1 Introduction
Broadening citizen participation in knowledge production about biodiversity is often presented as a priority by national and supra-national institutions and research agencies [ European Commission, 2013 ; Office of Science and Technology Policy, 2022 ]. In particular, a growing amount of attention and resources is devoted to citizen science’ programs [see Eitzel et al., 2017 , for a discussion on the meaning of this term]. This expression refers to a large variety of forms of participation by non-professional scientists (citizens, some NGOs members) in research, and mostly in environmental research [ Turrini, Dörler, Richter, Heigl & Bonn, 2018 ]. A majority of these citizen science projects consists of involving the public in identifying and surveying biodiversity [ Peter, Diekötter & Kremer, 2019 ]. These programs have largely demonstrated their efficiency to produce high-quality data that are useful for biodiversity monitoring and management [ Aceves-Bueno et al., 2017 ]. Besides their scientific relevance, other kinds of virtues are associated to biodiversity citizen science projects, such as helping participants to learn about ecology and biodiversity [ Phillips, Porticella, Constas & Bonney, 2018 ; Bonney, Phillips, Ballard & Enck, 2016 ] or restoring a form of public trust in experts’ advice regarding environment-related problems [ Ebel, Beitl, Runnebaum, Alden & Johnson, 2018 ]. In this context, a rich literature has emerged which explores the correlations of citizen participation with science learning [Peter et al., 2019 ]. These studies are generally motivated by the hypothesis that participation, by enabling citizens to take part in real-life research processes, may foster their familiarity both with the epistemological principles grounding knowledge production and their interest in sciences as a body of theoretical knowledge [ Aristeidou & Herodotou, 2020 ]. More sparsely, some studies have tried to evaluate the correlation between participation in biodiversity citizen science programs and the public trust placed in sciences [ Vitone et al., 2016 ]. However, there is still a glaring lack of evidence regarding the way citizen science might have an influence on the kind of trust placed in scientists, as well as in science in general and in specific disciplines such as ecology [ Wynne, 2006 ]. Our research is a first exploration of the forms of trust towards scientists and scientific results which characterize participants to biodiversity citizen science programs. In particular, we investigate the specificity of this citizen science-related trust compared to formal education regarding trust in science. Following the approach developed by Phillips et al. [ 2018 ] for framing the empirical assessments of citizen science’s learning outcomes, we first tried to distinguish different dimensions of trust in science, as a function of the exact object they refer to. We then considered five very similar biodiversity citizen science projects in France, which are part of the Vigie-Nature network of participative observatories, managed since 1989 by the Muséum National d’Histoire Naturelle (MNHN) in Paris, France. These five citizen science projects (hereafter, observatories) are open to the general public without prerequisite naturalist skills and are dedicated to surveying different groups of common species. These Vigie-Nature ’s observatories all share the same general organization: amateur citizens collect data, on a regular basis and with some form of standardized effort (specific protocol for each observatory), about a given species or group (pollinating insects, butterflies, birds, plants among others); they then share these data with scientists, who produce and publish scientific results, and disseminate them among participants.
We conducted our study by means of a questionnaire fully completed by 1,199 adults living in France and having, to different extents, some links with conservation NGOs associated with the observatories. Among them, 586 have participated to at least one of the Vigie-Nature observatories we consider in this study, the other 613 forming the non-participant group originating from a population that is most likely aware of these observatories but do not participate.
2 Trust in science and citizen science
Trust in governance has seen a steady and substantial decline across the world over the last decade (see, for instance, the Edelman Trust Barometer, https://www.edelman.com/trust/2022-trust-barometer ). Yet, in our “knowledge societies”, knowledge is considered as a commons and a public good, and it crucially informs policymaking processes and political action [ McCombs, 2008 ]; trust in governance is then closely related to trust in science, that is “the trust that society places in scientific research” [ Resnik, 2011 ]. Public trust in science has therefore become a key expression in science policy and ethics in recent years. Notably, concerns were raised about the decrease of this public trust in science [ Arimoto & Sato, 2012 ].
As formalized by Irzik and Kurtulmus [ 2019 ], an individual (M) placing its trust in a (group of) 72 scientists (S) as providers of information (P) means that “(S) believes that (P) honestly (that is, truthfully, accurately and wholly) communicates it to (M), either directly or indirectly… [and that] (P) is the output of reliable scientific research carried out by (S)” [Irzik & Kurtulmus, 2019 , pp. 1149–1150]. To put in more simply, an individual trusts S if she believes S is: a credible expert, that she produced her results with integrity , and that S is honest in communicating these results [see for instance Wintterlin et al., 2022 , on this distinction between expertise, integrity and honesty or benevolence as dimensions of trustworthiness]. An open question here is that of the determinants of one’s beliefs that (a group) of scientists is credible, honest and acts with integrity. Various criteria have been proposed in literature. For instance, Stern and Coleman [ 2015 , p. 122] distinguished four drivers of trust: dispositional trust (“the general tendency of an individual to trust or distrust another entity in a particular context”); rational trust (trust based on “evaluations of information about the trustee’s prior performance”); affinitive trust (based on the “cognitive or emotional assessment of the trustee’s integrity and/or benevolence”); and procedural trust (“trust in procedures or other systems that decrease the potential trustor’s vulnerability”). Given this general characterization, the very concept of public trust in science, and its epistemological, political, affective and psychological determinants remains quite ambiguous, basically because “the public” and “science” might themselves refer to different things [ Irzik & Kurtulmus, 2021 ]. For instance, “science” can both refer to the scientific endeavor and to the institutions leading scientific research: in this last case, it has been shown that the (private or public) origin of the fund strongly influences the strength of the trust placed in the generated scientific results [ Master & Resnik, 2013 ].
Another approach to trust in science then consists in starting from the concrete roles assigned to scientists and experts in our democratic societies: producing reliable and objective knowledge which can (eventually) be used to improve our lives (our health, comfort and security) and/or to solve collectively identified problems by feeding into public policies and public debates. This mere (rather consensual) claim already determines different objects to which trust can apply: (i) the scientific results which are produced and diffused to the public; (ii) the capacity of science to solve concrete problems we (as a society) face; (iii) the capacity of science to foster social progress; (iv) the utility of scientific data to guide public policies. Let us note here that these four dimensions of trust in science constitute an abstract and simplified model to assess public trust in science, notably because they do not take into account contextual factors, such the origin of the funds or the way science is communicated to the public [Master & Resnik, 2013 ]. Independently of these contextual factors, our point here is that there is no reason for these different dimensions of trust to be related: for instance, one can believe scientific results are honest and credible, but be skeptical regarding the capacity of science to foster social progress and well-being. In other words, empirical evaluation of public trust in science and scientists should differentiate all these different aspects — which are obviously not exhaustive. Yet, most of the existing empirical works on that topic have tried to measure trust by building scales which consider together different dimensions of trust in science. Nadelson et al. [ 2014 ] have been precursors in developing and testing a “ Trust in Science and Scientists Inventory ”. It consists of a 21-items test (such as “I trust scientists can find solutions to our major technological problems” and reversed phrase items such as “we cannot trust scientists because they are biased in their perspectives”), with a 5-points scale ranking. Chinn, Lane and Hart [ 2018 ] have measured “overall trust in science” by using a combined scale of three items: (1) “How much do you trust science in general?”, (2) “How credible is science in general?”, (3) “Scientists know what is good for the public”. This scale is supposed to capture together different dimensions of trust in science: general trust in science [ Gauchat, 2012 ], credibility of scientific information [ Liu & Priest, 2009 ], and deference to scientific actors [ Anderson, Scheufele, Brossard & Corley, 2012 ]. Finally, let us cite the Reliable short Credibility of Science scale from Hartman, Dieckmann, Sprenger, Stastny and DeMarree [ 2017 ]. It is a 6-item scale which aims to measure the “perceptions about the credibility of science”. 1
Among the factors that are expected to influence public trust in science, science knowledge or public understanding of science is perhaps the most obvious one [ Miller, 2004 ]. The classical “deficit model of science communication” [ Suldovsky, 2017 ] indeed postulates that attitudes of distrust or rejection of science are due to a lack of public knowledge and/or understanding [see Bodmer, 1985 , for a classical expression of this position]. This “deficit model” has been strongly criticized [ Smallman, 2018 ], but it is still not clear what are the relationships between this public knowledge or understanding of science, and the attitude towards scientific results and expertise — trust being one specific kind of attitude. Allum, Sturgis, Tabourazi and Brunton-Smith [ 2008 ] have reviewed a large number of works studying the link between knowledge and attitude with respect to science, and they find only “a small positive correlation between general attitudes towards science and general knowledge of scientific facts”.
