1 Introduction
1.1 Citizen science on urban mobility
Transport is a key contributor to urban air pollution and climate change and to reach net zero, transport emissions need to drop by 90%, indicating that mobility behaviours need to change [European Commission, 2020]. The public is largely aware of the need for change [Carmichael, 2019], yet less aware of the ways in which they can act, not only to change their behaviour, but also to influence policy, due to a perception that climate risk is distant and a lack of personal efficacy [Milfont, 2012]. Citizen science (CS) projects on these issues could help to address some of these gaps between citizens’ values and actions and the prioritisation of associated policies.
CS projects are often mutually beneficial: established to improve the scientific knowledge and skills of participants and to benefit researchers through the crowdsourcing of data [Cappa, Franco & Rosso, 2022]. Increasingly, CS projects are moving away from crowdsourcing data (top-down) to explicitly focusing on citizen empowerment and policy change (bottom-up). This change in how CS operates has been described as a shift from a ‘productivity view’ to a ‘democratisation view’ for sustainability transitions [Sauermann et al., 2020]. While there is progress in democratic CS, there remains a call for it to build citizens’ capacities, bridge communities with policy and decision-making processes and develop robust monitoring and evaluation plans to assess these social-political dimensions of CS, as to date most projects do not evaluate the citizens’ experience — or at least these findings are not publicly available [CitiMeasure, 2023].
1.2 WeCount — citizens observing urban transport
WeCount (2019–2021) was a Horizon 2020 CS project which sought to quantify local road transport, produce scientific knowledge in sustainable mobility and environmental pollution and co-design informed solutions for several transport challenges.
WeCount focused on six cities across five European case studies: Madrid and Barcelona (Spain), Leuven (Belgium), Ljubljana (Slovenia), Dublin (Ireland) and Cardiff (United Kingdom). WeCount followed participatory CS methods to co-create solutions to traffic issues, with data provided by innovative low cost, automated, road traffic counting sensors (called a Telraam1). Each participant mounted the free sensor to a window in their house that faced a road and they were allowed to keep the sensor once the project ended.
Citizens could keep track of traffic on their street and were able to analyse their local transport data using a digital platform. Citizens took part in several workshops and were able to work together to co-create solutions to local mobility issues. WeCount ran during the COVID-19 pandemic and original recruitment and engagement plans had to shift online [Sardo, Laggan, Franchois & Fogg-Rogers, 2022].
In WeCount, the project design, choice of sensor and the research framework were decided and created “top down”, without significant citizen involvement or input. However, several other aspects were co-created; for example, the online platform was co-created in Leuven with local participants. During the project, citizens were encouraged to make visual observations, discuss their data with other participants, and were able to suggest improvements in sensor design. Citizens were also involved in workshops and contributed to how sensor data were analysed.
This paper explores the suitability and value of CS for addressing sustainable mobility issues and reports on the evaluation findings of the citizens’ experience and engagement with a European CS project on urban mobility. It discusses key aspects involved in citizen participation in issues related to sustainable mobility, adding to the growing body of social science evidence on the value of CS for empowerment, behaviour change and collective political action.
2 Methods
2.1 Recruitment and overall demographics
Initially, the project team had planned a combination of in-person and online recruitment approaches. The online strategies involved creating awareness through social media campaigns and using established networks. Local teams planned on using intermediaries, such as advocacy groups, support organisations, or community workers, to organise face-to-face group meetings in locations like schools, community centers, care homes, etc. Neighbourhood and door-to-door campaigns, leaflet distribution, and activities/events at strategic community groups were also part of the recruitment plans.
However, as the project finalised its recruitment plans, the onset of the COVID-19 pandemic introduced numerous challenges. In-person recruitment methods became unfeasible due to travel restrictions, gathering limitations, and social-distancing measures. All recruitment activities shifted to an online format, heavily relying on social media campaigns and virtual interactions with existing stakeholders (e.g., schools, local councils, etc.).
WeCount adopted a focused and targeted recruitment strategy, employing social media and Zoom/Teams calls to reach specific volunteer groups, harnessing activists, leveraging existing contact networks, and gaining endorsement from local venues and institutions. In addition, WeCount citizens were encouraged to become dedicated “local champions” to promote project awareness and assist fellow citizens in their neighbourhoods.
