Consensus cues, issue salience and policy preferences: An experimental investigation’. North American Journal of Psychology 18 (2), pp. 405–417.
-
Plutzer, E., McCaffrey, M., Hannah, A. L., Rosenau, J., Berbeco, M. and Reid, A. H. (2016). ‘Climate confusion among U.S. teachers’. Science 351 (6274), pp. 664–665. https://doi.org/10.1126/science.aab3907 .
-
Prentice, D. A. and Miller, D. T. (1992). ‘When small effects are impressive.’ Psychological Bulletin 112 (1), pp. 160–164. https://doi.org/10.1037/0033-2909.112.1.160 .
-
Ranney, M. A. and Clark, D. (2016). ‘Climate Change Conceptual Change: Scientific Information Can Transform Attitudes’. Topics in Cognitive Science 8 (1), pp. 49–75. https://doi.org/10.1111/tops.12187 .
-
Rucker, D. D., Preacher, K. J., Tormala, Z. L. and Petty, R. E. (2011). ‘Mediation Analysis in Social Psychology: Current Practices and New Recommendations’. Social and Personality Psychology Compass 5 (6), pp. 359–371. https://doi.org/10.1111/j.1751-9004.2011.00355.x .
-
Shrout, P. E. and Bolger, N. (2002). ‘Mediation in experimental and nonexperimental studies: New procedures and recommendations.’ Psychological Methods 7 (4), pp. 422–445. https://doi.org/10.1037/1082-989x.7.4.422 .
-
Simonsohn, U., Nelson, L. D. and Simmons, J. P. (2014). ‘P-curve: A key to the file-drawer.’ Journal of Experimental Psychology: General 143 (2), pp. 534–547. https://doi.org/10.1037/a0033242 .
-
Slovic, P., Finucane, M. L., Peters, E. and MacGregor, D. G. (2004). ‘Risk as Analysis and Risk as Feelings: Some Thoughts about Affect, Reason, Risk, and Rationality’. Risk Analysis 24 (2), pp. 311–322. https://doi.org/10.1111/j.0272-4332.2004.00433.x .
-
— (2007). ‘The affect heuristic’. European Journal of Operational Research 177 (3), pp. 1333–1352. https://doi.org/10.1016/j.ejor.2005.04.006 .
-
Stone-Romero, E. F. and Rosopa, P. J. (2008). ‘The Relative Validity of Inferences About Mediation as a Function of Research Design Characteristics’. Organizational Research Methods 11 (2), pp. 326–352. https://doi.org/10.1177/1094428107300342 .
-
Todorov, A., Chaiken, S. and Henderson, M. D. (2002). The heuristic-systematic model of social information processing. Ed. by J. P. Dillard and M. Pfa. Thousand Oaks, CA, U.S.A.: Sage, pp. 195–211.
-
van der Linden, S. L. (2014). ‘On the relationship between personal experience, affect and risk perception:The case of climate change’. European Journal of Social Psychology 44 (5), pp. 430–440. https://doi.org/10.1002/ejsp.2008 .
-
— (2015). ‘A Conceptual Critique of the Cultural Cognition Thesis’. Science Communication 38 (1), pp. 128–138. https://doi.org/10.1177/1075547015614970 .
-
van der Linden, S. L. and Chryst, B. (2017). ‘No Need for Bayes Factors: A Fully Bayesian Evidence Synthesis’. Frontiers in Applied Mathematics and Statistics 3. https://doi.org/10.3389/fams.2017.00012 .
-
van der Linden, S. L., Clarke, C. E. and Maibach, E. W. (2015). ‘Highlighting consensus among medical scientists increases public support for vaccines: evidence from a randomized experiment’. BMC Public Health 15 (1), p. 1207. https://doi.org/10.1186/s12889-015-2541-4 .
-
van der Linden, S. L., Leiserowitz, A. and Maibach, E. (2017). ‘Scientific agreement can neutralize politicization of facts’. Nature Human Behaviour . https://doi.org/10.1038/s41562-017-0259-2 .
-
van der Linden, S. L., Leiserowitz, A. and Maibach, E. W. (2016). ‘Response to Kahan’. Figshare . https://doi.org/10.6084/m9.figshare.3486716 .