More recently, public engagement in scientific research (through the collection of data and/or to their interpretation, to the setting of the research agenda, to the formulation of research questions, to the design of protocols, etc.) was defended as a way to improve the trust relationship between the public and scientists as providers of information — in particular the perception of the credibility of the scientists, and of their trustworthiness [ Eleta, Galdon Clavell, Righi & Balestrini, 2019 ]. As noted by Aitken, Cunningham-Burley and Pagliari [ 2016 ], “public engagement with science has now largely replaced public understanding as the key mechanism for addressing the crisis of public trust”. A literature survey on Web of Science and Scopus gives 138 articles that address citizen science and trust in either title, abstract or keyword. An overview of the associated abstract indicates that all of them deal with the trust relationships among the participants or between the participants and the researchers managing the project. Only one of them adress empirically the relationships between engagement in citizen science and trust in science in general: Füchslin, Schäfer and Metag [ 2019 ] reveal that people’s interest in participating in citizen science is not correlated with a greater general trust in science. Yet, this result relies on the answers to only one question (“How high is your trust in science in general?”). Our contribution aims to start filling this gap by exploring the correlations between citizen science, science knowledge and different dimensions of trust in science.
More precisely, our research question is then the following: which forms of trust are placed in science and scientists by participants associated with biodiversity observatories? In particular, what is the specificity (if any) of this participation-related public trust in science, compared to the kind of public trust related to more formal ways for citizens to approach sciences (namely, the acquisition of university degrees)? One hypothesis is that participation, by developing participants’ research skills and relationships with scientists, and by giving them the opportunity to better understand how science and scientists work, promotes a form of public trust similar to that acquired by university education — that is, mediated by greater knowledge of (general and specific) science contents and research processes. To test this hypothesis, our questionnaire simultaneously explored the level and nature of trust and the degree of science knowledge (in terms of general science knowledge, biodiversity knowledge, epistemological beliefs and reasoning skills). To explore trust, we decided to distinguish between five dimensions of trust in science, based on the previous definition of the roles of science and expertise: (i) the credibility and perceived honesty of scientific information as they are presented to the public; (ii) the capacity of science to foster social progress; (iii) the capacity of science to solve concrete problems; (iv) the utility of scientific data to guide public policies; (v) the integrity of scientists with regards to non-scientific interests (that may be financial or related to power, or linked to scientists’ personal values). This approach is distinct from existing empirical studies of trust in science, which generally aims to generate unified scales to measure citizens’ perception of trust in science [ Nadelson et al., 2014 ] or scientific credibility [ Hartman et al., 2017 ]. Let us note right now that some of the dimensions of trust we consider in our study are considered elsewhere as characterizing more generally “attitudes” towards science [see notably Wintterlin et al., 2022 ]. This choice is cogent with the conceptual frame we adopt here [that of Irzik & Kurtulmus, 2019 ].
3 Materials and methods
3.1 Vigie-Nature observatories
Vigie-Nature started in 1989. It intends to support biodiversity conservation policies by improving scientific knowledge of biodiversity. It engages a large diversity of people (amateur naturalists, green areas managers, pupils, farmers and other citizens) in the collection of field data across the whole French metropolitan territory (for more information, please consult http://www.vigienature.fr/fr/presentation-2831 ). Our study’s empirical basis was constituted by the five observatories specifically dedicated to non-professional citizens without prerequisite skills.
The “Spipoll” (launched in 2010) is designed to survey pollinator species assemblages. Participants apply the following standardized protocol. First, they select a flowering plant species (possibly several individuals of the same species within a ten-meter diameter circle) of their choice and take two pictures: one of the plant itself and one of the surrounding environment. Then, they take pictures of every insect visiting the flowers of the selected plant species during a 20-minute period. Second, they identify insects and plants using a dedicated online identification tool. Third, they upload their pictures and associated identification, as well as date, time, location of observations — on the Spipoll’s website, where data are shared among participants.
The “ Sauvage de ma rue ” (launched in 2011) project aims at studying urban wild vegetation. Whenever they want, participants choose a section of a street in their city, they identify plants by using an identification key, and they upload their data in the dedicated website.
“Birdlab” (launched in 2014) studies birds’ feeding behaviors. Participants install two bird feeding tables in their garden or terrace and, in an online application, register individual birds’ movements — landing on and departing from the two bird tables (over a 5-minute period). This may be repeated as often as desired by participants, all along the winter season.
“ Opération papillons ” (launched in 2006) aims to improve our knowledge of butterflies and their living environments. On a weekly basis, participants upload to the dedicated website a report about the butterflies they observed in their private gardens. These reports indicate, for every identified species, the largest number of individuals observed simultaneously during the week.
“ Oiseaux des jardins ” (launched in 2012) studies the effects of climate change, agriculture and urbanization on garden birds’ biodiversity. Participants record the number of individuals of every identified species who land in their gardens during an observation session (duration defined by the participant). This may be repeated as often as desired by the participants.
All these programs are designed on a free-contribution basis: participants collect and send data whenever they want. Besides, these five observatories are related to naturalist NGOs. These associations recruit participants through their own communication channels: newsletters, websites, etc. One important point: these associations also gather people who do not participate in Vigie-Nature citizen science programs. Let us point out here that we decided to consider these five programs as all together providing a unique sample of “participants”. Two reasons may justify that choice. First, these programs function on a very similar basis, and they all constitute typical instances of “contributory citizen science” in the sense of Bonney et al. [ 2009 ]. However, contrary to other contributory citizen science programs [see for example Jordan, Gray, Howe, Brooks & Ehrenfeld, 2011 ], there is no dedicated participants training: only detailed protocols are available online, as well as identification keys to help participants identify the species they report. Participants’ training and learning about biodiversity and the research process is then expected to develop gradually through participation itself.
Let us also say a word here about the content of the online resources posted by the programs’ managers on the dedicated websites. New resources are posted every two weeks in average under the form of “news” in both Vigie-Nature websites ( https://www.vigienature.fr/fr/actualites ), and in the different programs’ specific websites (e.g., https://www.vigienature.fr/fr/spipoll-0 ). Contents are as follows: about 60% of the publications present scientific results or data (including Vigie-Natures ’) in an accessible, easy-to-read format (for instance, new findings concerning the impact of pesticides on biodiversity, or interviews from experts on a specific topic regarding biodiversity); about 30% are interviews and testimonies from participants; and 10% present news about participative programs’ recent developments (evolution of the protocols, hiring of new partners, new design of the websites). The regular sharing of these resources, as well as the absence of formal training programs are Vigie-Nature observatories’ two main distinctive characteristics.