Sensors were delivered by hand to all citizens, using a safe door-step drop-off, in line with the pandemic restrictions.
WeCount engaged with a total of 843 citizens and stakeholders through workshops, seminars, mutual learning and science-policy dialogue workshops (Table 1),
with some of the participants taking part in more than one engagement method (i.e. owned a sensor and took part in workshops). A total of 368 (43%) citizen scientists with sensors from WeCount case studies directly engaged with the project over its 24-month duration. Evaluation participants self-selected from the people taking part in all activities, resulting in (out of 368) people completing the final survey. This represents 64% of all WeCount members who were part of a case study network. Additionally, 37 citizens were interviewed. Each local team invited 6–8 citizens from their case study to be interviewed. The teams made an effort to recruit interviewees with diverse backgrounds, interests and roles in the project. Ten WeCount team members took part in interviews.
The interview qualitative data were triangulated with quantitative data from the survey and will be integrated together throughout the results section.
There was a nearly even split of male (51%; ) and female (49%; ) participants in the project. Thanks to efforts to work with schools, WeCount attracted a young sample with 29% () of participants younger than 16. Adult WeCount citizens were highly educated (82%; ; had a degree or above).
2.2 Online surveys
Sign-up form. WeCount participants consented to take part in the project using an online sign-up form. Eligibility was based on having a window facing the street with a minimum and maximum distance pre-defined for the sensor to generate reliable data.
End of project survey. Participation experience was evaluated using an online survey, which was designed to be relatively short, quick and easy to complete. Closed questions included Likert scale rating scales and multiple-choice options. Open-ended questions allowed participants to provide answers in their own terms [Grand & Sardo, 2017] but were kept to a minimum, since they tend to have a lower response rate [Groves et al., 2004]. Surveys were prepared in English, translated and distributed to participants in their local languages.
Qualitative data from open questions was given an initial review to identify and code themes [Braun & Clarke, 2006]. Data were imported into NVivo for a deeper analysis of content and themes.
Raw quantitative data was cleaned in Excel, with demographic data and project reach processed and analysed and closed questions coded. Relational statistics were performed to see how certain themes relate, if at all, to demographic characteristics and other themes and were considered significant at a 95% confidence interval (). For statistical analysis, all survey data were coded and then imported into SPSS. Data were explored initially to test for normal distribution. As data were not normally distributed, nonparametric tests (Mann-Whitney and Kruskal Wallis) were run for each question asked (a 95% confidence interval was used ()). Where relevant and possible, post-hoc testing was subsequently run to ascertain which groups were different from each other.
2.3 Interviews
Citizens took part in semi-structured interviews at the end of the project to further explore sustainable mobility and CS topics, alongside citizens’ experiences. Interviews were designed as semi-structured allowing participants to provide answers in their own terms [Groves et al., 2004]. The in-depth interviews occurred online in the local languages and were audio recorded, transcribed by professional transcribers and translated to English for analysis. Quotes from interviews with citizens have the following ID: CITY Citizen InterviewNUMBER.
Interviews were also used to explore the experience of the WeCount team, using a similar method. Staff members were asked to reflect on the project process, their experiences and the project’s impact on themselves and the community. Quotes from interviews with the team have the following ID: Staff InterviewNUMBER.
Each interview set — from citizens and from staff, was analysed in NVivo using the process of thematic analysis [Braun & Clarke, 2006], searching for themes that captured patterned meaning across the data. The codes were refined and accumulated into themes that represented the meaning across the dataset. Secondary analysis was performed with review by the evaluation team to ensure the themes adequately represented the original data.
Interviews with citizens were analysed using one coding frame, which resulted in six themes. Interviews with staff were inductively coded as well, resulting in seven themes which relate to the Impact Assessment Framework below.
2.4 Impact assessment framework
The WeCount team was aware of the need to look at both individual and broader sustainability factors within CS, as this is needed for more integrative approaches that look within and outside of projects and support the research community to systematically assess project quality. Therefore, each interviewed team member quantitatively assessed the perceived impact of the project across several dimensions, using an adapted version of the Impact Assessment Framework2 [Passani, Janssen, Hölscher & Di Lisio, 2022]. The framework aims to help standardise impact assessment of CS and considers five areas of impact: scientific, social, economic, political and environmental impact. A 1 to 5 Likert scale was used whereby 1 was not relevant (no perceived impact) and 5 was very relevant (crucial perceived impact area).