-
van der Linden, S. L., Leiserowitz, A. A., Feinberg, G. D. and Maibach, E. W. (2014). ‘How to communicate the scientific consensus on climate change: plain facts, pie charts or metaphors?’ Climatic Change 126 (1–2), pp. 255–262. https://doi.org/10.1007/s10584-014-1190-4 .
-
— (2015). ‘The Scientific Consensus on Climate Change as a Gateway Belief: Experimental Evidence’. PLOS ONE 10 (2), e0118489. https://doi.org/10.1371/journal.pone.0118489 .
-
van der Linden, S. L., Maibach, E., Cook, J., Leiserowitz, A., Ranney, M., Lewandowsky, S., Árvai, J. and Weber, E. U. (2017a). ‘Culture versus cognition is a false dilemma’. Nature Climate Change 7 (7), p. 457. https://doi.org/10.1038/nclimate3323 .
-
van der Linden, S. L., Leiserowitz, A., Rosenthal, S. and Maibach, E. (2017b). ‘Inoculating the Public against Misinformation about Climate Change’. Global Challenges 1 (2), p. 1600008. https://doi.org/10.1002/gch2.201600008 .
-
Webb, B. S. and Hayhoe, D. (2017). ‘Assessing the Influence of an Educational Presentation on Climate Change Beliefs at an Evangelical Christian College’. Journal of Geoscience Education 65 (3), pp. 272–282. https://doi.org/10.5408/16-220.1 .
-
Wood, T. and Porter, E. (2016). The elusive backfire effect: Mass attitudes’ steadfast factual adherence . URL: https://ssrn.com/abstract=2819073 .
-
Zajonc, R. B. (1980). ‘Feeling and thinking: Preferences need no inferences.’ American Psychologist 35 (2), pp. 151–175. https://doi.org/10.1037/0003-066x.35.2.151 .
Authors
Sander van der Linden is a University Lecturer (Assistant Professor) in Social Psychology and Director of the Cambridge Social Decision-Making Laboratory at the University of Cambridge. He is also a Fellow in Psychological and Behavioural Sciences at Churchill College, Cambridge and the Royal Society of Arts (FRSA). He is an Associate Fellow (AFBPsS) of the British Psychological Society and a recipient of the 2017 Frank Prize for research in Public Interest Communications. He has also received awards from the American Psychological Association (APA), the International Association of Applied Psychology (IAAP) and the Society for the Psychological Study of Social Issues (SPSSI). He is affiliated with the Yale Program on Climate Change Communication. Prior to Cambridge, he held appointments at Princeton University, Yale University, and the London School of Economics (LSE). E-mail: sander.vanderlinden@psychol.cam.ac.uk .
Anthony Leiserowitz is Director of the Yale Program on Climate Change Communication at Yale University and a Senior Research Scientist at the Yale University School of Forestry & Environmental Studies. E-mail: anthony.leiserowitz@yale.edu .
Edward Maibach is a Distinguished University Professor in the Department of Communication at George Mason University and Director of Mason’s Center for Climate Change Communication (4C). E-mail: emaibach@gmu.edu .
Endnotes
1 We note that there is some debate about the validity of this replication.
2 Sequential means that the (indirect) effect of an independent variable on a dependent variable operates through a chain of mediator variables. SEM models often have multiple dependent and mediator variables.
3 The main effects of the consensus message (by experimental condition) are described in detail in van der Linden et al. [ 2014 ] on which the subsequent mediational analysis in van der Linden et al. [ 2015 ] is based.
4 Kahan uses Bayes Factors to evaluate evidence for our model. van der Linden and Chryst [ 2017 ] point out that Bayes Factors are inappropriate for evaluating strength of evidence. Kahan’s criticism of van der Linden & Chryst’s approach to Bayesian Evidence Synthesis [ 2017 ] has nothing to do with the fact that Bayes Factors are still logically incoherent, see also Gelman and Rubin [ 1995 ] for why model comparison using BIC is ill-advised.
5 Moreover, in our experiments, subjects are typically assigned to three randomized blocks of questions on different media topics so that it is unlikely that participants know we are interested in their opinions about climate change. We also give the appearance that the scientific consensus statement is selected randomly from a large database and introduce distractors after treatment exposure to introduce a delay between the pre and post-test measures.
6 van der Linden and Chryst [ 2017 ] recommend examining the posterior distribution rather than the Bayes Factor.