3.2 Questionnaire
Our questionnaire was first tested on a panel of eight people from our research unit (not belonging to the study, though) to collect their comments, and was corrected accordingly in order to clarify and adapt items, when needed. We then conducted the online survey (in French) from February to April 2021. This questionnaire was presented as a scientific study aiming to evaluate people’s perceptions of science. It was designed with LimeSurvey. All responses were completed online and were completely anonymized (including IP address) in order to comply with the French law on data privacy. Respondents were provided with a short text explaining the study’s main objectives. Given the aims of our study, we targeted both participants and non-participants to biodiversity conservation citizen science projects. To do so, we used different communication channels. First, the questionnaire was distributed through the main naturalist NGOs managing Vigie-Nature observatories (associations’ newsletters and websites). As explained previously, these associations also gather the details of people who — while being sympathetic to, or concerned with — the environment and biodiversity, do not participate in Vigie-Nature observatories. Second, it was posted on the Vigie-Nature website. Third, it was also disseminated within participants’ arenas of discussions, when available (forums, Facebook pages, etc.). We obtained 1,199 complete responses in total. Among them, 586 were part of (at least one of) the five Vigie-Nature observatories we focus on. 613 respondents never took part to a Vigie-Nature biodiversity citizen science project, while being linked to at least one of the corresponding naturalist NGOs. As a consequence, we expected a majority of these non-participants to feel relatively concerned with biodiversity issues compared to the general population living in France. This element is interesting, since it allows us to isolate the specificities of participation vis-à-vis mere interest towards biodiversity and/or environment sciences.
The questionnaire was built to get three types of information, presented in distinct sections: individual information and participation practices; general knowledge about science, reasoning skills and knowledge about biodiversity; trust placed in science and researchers.
3.2.1 Individual participation practices
An initial category of questions collected personal information: age, gender (man = 1, woman = 0, and an option “other”, which was never chosen), education (quantitative variable: 0 = Junior-high school; 1 = senior-high school; 2 = technical school; 3 = Bachelor; 4 = Master; 5 = Ph.D.), and participation to at least one of the Vigie-Nature biodiversity observatories (Yes = 1 or No = 0). Vigie-Nature participants were also asked about their participation practices:
-
Duration of their involvement in the observatories (in years, Duration explanatory variable)
-
Intensity of their participation, measured as the average quantity of data uploaded per year (as declared by respondents). Since each program differs in the frequency of data being sent, we scaled the related variable Intensity by dividing it by the average value obtained in each program.
-
Reading of scientific publications available on participation websites, written based on these data (Yes or no, Publication variable).
-
Frequency of consultation of the online resources posted by coordination teams within the programs’ websites (5-point Likert scale, Resources variable).
Please note we included age and gender in our correlation models in order to control the influence of these variables; however, the specific discussion of their effects exceeds the scope of the paper. As far as there is no interaction between these variables and participation within the correlation models, we will not discuss them in the interpretation of our results.
3.2.2 Knowledge about science and biodiversity
A set of eight questions (listed in appendix A ) was proposed to assess the level of general knowledge about science ( Science content response variable). Questions were all taken from the classical science literacy index, sometimes known as the “Oxford scale” [ Stocklmayer & Bryant, 2012 ]. This scale is used both in academic studies [ Miller, 1998 ] and in institutional surveys such as the Eurobarometers or the U.S. science and engineering indicators [ National Science Board, 2016 ]. Translations from English to French and from French to English (notably for appendix A ) was done in a collaborative way within the research team during a meeting. A score of individuals’ general knowledge of science was computed by assigning 1 to correct answers, and 0 to false ones. We then sum the score obtained by each participants. The Cronbach’s alpha for this set of questions is 0.9. However, we would like to point out we do not claim to provide a score which would measure “science knowledge” as a clear-cut concept. We envisioned our set of questions more as a science knowledge test . Consequently, we did not perform reliability analysis to select scale items. The same remark applies for the three following variables ( Biodiversity , Process , and Reasoning variables). In each case, we then give the Cronbach’s alpha values as supplementary indicators, but do not discuss them.
To assess knowledge about biodiversity ( Biodiversity response variable), we asked six questions (listed in appendix A ) all derived from Prévot, Cheval, Raymond and Cosquer [ 2018 ]. They were used together to test knowledge about biodiversity. We also asked Vigie-Nature participants one self-evaluation question (that is, a question where respondents are invited to evaluate by themselves the impact of their participation) about their perception of the knowledge they have gained about biodiversity by taking part in (at least) one of the participative observatories (4-point Likert scale, Biodiversity evaluation response variable). Cronbach’s alpha for these questions was 0.6.
Third, we assessed epistemological beliefs about the nature of the research process ( Processes response variable), with a list of four statements (Cronbach’s alpha , see appendix A for items) all taken or adapted from Liang et al. [ 2006 ]’s Student Understanding of Science and Scientific Inquiry scale. This scale consists in questions such as “Scientific theories based on accurate experimentation will not be changed” (5-point Likert-scale). This scale was used to test epistemological beliefs about the research process.
Finally, we designed a 4-question scale (Cronbach’s alpha , see appendix A for items) to assess their respective levels of mastering scientific reasoning skills (e.g., the distinction between correlation and causality, Reasoning response variable, 4-point Likert scale). We also designed one self-evaluation question asking Vigie-Nature participants whether their involvement had improved their understanding of “the way science works” (4-point Likert scale, Processes evaluation variable).
3.2.3 Trust in science
The last section of the questionnaire evaluates different dimensions of public trust in science (5-question, 5-point Likert scale from “I strongly disagree” to “I strongly agree”).
-
Trust in researcher’s honesty: “We can trust researchers to honestly communicate about their results” ( Honesty response variable). Adapted from Jensen and Hurley [ 2012 ].
-
Trust in science as a factor of social progress: “Science and technology are making our lives healthier, easier, and more comfortable” ( Progress ). Taken from Ross, Struminger, Winking and Wedemeyer-Strombel [ 2018 ].
-
Trust in technical solutions to environmental problem: “Technical progress will allow us to mend environmental harm caused by our activities” ( Techniques ). Taken from Prévot et al. [ 2018 ].
-
Trust in science as providing data useful for science policy (hereafter, trust in evidence-based policy): “Without scientific data, the government cannot make responsible policies that are in people’s best interests” ( Politics ). Taken from Ross et al. [ 2018 ].
-
Trust in researcher’s integrity: “When researchers disagree, it is often because they defend financial interests” ( Integrity , reverse coding).
The Cronbach’s for these items is 0.48, which confirms that they represent distinct dimensions of trust that cannot be merge in one scale.
3.3 Statistical analysis
We performed all the statistical analysis in Matlab R2021a. To test the correlation between our explanatory variables (demographic information and participation practices) and science knowledge, we computed generalized linear models with mean levels of knowledge (general knowledge, reasoning skills and knowledge about biodiversity) as response variables. For each of these response variables, we first used the glmfit Matlab function to find the regression coefficients and the corresponding p -values associated to explanatory variables. The explanatory models of trust in science were assessed by ordinal modeling, since the corresponding response variables take their values on 5- or 6-level Likert-scales. Here, we used the fitlm Matlab function, which fits ordinal multinomial regression models. We also used this method to assess correlation between demographic information and individuals’ participation features. In these models, we took into account interaction terms between the Participation variable and the different demographic variables. We then applied stepwise regression methods to identify those variable which significantly improve the fit (we compared the regression models by computing likelihood ratio tests). We finally checked for the homoscedasticity of the residuals from the regression models by using archtest Matlab function. This procedure (which is equivalent to a Type II Anova) enables us to assess the effect of each variable by taking all the other ones into account. Collinearity between demographic variables (gender, age, and diploma) were found to be low enough to integrate them in the same models (between 0.06–0.14).