3 Results
3.1 Motivations for joining
The main motivations for taking part in WeCount (Figure 1) were as follows: ‘an interest in sustainable mobility’ (22%; ) and ‘to contribute to research’ (21%; ). There is a highly significant difference between gender and original motivation to join due to an interest in technology (Mann-Whitney ; ; two-tailed). Men were significantly more likely than women to join WeCount out of an interest in technology.
There was a significant difference between higher education attainment and more science-related motivations (Kruskal Wallis test), with highly educated people more likely to choose these motivations. These motivations were ‘to count traffic’ (; ) and ‘to contribute to research’ (; ). There is no significant difference between age and motivation.
Interview responses aligned with these motivations, with interviewees saying that they had chosen to take part due to the ability to collect and analyse data. Many described regularly checking the website to visualise patterns across the city, or to monitor travel at certain times of the day. As one participant stated:
I thought it was very interesting. When I first looked into [the] Barcelona map and learned where traffic measuring devices [were] I thought it was very interesting because that way we can characterise the area somehow. Moreover, having citizens involved make it very modern. (Barcelona Citizen Interview01)
Interviewees said that they wanted to take part because they wanted to gather objective evidence about the traffic on their street. Many told stories about discussing the traffic levels and speed (and directly related impacts such as noise and air pollution) with policymakers but being previously unable to prove it. Some described how they had reported this to authorities but had previously been dismissed as emotional or exaggerating or how the authorities had monitored the street but during quiet periods, so they could then dismiss the claims. The continuous stream of data from the sensor meant they felt they could no longer be dismissed. For example:
I think the situation is actually worse than we thought it was. It’s been eye-opening really. It’s actually busier than we thought it was because the data actually shows us that it’s busier. It’s really revealing and hopefully, it can be used for some kind of constructive change. That’s what we’re hoping. (Cardiff Citizen Interview07)
3.2 The citizen’s experience
64% () of WeCount members who owned a sensor took part in the final survey. Respondents’ expectations (Figure 2) were largely met, with 67% () saying they were met ‘extremely’ or ‘very’ well and only 5% () believing their expectations to be met unsatisfactorily. Overall, survey respondents had a positive experience, with 83% () rating their time as either excellent or good. 13% () had an ‘average’ time, while just 3% () had a ‘poor’ time on the project.
The interview themes reinforced the survey data, with most participants stating that they had enjoyed being part of the project. They felt that WeCount had operated smoothly, with good communication between case study staff and participants. Many participants described the data as an excellent legacy, for example:
I think it’s a wonderful project. I would love to see it maybe happen again and maybe greater outreach into other areas particularly. It was very well done, very user-friendly. The information is great, even if you weren’t going to use it… I’ll keep that Telraam going for months to come. (Dublin Citizen Interview07)
However, many participants (around 30%) did experience difficulties setting up the sensor and maintaining its operation over several weeks, making dealing with the technology a frustrating experience. The technology was the main reason as to why participants expressed negative experiences, as it was not always easy to use.
3.3 Learning about urban mobility
The survey indicated that 75% () of respondents saw at least some improvement in their knowledge (Figure 3), with 52% () seeing an extreme improvement. Kruskal Wallis testing found that neither age, gender, educational attainment or case study had a bearing on knowledge improvement.
For survey respondents, ‘being part of a research project’ was their favourite part of being involved (34%; ). Largely reflecting original motivations for joining, this was followed by a feeling that they were ‘making a difference’ (19%; ). Interestingly, the technology (18%; ) came third, even though it was ranked 6th for motivation to join, which suggests that value may have been added from using the Telraam sensor and associated tools and platforms during the project. Gathering evidence to support a campaign (15%; ) came fourth, which likely relates to respondents’ pre-existing interest in sustainable mobility.
There was no statistical difference between age or educational attainment and favourite aspect, however there was for gender. Kruskal Wallis testing found that working collectively to solve problems was a highly significant difference between genders (; ). Post-hoc Mann Whitney testing found that the mean score for this favourite aspect reported by men is on average points less than for the mean score reported by women. This mean difference is significant at the 0.05 level (Mann-Whitney , , two-tailed). In other words, women were statistically more likely than men to consider collective problem solving to be their favourite aspect of WeCount.
3.4 Sustainable mobility action and behaviour change
The interview and survey data indicated that many citizens joined WeCount to gather evidence to further their vision of safer communities, with the project fulfilling these aims. Citizens described many reasons for taking action, from cars speeding on their streets, to noise pollution, air pollution, and unsafe walking and cycling routes. They planned to use the data from the sensor to engage other citizens and local policymakers.
Survey respondents reported taking 24 individual actions after seeing the data for mobility on their street. The top three actions taken with WeCount data were: notified local government/responded to a consultation (); shared knowledge among the community () and applied for a neighbourhood action grant ()/notified the police, business, or other ().
Kruskal Wallis testing found a statistical difference between likelihood of action taken and two favourite aspects: the technology (; ) and ‘gathering evidence to support my campaign’ (; ). Those who preferred gathering evidence to support their mobility campaign or preferred the technological aspects of WeCount, were more likely to act than those that did not prefer these aspects.
Survey respondents’ motivation for joining also had a bearing on subsequent action taken. A Kruskall Wallis test revealed a statistical difference between the motivations ‘to count traffic’ (; ) and ‘to make a difference’ and subsequently action taken (; ). This means that these motivations were significantly more likely to lead to subsequent action than general interest in the issues or passive motivations (i.e., to contribute to research). In sum, action or technology-based motivations were good predictors of action taking. No correlation was found between demographic characteristics and action taken, although we expect this is an artefact of a low sample size. Enjoyment of WeCount and motivation to join had no impact on taking action.
Several citizens commented that involvement in WeCount strengthened pro-environmental behaviours:
I used to be really active until my life took a different turn, and so not active at all. Then this project kind of reminded me that that’s my nature. I want to go back to being more proactive about sustainable travel, promoting this. So yes, thanks for the reminder. (Cardiff Citizen Interview05)
Although the number of actions taken was modest, a relationship was found between situated knowledge/opinion change and action taken. There is a statistical correlation for instance between knowledge about ‘local traffic issues and solutions’ and action taken (). Participants were more likely to take action if they saw improvement to their local knowledge (as opposed to general knowledge). Meanwhile, the greater the opinion change at street () or neighbourhood level () the more likely citizens were to take action. On average, 45% ( of 209) of respondents who answered this survey question saw a change in opinion about traffic-related issues to some degree.
3.5 Creating community
The ability to self-sustain networks beyond the end of a project is crucial in terms of supporting ongoing citizen empowerment and future project development. There were positive signs that the WeCount communities could continue beyond the end of the project. Many citizens and stakeholders expressed a willingness to continue after WeCount officially ended (48%; ). Enjoyment and level of involvement significantly influenced willingness to continue. Respondents’ rating of their enjoyment showed highly significant differences in whether or not they were likely to continue, according to Kruskal Wallis (). Post-hoc testing (Mann-Whitney , , two-tailed) found that the mean rank for “Yes, I will continue” (53) was significantly lower than the mean rank for “No, I won’t” (91). As ranking was scaled from high to low (1 = excellent, 2 = good, etc.), these means signifies that those willing to continue were more likely to rate their time highly and vice versa. Looked at descriptively, 74% of participants who rated their time as excellent said they would continue ( of 80) — 33% said they were not sure if they would continue and 1% said they would not continue (). The more a participant enjoyed their time, the more likely they were to say they would continue working with WeCount data after the project ended.
3.6 Broader impacts
Ten staff members completed the ACTION framework impact scoring (Table 2 and Figure 4) and the interviews.