4 Results
4.1 Characteristics of the sample and participation practices
Table 1 gives respondents’ key-demographic features, both for Vigie-Nature participants and non-participants. The “Participants” group was significantly older than the “Non-participants” cohort (t-test statistics , , ).
This result confirms the well-known finding that participants to contributory projects are relatively older and then more likely to be retired than the general population [ Merenlender, Crall, Drill, Prysby & Ballard, 2016 ]. There were also significantly more men in the “Participants” group ( , , ), and participants were less educated than non-participants ( , , ): 57% of participants and 66% of non-participants hold a university degree. Even if a bias does exist regarding the French population generally (40% of citizens have a university degree, see https://www.insee.fr/fr/statistiques/2416872 ), these statistics suggest Vigie-Nature observatories are rather inclusive regarding education, in that they do not over-select educated people among the pool of individuals already interested in environmental and biodiversity issues (notably, naturalist associations’ members).
Participants were engaged in the different Vigie-Nature projects for years, on average. We did not find any correlation between demographic variables and participation intensity or duration. However, participants who declared reading scientific publications were significantly more educated that the others (Beta coefficient of the regression: , p-value ). Interestingly, we found a significant negative correlation between education level and the Resources variable: people with less diploma consult more frequently than others the news and scientific results posted by researchers on the projects’ websites (Beta coefficient: , p-value ).
4.2 Participation is not correlated with science knowledge
Table 2 presents the results of the correlation models for the Science content , Reasoning , Processes and Biodiversity response variables. These different dimensions of scientific knowledge are all correlated with education level ( for content , reasoning and biodiversity ; for Processes ), and not with participation.
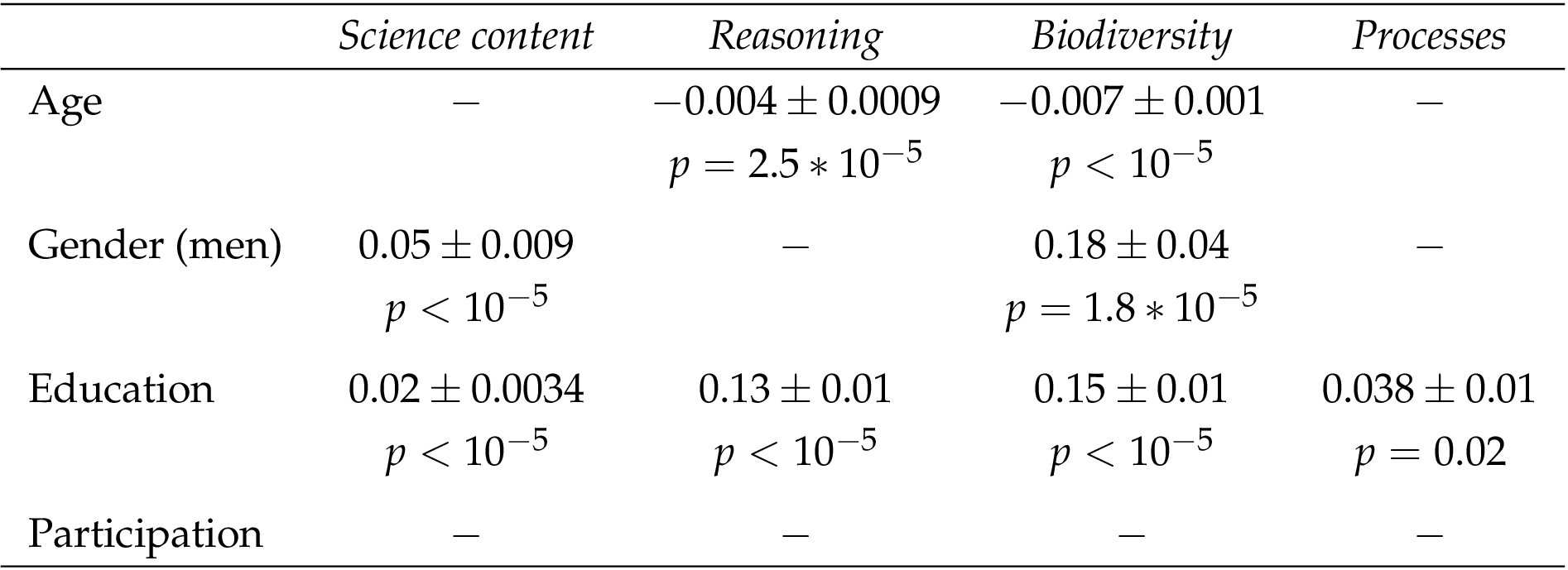
Regarding the self-evaluation questions ( Biodiversity evaluation and Processes evaluation variables), on a 0 to 3 scale, participants posted on average when they were asked if being a citizen scientist helped them to better understand “how science works” (between “I don’t think so” and “I think it did”). When they were asked if being a citizen scientist helped them to improve their knowledge of biodiversity, they posted on average (between “I think it did” and “totally”). Even if these two questions measure different things and are not directly comparable, it is still interesting to note that participation seems to be more impactful regarding biodiversity theoretical knowledge than regarding the knowledge of science process (when comparing the answers to the two self-evaluation questions, the result is significantly higher for the biodiversity-related one than for the science process-related one, with p-value under a Student test).
4.3 Participation is positively correlated with trust in science, and this effect is reinforced by the consultation of scientific information shared by projects’ managers
Table 3 presents the correlation models for the response variables related to different dimensions of public trust in science: trust in research honesty, trust in science as a factor of social progress, trust in technical solutions to environmental problems, trust in science-based policy, and trust in research independence with respect to financial interest.