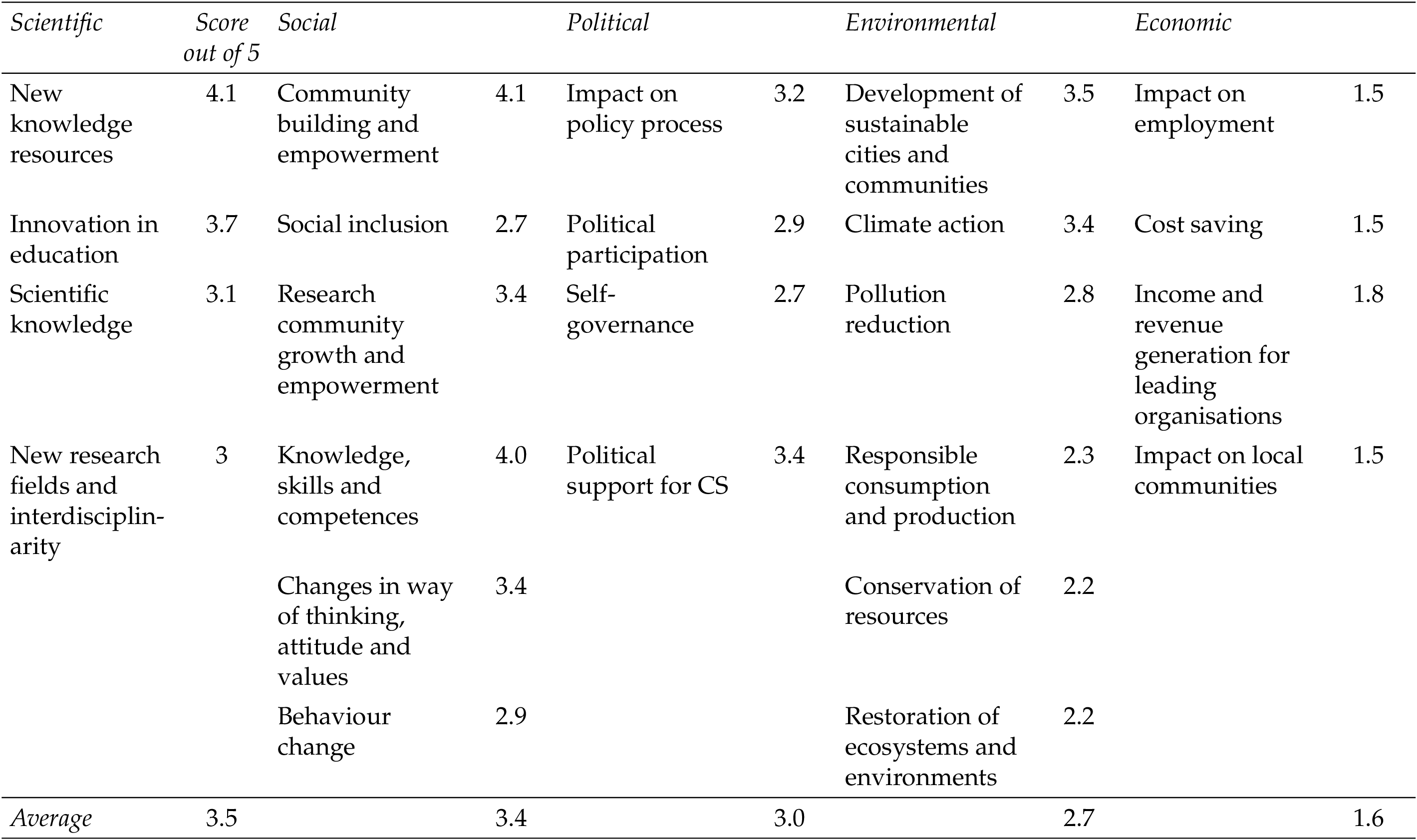
3.7 Scientific impact
Scientific impact was an important and inherent component of WeCount. It was scored most highly by the team, with an overall score of 3.5 out of 5. Looking at sub-domains, ‘innovation in education’ and ‘new knowledge resources’ scored slightly higher than the rest (3.7 and 4.1 respectively). Guidelines and toolkits were developed during the project which may have influenced the high score for knowledge resources. While the project team and research community gained new knowledge about citizen engagement and practices, staff members also thought the citizens gained new knowledge and awareness and that this new knowledge and data empowered citizens to share their understanding with others. It is clear from the survey results that these reflections align as citizens did gain new knowledge and took positive steps towards local sustainable mobility.
3.8 Social impact
This domain was ranked highly with a score of 3.4. Broken down by sub-domains, the average scores show substantial differences. ‘Social inclusion’ and ‘behavioural change’ received the lowest impact scores (2.7 and 2.9 respectively); ‘Community building and empowerment’ and ‘knowledge, skills and competences’ were given the highest impact scores (4.1 and 4.0, respectively) and this is likely due to the explicit efforts to build community at the start of the project and support citizens with how to sessions on sensor set-up and advocacy.
The team was surprised by how much participants took ownership of the data. Citizens analysed and discussed the data in innovative ways, such as exploring how much space would be required to park all the passing cars detected by the sensor. Sharing the data enabled the citizen scientists to develop as a community, for example:
I guess community building and empowerment. I gave it a four because I do believe that we were able, to some extent, to join individuals that were interested of different kinds and even different communities. (Staff Interview03)
3.9 Political impact
The political dimension of the ACTION framework aims to understand how CS results are being transferred and used. The expected political impact from WeCount was rather moderate (average score of 3). Impact scores by sub-dimension show that the greatest impact was on the political support for CS (3.4), and the lowest was on self-governance (2.7).
In the interviews, staff noted that political impact was a difficult domain in which to see change. While staff and citizens were keen to engage with politics to make changes locally, they indicated that policymakers were not always so transparent or willing to listen. For example:
The contact with the local government was a tough one. It really went up and down, but with a lot of up and downs. We did a lot of meetings and at many points we had the feeling that we were responsible for communication in-between departments of the city instead of them as one, talking to us, so that was really a challenge. (Staff Interview02)
However, staff members felt that the project and data opened doors for changes to be made.
3.10 Environmental impact
In general, the WeCount team ranked environmental impact quite low (2.7) as air quality or noise quality sensors were not the focus. The ‘development of sustainable cities and communities’ and ‘climate action’ scored highest (3.5 and 3.4, respectively) and ‘conservation of resources’ and ‘restoration of ecosystems and environments’ received the lowest impact scores (2.2 each).
3.11 Economic impact
Economic impact aims to understand to what extent CS can have an impact on both participating organisations and participants. The economic impact of WeCount was very low (average score of 1.6). Both the impact on ‘employment’, ‘cost-saving’ and ‘local communities’ received an average score of 1.5; while the impact on ‘income and ‘revenue generation for leading organisations’ received an average score of 1.8.
4 Discussion
WeCount is an example of democratic CS, unlike most CS projects which are designed to crowdsource or distribute intelligence [Sardo et al., 2022]. By focusing on co-creative and participatory methods, WeCount has added to the democratization of CS [Sauermann et al., 2020], where the scientist’s role shifts to co-designer and facilitator, with citizens and civil society organisations taking more of a central role in co-creation and in defining and addressing problems [Senabre Hidalgo et al., 2021].
An important consideration in environmental CS projects is the focus on addressing real-world problems and issues that concern local citizens [Phillips, Ballard, Lewenstein & Bonney, 2019], and this was a key motivator for citizens participating in WeCount. Pandya [2012] argues that when co-created CS projects focus on tackling real-world problems, the impact on public understanding is significant. It is clear from the evaluation that this approach works in making participants feel empowered.
Our findings show a significant link between levels of engagement and enjoyment: the more a participant enjoyed their time, the more likely they were to say they will continue working with WeCount data after the project ends. The link between enjoyment and taking part in CS projects is well described in the literature [Geoghegan, Dyke, Pateman, West & Everett, 2016; West, Pateman & Dyke, 2016]. For digital/computer-based projects, enjoyment is perceived as one of the main motivations to take part [Jennett et al., 2016]. By the end of the project, 10% of participants had taken action based on their experience with WeCount. This outcome is in line with the literature. The diffusion of innovation theory [Rogers, 2003] states it is unlikely that actions taken will exceed a small percentage of the engaged population.
Collaboration is key to the success of democratic CS projects, with research indicating it needs time, space and facilitation [Rolston III, 2011]. Where WeCount case studies worked closely with more active citizens it led to new avenues for exploration and greater opportunity to expand the network. Supporting people who feel less confident, with tailored training or doorstep assistance was also well received.