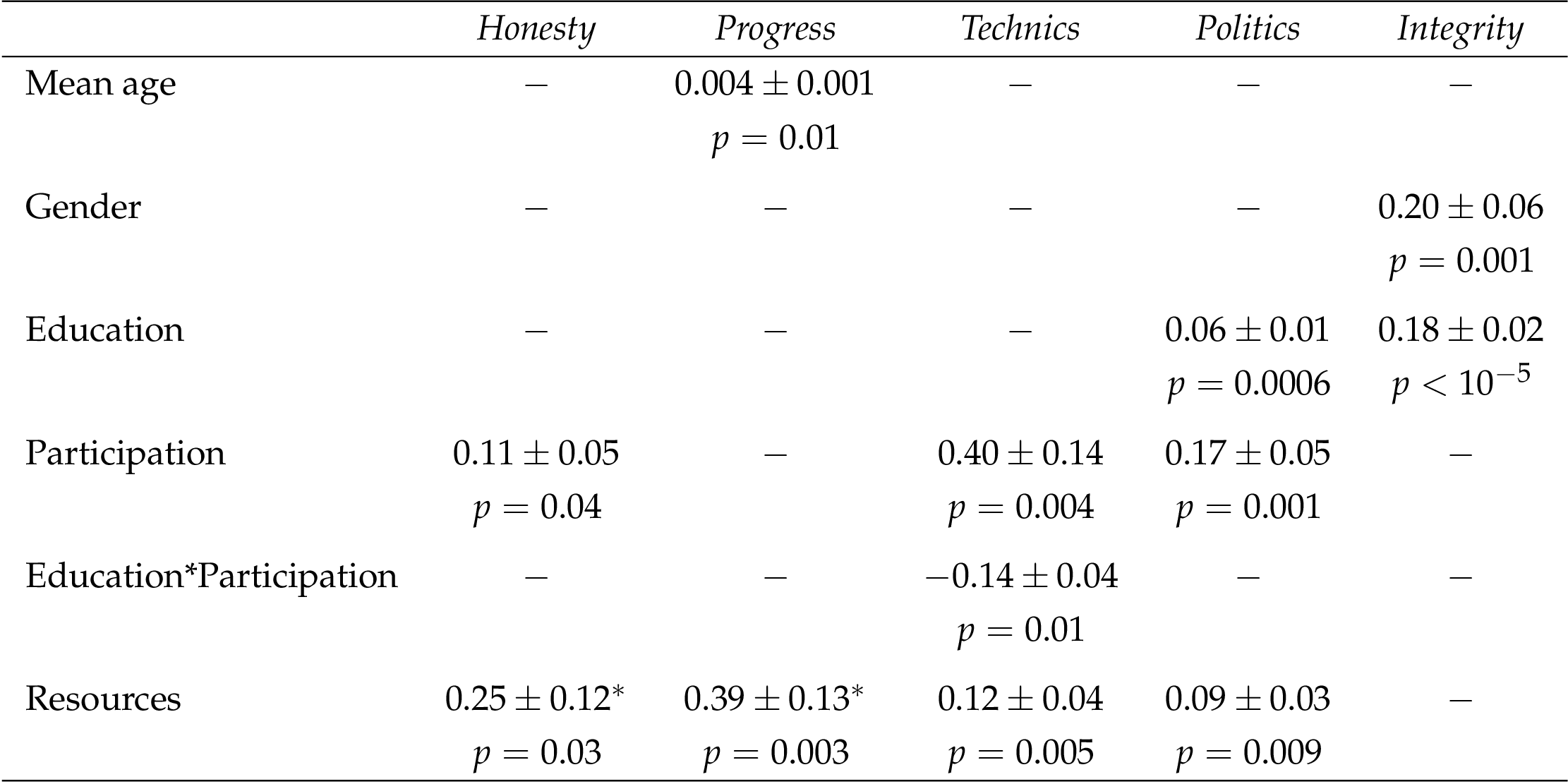
Results show that participation was positively correlated with the Honesty , Techniques and Politics variables, which indicates a greater trust along the corresponding dimensions for citizen scientists. The Techniques variable was negatively correlated with the interaction term EducationÕParticipation, suggesting that the positive effect of participation on trust in technical solutions decreases with education level. Interestingly, the consultation of the resources posted on the projects’ websites ( Resources variable) was also correlated positively with these three dimensions of trust in science. Besides, participants consulting these resources most frequently were also significantly more confident than non-participants regarding science as a factor of social progress ( Progress variable). It is worth noting that regarding the Honesty and Progress variables, the correlation with the consultation of resources was significant only between participants who “Systematically” consult these resources and those who “Never” do so. Finally, we did not find correlation between the different dimensions of trust and the duration or intensity of the engagement in citizen science.
Figures 1 , 2 and 3 illustrate the correlations between these dimensions of trust in science, and the explanatory variables Participation , Resources , and Education . We note that even if ordinal regression does not indicate a significant correlation between trust in technical solutions and education level (5 ordinal degrees), participants without a university degree have a higher degree of trust than citizens holding one ( , see Figure 2 ).
5 Discussion
This study focuses on the forms of trust in science associated with participation in biodiversity citizen science projects. To our knowledge, it is the first study which directly targets this issue in an empirical way. An originality of our work is to acknowledge the diversity of the objects to which trust in science might apply. Contrary to existing studies which build scales of trust [e.g. Nadelson et al., 2014 ; Hartman et al., 2017 ], we designed our survey so as to recognize this diversity (and to take it as a matter of discussion in itself).
5.1 Participation and education are differently correlated with trust in science
Our main result is that participation and education are differently correlated with trust in science. Participation is positively correlated with trust in researchers’ honesty when communicating results, with trust in science as providing data to be used in public policies, and with trust in technical solutions to environmental problems. In addition, there are also positive correlations between these three trust variables and the consultation of the online resources shared by projects’ managers. Besides, participants who “systematically” consult these resources show a significantly higher level of trust in science as a source of social progress. By contrast, education is not correlated with trust in scientists’ honesty and with trust in science as a factor of social progress. Furthermore, the positive correlation of participation with trust in technical solutions decreases with education level (more precisely, there is no correlation with individuals with a university degree). Let us note here that we conducted the same analysis by considering science knowledge instead of the education level, with similar results: that is, we found the same distinction between participation-related and knowledge-related trust (which was totally predictable, since education and science knowledge are linearly related). In order to make sense of these results, we note that participation (if we add the consultation of online resources) is positively correlated with four out of our five dimensions of trust, whereas education is positively correlated only with two of them, and seems to negatively impact one dimension (trust in technical solution). This perspective goes in the direction of the now classical criticism of the “deficit model” of public attitudes towards science — basically: the lack of support or trust in science comes from a deficit in science knowledge [e.g., see Smallman, 2018 ]. This idea has been criticized notably because it has been shown that people with more science knowledge or with more experience with science do not necessarily have more positive attitudes towards science in general, or specific science topics [ Evans & Durant, 1995 ; Drummond & Fischhoff, 2017 ; Wintterlin et al., 2022 ]. Our results confirm that education is not a direct predictor of all dimensions of trust in science. More precisely, education is not correlated with (or even seems to have a negative impact on) those dimensions of trust most related to a form of positivist vision of science. We take the notion of positivism in the sense of Wintterlin et al. [ 2022 ], which define and measure a “positivist attitude” towards science as the belief that science will gradually foster social program by solving most of the problem we (as a society) are facing. The authors measure this positivist attitude with a set of five items, with two of them corresponding to our Technics and Progress variables (“Science and research can solve any problem” and “Science and research improve our lives”). By contrast, participation seems to go with a less selective trust in science. An exception is for the belief that scientific controversies are fuelled by financial interests: education has a strong negative impact on that view, whereas we did not find any correlation with participation. Our hypothesis is that this aspect of trust is mainly driven by the knowledge of the “nature of science” [ Phillips et al., 2018 ], that is, the idea that the research process often generates disagreements which are epistemically grounded. This hypothesis of a positivist form of trust as a characteristic of participants to citizen science programs should now be investigated further. Different methodologies might be used. First, new items could be developed to generate a scale which would investigate more precisely each of the dimensions of trust we have shown to be relevant to characterize participation-related trust. Second, qualitative studies could be done to analyze more thoroughly attitudes towards science in the context of citizen science. Finally, these studies should be done by following a “pre-post” methodology [ Peter et al., 2019 ] in order to assess the direction of the causal links between participation and trust.
5.2 Limits of the study
To conclude, this exploratory study aim to identify which forms of trust are susceptible to be specifically (that is, independently of other variables such as education level) associated with engagement in citizen science. Because of its exploratory nature, this work has various limits we should consider. First, our study was not built to infer causal relationship between participation and trust: we only assess correlations. However, it is worth noting that we considered the level of engagement (intensity of participation and duration) as explanatory variables, and we did not find any correlations with our tested variables. This result could be interpreted as an indication that there might not be causal relationships between participation and trust. By contrast, it seems reasonable to consider education as a causal variable: that is, measured “effects” of education on outcome variables (trust and science knowledge) will be interpreted as caused by the acquisition of a certain education level. A second limit derives from the recruitment of the respondents: we sent the survey through the communication channels from naturalist associations. Consequently, there is a bias towards individuals who are interested in biodiversity and environment issues in the non-participant group. If this bias might limit the generalization of our results to the whole population, it might also be seen as a strength for the present study, since it reinforces the argument that the measured features are specific to participation. A third limit comes from the survey itself. We made the choice to assess each dimension of trust with only one item, to limit the length of the questionnaire and reach a large sample of respondents. This choice is justified by the exploratory nature of our study: our objective is to propose a first pattern of participation-related trust by identifying differences between participation-related and education-related trust. That said, we argue our study is valuable as it opens a way for future researches. First, new studies could verify our results by building robust scales addressing each of the relevant dimensions of trust. Notably, new items could be developed to generate a scale which would investigate more precisely each of the dimensions of trust we have shown to be relevant to characterize participation-related trust. Second, qualitative studies could be done to analyze more thoroughly attitudes towards science in the context of citizen science. These studies could be done by following a “pre-post” methodology [ Peter et al., 2019 ] in order to assess the direction of the causal links between participation and trust.