WeCount shows that CS brings people together while providing data which can make issues of relevance, such as urban mobility, visible to communities.
5 Conclusions
This study set out to explore key aspects involved in citizen participation in CS for sustainability and climate change resilience. WeCount enabled citizens in five European case studies to gather data on their own streets and utilise this data in their own sustainable mobility campaigns. While there is definite room for improvement (in terms of participant diversity and inclusion and the ability to continue after the project ends), WeCount has moved towards participatory CS for sustainable mobility.
In WeCount, efforts were made to emphasise the co-creative element of the project, with citizens having input in the sensor improvements, using visual observations, discussing data with other participants and co-designing data analysis. The Spanish case study exemplifies the importance of co-creation. Once sensors were deployed in Spain, citizens quickly started providing feedback that they were not suitable, due to unique architectural features of the city, such as balconies, which prevented the sensors from working. Co-creating with citizens, other tools were sought such as biosensors (strawberry plants that were used to monitor air quality). This meant that 1,000 additional citizens became involved in WeCount, measuring air quality with a biosensor. If the choice of sensor had been co-created from the onset of the project, alternative tools could have been co-developed and deployed earlier in the process.
This evaluation shows the importance of co-designing CS projects with citizens so that the projects are engaging, enjoyable and empowering to achieve sustainability transitions. The more a citizen enjoyed their time in the project, the more likely they reported intention to continue working with WeCount data after the project ends. This could eventually lead to taking more action. This paper demonstrates the suitability and value of CS to address and contribute to sustainable mobility issues and policies. By reflecting upon the comprehensive, multi-level, and multi-method evaluation of the experience of WeCount participants, this study offers practical insights on how democratic CS can be effectively carried out in this domain. Empirically-based learnings on how to achieve impact from citizen participation in WeCount are proposed to both academics and practitioners that aim at further exploring and implementing CS for sustainable mobility.
Acknowledgments
The authors would like to express their sincere gratitude to all the citizens who so kindly gave feedback as part of the evaluation. We are grateful to the whole WeCount consortium for their support in completing the evaluation. Ethical approval was received from the University of the West of England, Bristol Research Ethics Committee. This research was funded by the European Union’s Horizon 2020 Research and Innovation Programme, under grant agreement No. 872743.
References
-
Braun, V. & Clarke, V. (2006). Using thematic analysis in psychology. Qualitative Research in Psychology 3 (2), 77–101. doi:10.1191/1478088706qp063oa
-
Cappa, F., Franco, S. & Rosso, F. (2022). Citizens and cities: leveraging citizen science and big data for sustainable urban development. Business Strategy and the Environment 31 (2), 648–667. doi:10.1002/bse.2942
-
Carmichael, R. (2019). Behaviour change, public engagement and Net Zero. A report for the Committee on Climate Change. Imperial College London. London, U.K. Retrieved from http://www.imperial.ac.uk/icept/publications/
-
CitiMeasure (2023). Guidelines on behaviour and policy change. A set of recommendations for cities and the citizen science community. Retrieved from https://citimeasure.eu/change-guidelines/#page=1
-
European Commission (2020). Sustainable and Smart Mobility Strategy – putting European transport on track for the future. COM(2020) 789 final. Brussels, Belgium. Retrieved from https://eur-lex.europa.eu/resource.html?uri=cellar:5e601657-3b06-11eb-b27b-01aa75ed71a1.0001.02/DOC_1&format=PDF
-
Geoghegan, H., Dyke, A., Pateman, R., West, S. & Everett, G. (2016). Understanding motivations for citizen science. Final report on behalf of UKEOF. University of Reading, Stockholm Environment Institute (University of York) and University of the West of England. Retrieved from https://www.ukeof.org.uk/resources/citizen-science-resources/citizenscienceSUMMARYReportFINAL19052.pdf
-
Grand, A. & Sardo, A. M. (2017). What works in the field? Evaluating informal science events. Frontiers in Communication 2, 22. doi:10.3389/fcomm.2017.00022
-
Groves, R. M., Fowler, F. J., Couper, M. P., Lepkowski, J. M., Singer, E. & Tourangeau, R. (2004). Survey methodology. Hoboken, NJ, U.S.A.: Wiley-Interscience.