A Scales used to assess science knowledge
A.1 Knowledge of science content (1 – yes; 0 – no)
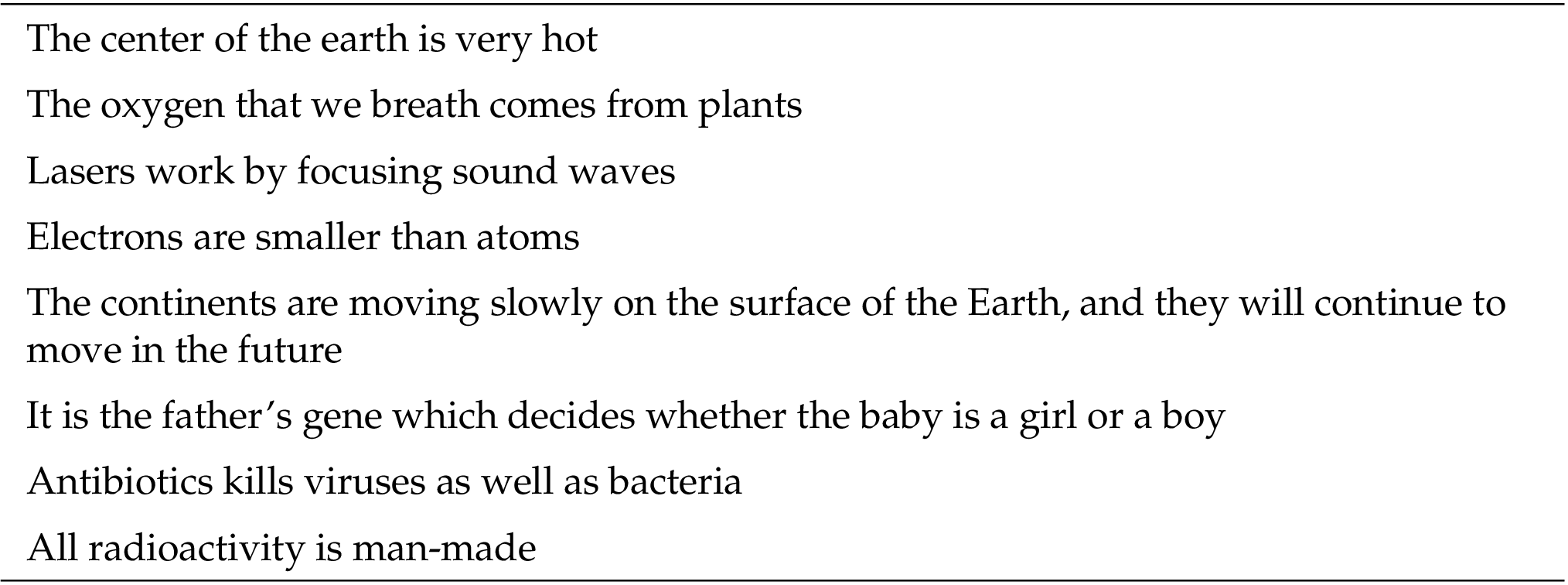
A.2 Knowledge about biodiversity (1 – I strongly disagree; 2 – I rather disagree; 3 – I moderately agree; 4 – I rather agree; 5 – I totally agree)
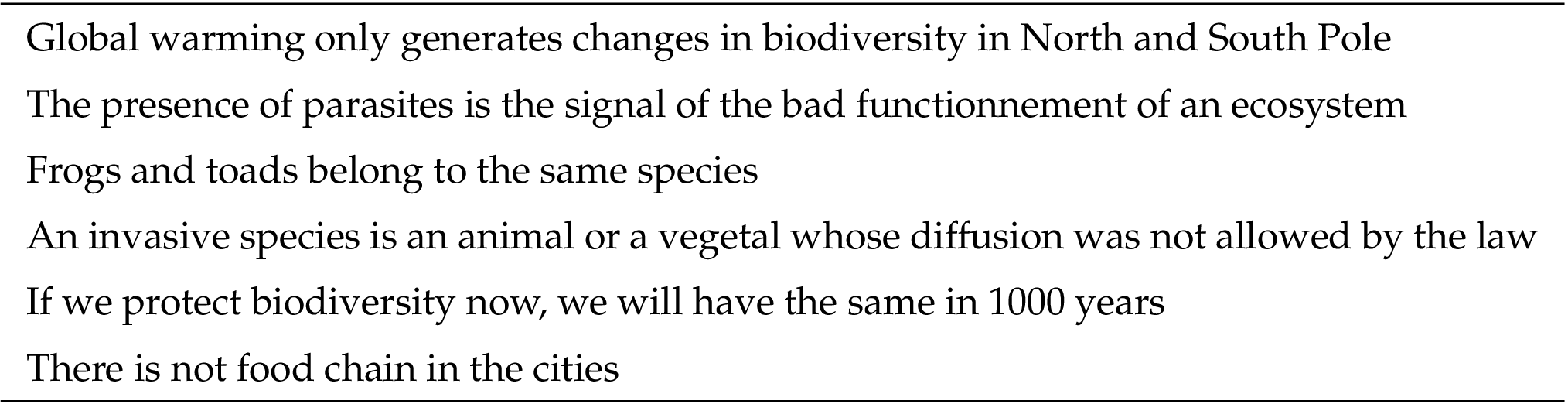
A.3 Epistemological believes about the nature of the research process (1 – I strongly disagree; 2 – I rather disagree; 3 – I moderately agree; 4 – I rather agree; 5 – I totally agree)
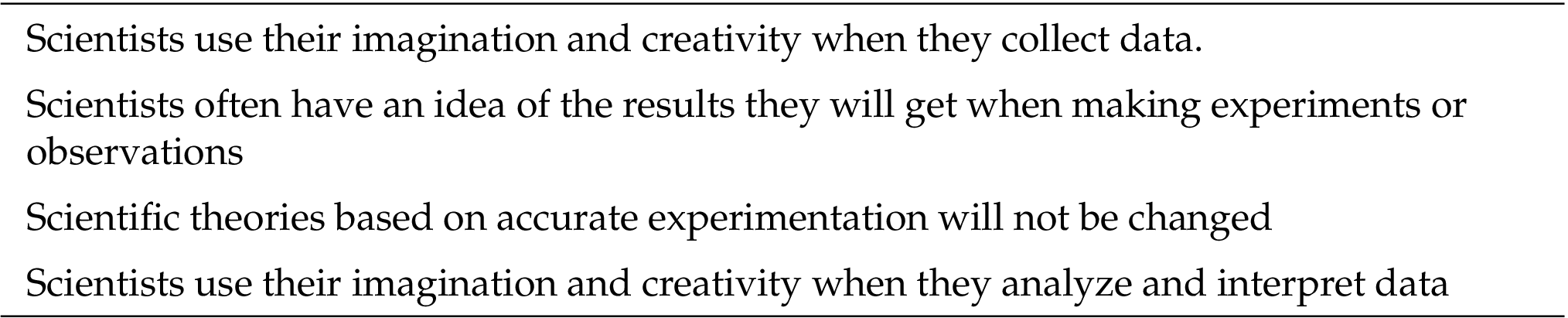
A.4 Reasoning skills (1 – not at all; 2 – I do not think so; 3 – I think yes; 4 – totally)
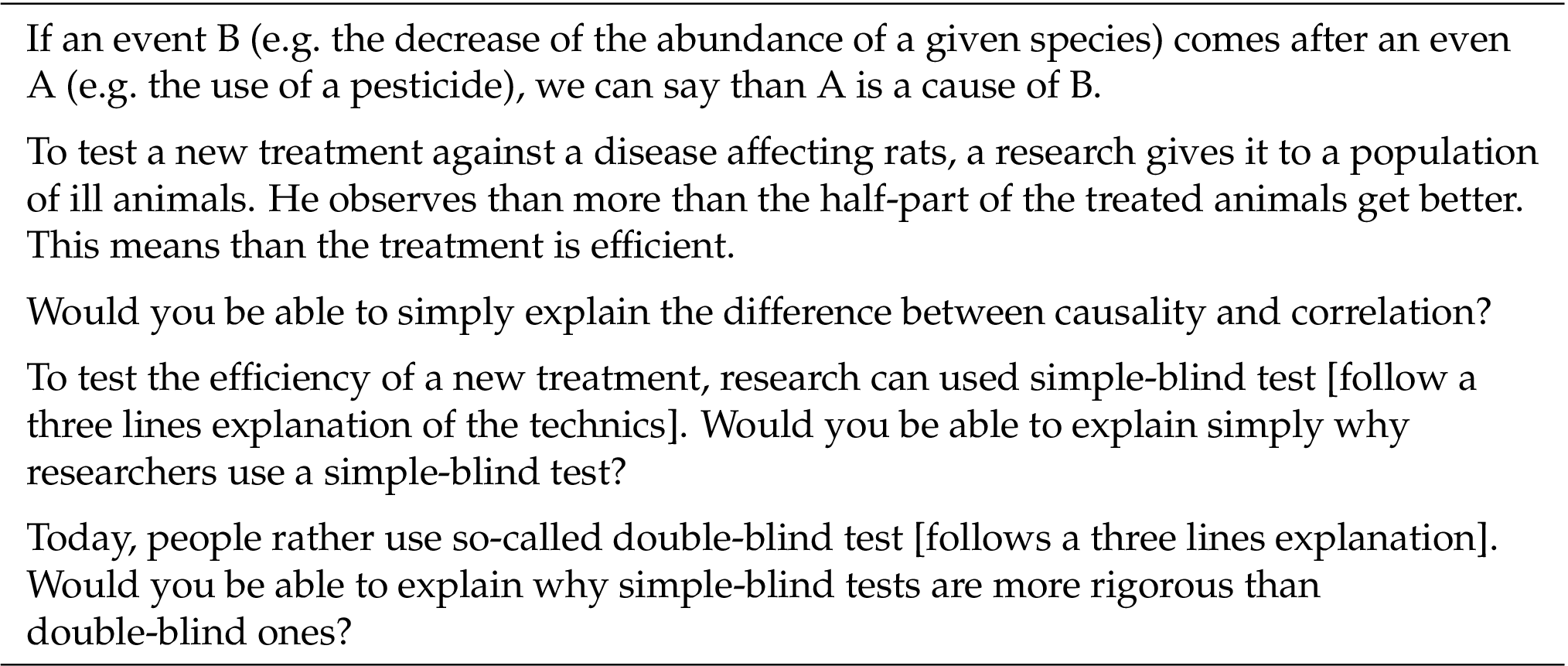
References
-
Aceves-Bueno, E., Adeleye, A. S., Feraud, M., Huang, Y., Tao, M., Yang, Y. & Anderson, S. E. (2017). The accuracy of citizen science data: a quantitative review. Bulletin of the Ecological Society of America 98 (4), 278–290. doi: 10.1002/bes2.1336
-
Aitken, M., Cunningham-Burley, S. & Pagliari, C. (2016). Moving from trust to trustworthiness: experiences of public engagement in the Scottish Health Informatics Programme. Science and Public Policy 43 (5), 713–723. doi: 10.1093/scipol/scv075
-
Allum, N., Sturgis, P., Tabourazi, D. & Brunton-Smith, I. (2008). Science knowledge and attitudes across cultures: a meta-analysis. Public Understanding of Science 17 (1), 35–54. doi: 10.1177/0963662506070159
-
Anderson, A. A., Scheufele, D. A., Brossard, D. & Corley, E. A. (2012). The role of media and deference to scientific authority in cultivating trust in sources of information about emerging technologies. International Journal of Public Opinion Research 24 (2), 225–237. doi: 10.1093/ijpor/edr032
-
Arimoto, T. & Sato, Y. (2012). Rebuilding public trust in science for policy-making. Science 337 (6099), 1176–1177. doi: 10.1126/science.1224004
-
Aristeidou, M. & Herodotou, C. (2020). Online citizen science: a systematic review of effects on learning and scientific literacy. Citizen Science: Theory and Practice 5 (1), 11. doi: 10.5334/cstp.224
-
Bodmer, W. F. (1985). The public understanding of science . The Royal Society. London, U.K.
-
Bonney, R., Ballard, H., Jordan, R., McCallie, E., Phillips, T., Shirk, J. & Wilderman, C. C. (2009). Public participation in scientific research: defining the field and assessing its potential for informal science education. A CAISE Inquiry Group report . Center for Advancement of Informal Science Education. Washington, DC, U.S.A. Retrieved from https://resources.informalscience.org/public-participation-scientific-research-defining-field-and-assessing-its-potential-informal-science
-
Bonney, R., Phillips, T. B., Ballard, H. L. & Enck, J. W. (2016). Can citizen science enhance public understanding of science? Public Understanding of Science 25 (1), 2–16. doi: 10.1177/0963662515607406
-
Chinn, S., Lane, D. S. & Hart, P. S. (2018). In consensus we trust? Persuasive effects of scientific consensus communication. Public Understanding of Science 27 (7), 807–823. doi: 10.1177/0963662518791094
-
Drummond, C. & Fischhoff, B. (2017). Individuals with greater science literacy and education have more polarized beliefs on controversial science topics. Proceedings of the National Academy of Sciences 114 (36), 9587–9592. doi: 10.1073/pnas.1704882114