-
Jennett, C., Kloetzer, L., Schneider, D., Iacovides, I., Cox, A., Gold, M., … Talsi, Y. (2016). Motivations, learning and creativity in online citizen science. JCOM 15 (03), A05. doi:10.22323/2.15030205
-
Milfont, T. L. (2012). The interplay between knowledge, perceived efficacy, and concern about global warming and climate change: a one-year longitudinal study. Risk Analysis 32 (6), 1003–1020. doi:10.1111/j.1539-6924.2012.01800.x
-
Pandya, R. E. (2012). A framework for engaging diverse communities in citizen science in the US. Frontiers in Ecology and the Environment 10 (6), 314–317. doi:10.1890/120007
-
Passani, A., Janssen, A., Hölscher, K. & Di Lisio, G. (2022). A participatory, multidimensional and modular impact assessment methodology for citizen science projects. fteval Journal for Research and Technology Policy Evaluation 53 (54), 33–42. doi:10.22163/fteval.2022.569
-
Phillips, T. B., Ballard, H. L., Lewenstein, B. V. & Bonney, R. (2019). Engagement in science through citizen science: moving beyond data collection. Science Education 103 (3), 665–690. doi:10.1002/sce.21501
-
Rogers, E. M. (2003). Diffusion of innovations (5th ed.). New York, NY, U.S.A.: Free Press.
-
Rolston III, H. (2011). SuperCooperators: altruism, evolution, and why we need each other to succeed by Martin A. Nowak, with Roger Highfield. Zygon: Journal of Religion & Science 46 (4), 1003–1005. doi:10.1111/j.1467-9744.2011.01219.x
-
Sardo, A. M., Laggan, S., Franchois, E. & Fogg-Rogers, L. (2022). Reflecting on deepening participation in recruitment and evaluation in citizen science — lessons from the WeCount project. fteval Journal for Research and Technology Policy Evaluation 53 (54), 20–32. doi:10.22163/fteval.2022.568
-
Sauermann, H., Vohland, K., Antoniou, V., Balázs, B., Göbel, C., Karatzas, K., … Winter, S. (2020). Citizen science and sustainability transitions. Research Policy 49 (5), 103978. doi:10.1016/j.respol.2020.103978
-
Senabre Hidalgo, E., Perelló, J., Becker, F., Bonhoure, I., Legris, M. & Cigarini, A. (2021). Participation and co-creation in citizen science. In K. Vohland, A. Land-Zandstra, L. Ceccaroni, R. Lemmens, J. Perelló, M. Ponti, … K. Wagenknecht (Eds.), The science of citizen science (pp. 199–218). doi:10.1007/978-3-030-58278-4_11
-
West, S., Pateman, R. & Dyke, A. (2016). Data submission in citizen science projects. Report for Defra (Project number PH0475). Stockholm Environment Institute, University of York. Retrieved from https://www.york.ac.uk/media/sei/documents/publications/projectreports/West-Pateman-Dyke-DEFRA-Data-Submission-in-Citizen-Science-Projects.pdf
Authors
Ana Margarida Sardo (corresponding author).
Senior Research Fellow in Science Communication, at UWE Bristol, U.K.
E-mail: Margarida.Sardo@uwe.ac.uk
Sophie Laggan.
Research Fellow in Science Communication, at UWE Bristol, U.K.
@LagganSophie E-mail: sophie.laggan@uwe.ac.uk
Laura Fogg-Rogers.
Associate Professor for Engineering in Society, at UWE Bristol, U.K.
@LauraFoggRogers E-mail: laura.foggrogers@uwe.ac.uk
Elke Franchois.
Project Officer, Citizen Science at Mobiel 21, Belgium.
@EFranchois E-mail: elke.franchois@mobiel21.be
Giovanni Maccani.
Research Director at Ideas for Change, Spain.
@GiovanniMaccani E-mail: giovannimaccani@ideasforchange.com
Kris Vanherle.
Researcher at Transport & Mobility Leuven, Belgium.
@krisvanherle E-mail: kris.vanherle@tmleuven.be
Enda Hayes.
Professor of Air Quality & Carbon Management at UWE Bristol, U.K.
@HayesEnda E-mail: enda.hayes@uwe.ac.uk