-
Ebel, S. A., Beitl, C. M., Runnebaum, J., Alden, R. & Johnson, T. R. (2018). The power of participation: challenges and opportunities for facilitating trust in cooperative fisheries research in the Maine lobster fishery. Marine Policy 90 , 47–54. doi: 10.1016/j.marpol.2018.01.007
-
Eitzel, M. V., Cappadonna, J. L., Santos-Lang, C., Duerr, R. E., Virapongse, A., West, S. E., … Jiang, Q. (2017). Citizen science terminology matters: exploring key terms. Citizen Science: Theory and Practice 2 (1), 1. doi: 10.5334/cstp.96
-
Eleta, I., Galdon Clavell, G., Righi, V. & Balestrini, M. (2019). The promise of participation and decision-making power in citizen science. Citizen Science: Theory and Practice 4 (1), 8. doi: 10.5334/cstp.171
-
European Commission (2013). Science for environment policy in-depth report: environmental citizen science . Retrieved from https://aarhusclearinghouse.unece.org/news/science-for-environment-policy-depth-report-environmental-citizen-science
-
Evans, G. & Durant, J. (1995). The relationship between knowledge and attitudes in the public understanding of science in Britain. Public Understanding of Science 4 (1), 57–74. doi: 10.1088/0963-6625/4/1/004
-
Füchslin, T., Schäfer, M. S. & Metag, J. (2019). Who wants to be a citizen scientist? Identifying the potential of citizen science and target segments in Switzerland. Public Understanding of Science 28 (6), 652–668. doi: 10.1177/0963662519852020
-
Gauchat, G. (2012). Politicization of science in the public sphere: a study of public trust in the United States, 1974 to 2010. American Sociological Review 77 (2), 167–187. doi: 10.1177/0003122412438225
-
Hartman, R. O., Dieckmann, N. F., Sprenger, A. M., Stastny, B. J. & DeMarree, K. G. (2017). Modeling attitudes toward science: development and validation of the credibility of science scale. Basic and Applied Social Psychology 39 (6), 358–371. doi: 10.1080/01973533.2017.1372284
-
Irzik, G. & Kurtulmus, F. (2019). What is epistemic public trust in science? The British Journal for the Philosophy of Science 70 (4), 1145–1166. doi: 10.1093/bjps/axy007
-
Irzik, G. & Kurtulmus, F. (2021). Well-ordered science and public trust in science. Synthese 198 (S19), 4731–4748. doi: 10.1007/s11229-018-02022-7
-
Jensen, J. D. & Hurley, R. J. (2012). Conflicting stories about public scientific controversies: effects of news convergence and divergence on scientists’ credibility. Public Understanding of Science 21 (6), 689–704. doi: 10.1177/0963662510387759
-
Jordan, R. C., Gray, S. A., Howe, D. V., Brooks, W. R. & Ehrenfeld, J. G. (2011). Knowledge gain and behavioral change in citizen-science programs. Conservation Biology 25 (6), 1148–1154. doi: 10.1111/j.1523-1739.2011.01745.x
-
Liang, L. L., Chen, S., Chen, X., Kaya, O. N., Adams, A. D., Macklin, M. & Ebenezer, J. (2006). Student understanding of science and scientific inquiry (SUSSI): revision and further validation of an assessment instrument, 3rd–6th April 2006. Annual Conference of the National Association for Research in Science Teaching (NARST). San Francisco, CA, U.S.A.
-
Liu, H. & Priest, S. (2009). Understanding public support for stem cell research: media communication, interpersonal communication and trust in key actors. Public Understanding of Science 18 (6), 704–718. doi: 10.1177/0963662508097625
-
Master, Z. & Resnik, D. B. (2013). Hype and public trust in science. Science and Engineering Ethics 19 (2), 321–335. doi: 10.1007/s11948-011-9327-6
-
McCombs, G. M. (2008). Understanding knowledge as a commons: from theory to practice. College and Research Libraries 69 (1), 92–94. doi: 10.5860/crl.69.1.92
-
Merenlender, A. M., Crall, A. W., Drill, S., Prysby, M. & Ballard, H. (2016). Evaluating environmental education, citizen science, and stewardship through naturalist programs. Conservation Biology 30 (6), 1255–1265. doi: 10.1111/cobi.12737
-
Miller, J. D. (1998). The measurement of civic scientific literacy. Public Understanding of Science 7 (3), 203–223. doi: 10.1088/0963-6625/7/3/001
-
Miller, J. D. (2004). Public understanding of, and attitudes toward, scientific research: what we know and what we need to know. Public Understanding of Science 13 (3), 273–294. doi: 10.1177/0963662504044908
-
Nadelson, L., Jorcyk, C., Yang, D., Jarratt Smith, M., Matson, S., Cornell, K. & Husting, V. (2014). I just don’t trust them: the development and validation of an assessment instrument to measure trust in science and scientists. School Science and Mathematics 114 (2), 76–86. doi: 10.1111/ssm.12051
-
National Science Board (2016). Science and engineering indicators . National Science Foundation. Arlington, VA, U.S.A. Retrieved from https://www.nsf.gov/statistics/2016/nsb20161/#/
-
Office of Science and Technology Policy (2022). Implementation of federal prize and citizen science authority: fiscal years 2019–20 . Retrieved from https://www.whitehouse.gov/wp-content/uploads/2022/05/05-2022-Implementation-of-Federal-Prize-and-Citizen-Science-Authority.pdf
-
Peter, M., Diekötter, T. & Kremer, K. (2019). Participant outcomes of biodiversity citizen science projects: a systematic literature review. Sustainability 11 (10), 2780. doi: 10.3390/su11102780
-
Phillips, T., Porticella, N., Constas, M. & Bonney, R. (2018). A framework for articulating and measuring individual learning outcomes from participation in citizen science. Citizen Science: Theory and Practice 3 (2), 3. doi: 10.5334/cstp.126
-
Prévot, A.-C., Cheval, H., Raymond, R. & Cosquer, A. (2018). Routine experiences of nature in cities can increase personal commitment toward biodiversity conservation. Biological Conservation 226 , 1–8. doi: 10.1016/j.biocon.2018.07.008
-
Resnik, D. B. (2011). Scientific research and the public trust. Science and Engineering Ethics 17 (3), 399–409. doi: 10.1007/s11948-010-9210-x
-
Ross, A. D., Struminger, R., Winking, J. & Wedemeyer-Strombel, K. R. (2018). Science as a public good: findings from a survey of March for Science participants. Science Communication 40 (2), 228–245. doi: 10.1177/1075547018758076
-
Smallman, M. (2018). Citizen science and responsible research and innovation. In S. Hecker, M. Haklay, A. Bowser, Z. Makuch, J. Vogel & A. Bonn (Eds.), Citizen science: innovation in open science society and policy (pp. 241–253). doi: 10.14324/111.9781787352339
-
Stern, M. J. & Coleman, K. J. (2015). The multidimensionality of trust: applications in collaborative natural resource management. Society & Natural Resources 28 (2), 117–132. doi: 10.1080/08941920.2014.945062
-
Stocklmayer, S. M. & Bryant, C. (2012). Science and the public — what should people know? International Journal of Science Education, Part B 2 (1), 81–101. doi: 10.1080/09500693.2010.543186
-
Suldovsky, B. (2017). The information deficit model and climate change communication. In Oxford Research Encyclopedia of Climate Science . doi: 10.1093/acrefore/9780190228620.013.301
-
Turrini, T., Dörler, D., Richter, A., Heigl, F. & Bonn, A. (2018). The threefold potential of environmental citizen science — generating knowledge, creating learning opportunities and enabling civic participation. Biological Conservation 225 , 176–186. doi: 10.1016/j.biocon.2018.03.024
-
Vitone, T., Stofer, K., Sedonia Steininger, M., Hulcr, J., Dunn, R. & Lucky, A. (2016). School of Ants goes to college: integrating citizen science into the general education classroom increases engagement with science. JCOM 15 (01), A03. doi: 10.22323/2.15010203
-
Wintterlin, F., Hendriks, F., Mede, N. G., Bromme, R., Metag, J. & Schäfer, M. S. (2022). Predicting public trust in science: the role of basic orientations toward science, perceived trustworthiness of scientists, and experiences with science. Frontiers in Communication 6 , 822757. doi: 10.3389/fcomm.2021.822757
-
Wynne, B. (2006). Public engagement as a means of restoring public trust in science — hitting the notes, but missing the music? Community Genetics 9 (3), 211–220. doi: 10.1159/000092659
Authors
Baptiste Bedessem is a researcher in philosophy of science.
E-mail:
baptiste.bedessemp@gmail.com
.
Anne Dozières is a researcher in ecology, and manages the Vigie-Nature
program.
E-mail:
anne.dozieres@mnhn.fr
.
Anne-Caroline Prévot is a researcher in environmental psychology.
E-mail:
anne-caroline.prevot@mnhn.fr
.
Romain Julliard is a researcher in ecology.
E-mail:
romain.julliard@mnhn.fr
.
Endnotes
1 (1) “People trust scientists a lot more than they should”, (2) “People don’t realize just how flawed a lot of scientific research really is”, (3) “A lot of scientific theories are dead wrong”, (4) “Sometimes I think we put too much faith in science”, (5) “Our society places too much emphasis on science”, (6) “I am concerned by the amount of influence that scientists have in society”.